Hierarchical Attention and Knowledge Matching Networks With Information Enhancement for End-to-End Task-Oriented Dialog Systems.
IEEE ACCESS(2019)
摘要
Nowadays, most end-to-end task-oriented dialog systems are based on sequence-to-sequence (Seq2seq), which is an encoder-decoder framework. These systems sometimes produce grammatically correct, but logically incorrect responses. This phenomenon is usually due to information mismatching. To solve this problem, we introduce hierarchical attention and knowledge matching networks with information enhancement (HAMI) for task-oriented dialog systems. It contains a hierarchical attention dialog encoder (HADE) that models dialogs at the word and sentence level separately. HADE can focus on important words in a dialog history and generate context-aware representations. HAMI also contains a unique knowledge matching module to detect entities and interact with a knowledge base (KB). Then, dialog history and KB result representations serve as guidance for system response generation. Finally, HAMI's loss function is designed with an information regularization term to emphasize the importance of entities. The experimental results show that HAMI improves 9.8% in entity F1 compared with vanilla Seq2seq. HAMI also outperforms state-of-the-art models in both the entity F1 and dialog accuracy metrics.
更多查看译文
关键词
Dialog systems,end-to-end response generation,context modeling,hierarchical attention,knowledge base interaction,neural networks,information enhancement
AI 理解论文
溯源树
样例
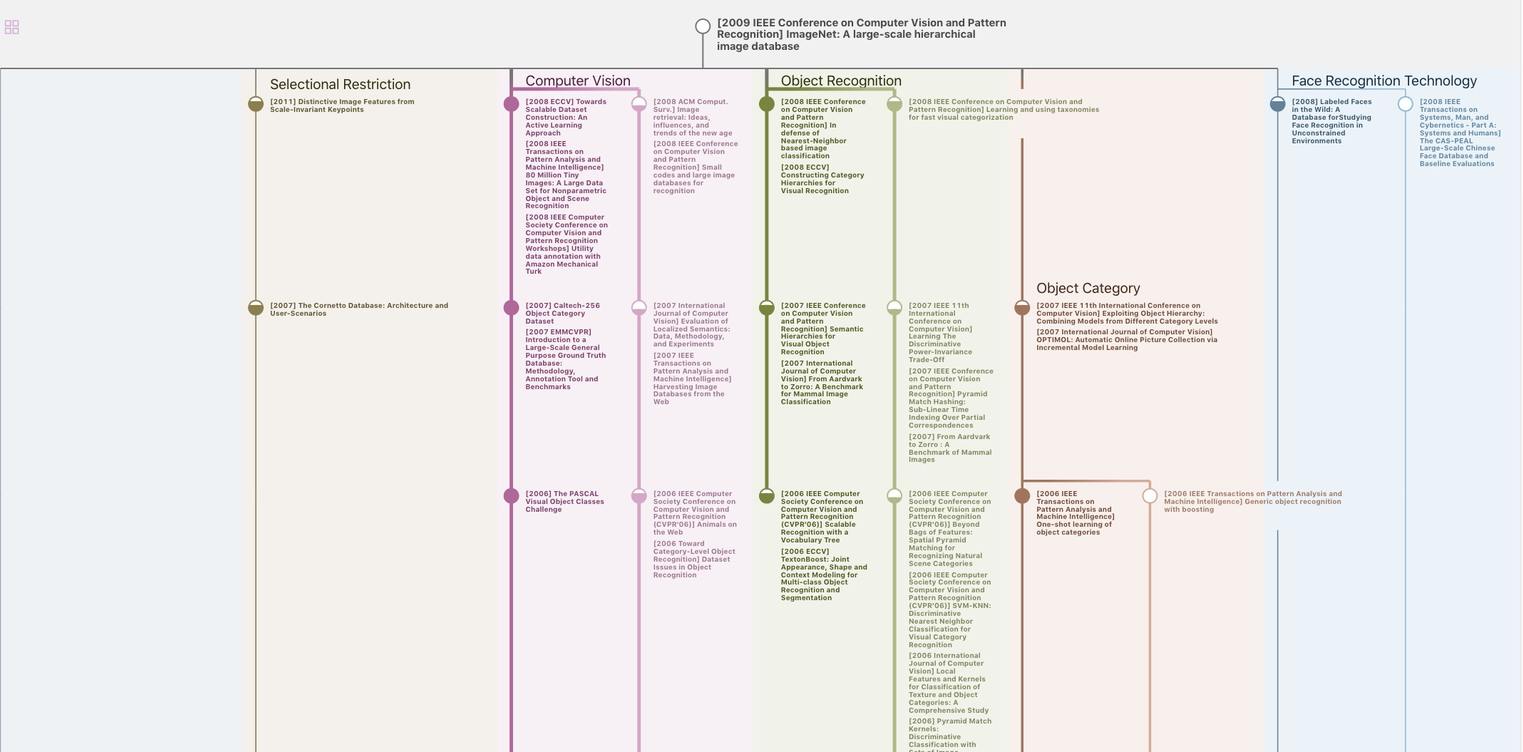
生成溯源树,研究论文发展脉络
Chat Paper
正在生成论文摘要