Facial Component-Landmark Detection with Weakly-Supervised LR-CNN
IEEE access(2019)
摘要
In this paper, we propose a weakly supervised landmark-region-based convolutional neural network (LR-CNN) framework to detect facial component and landmark simultaneously. Most of the existing course-to-fine facial detectors fail to detect landmark accurately without lots of fully labeled data, which are costly to obtain. We can handle the task with a small amount of finely labeled data. First, deep convolutional generative adversarial networks are utilized to generate training samples with weak labels, as data preparation. Then, through weakly supervised learning, our LR-CNN model can be trained effectively with a small amount of finely labeled data and a large amount of generated weakly labeled data. Notably, our approach can handle the situation when large occlusion areas occur, as we localize visible facial components before predicting corresponding landmarks. Detecting unblocked components first helps us to focus on the informative area, resulting in a better performance. Additionally, to improve the performance of the above tasks, we design two models as follows: 1) we add AnchorAlign in the region proposal networks to accurately localize components and 2) we propose a two-branch model consisting classification branch and regression branch to detect landmark. Extensive evaluations on benchmark datasets indicate that our proposed approach is able to complete the multi-task facial detection and outperforms the state-of-the-art facial component and landmark detection algorithms.
更多查看译文
关键词
Weakly-supervised,facial landmark,generative adversarial network,region-based convolutional neural network
AI 理解论文
溯源树
样例
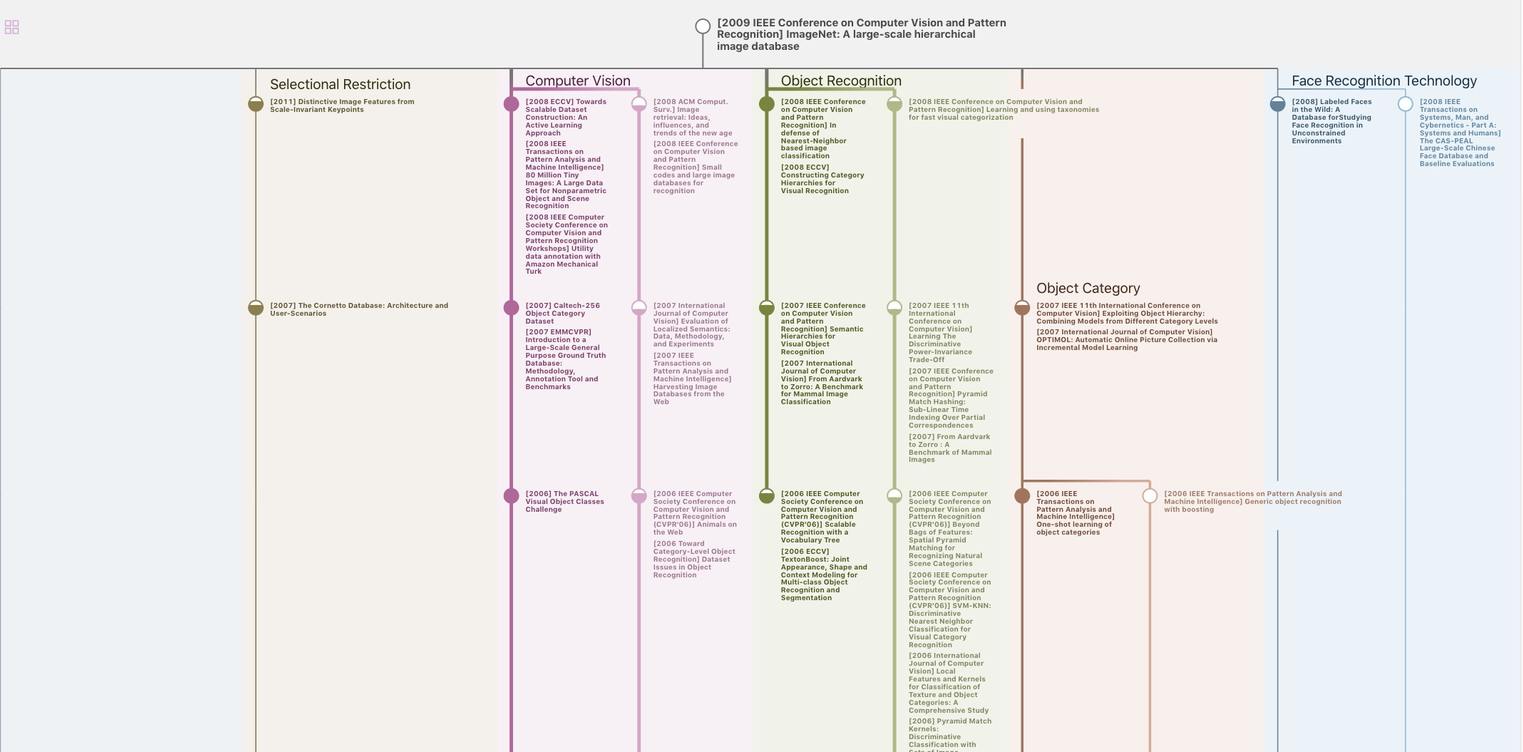
生成溯源树,研究论文发展脉络
Chat Paper
正在生成论文摘要