A Heuristic Transformation in Discriminative Dictionary Learning for Person Re-Identification.
IEEE ACCESS(2019)
摘要
Person re-identification (ReID) is an important technology for target association in surveillance applications. Recently, sparse representation-based classification has been applied to person ReID with the advantage of discriminative feature extraction and has produced excellent results. The dictionary learning (DL) method is vital to the sparse representation, and the discriminative power of the learned dictionary determines the performance of ReID. Unlike previous approaches that only added constraints in DL, we propose a discriminative dictionary learning model (DDLM) that learns the discriminative dictionary by transforming the dictionary representation space in the training process. We determine the statistical distribution from the training data and divide the data into two categories according to the contribution for sparse representation: the high-contribution data and low-contribution data. Then, we extend the information space that contains the most high-contribution data and shrink the remaining parts. As the representation space of the dictionary is transformed, the solving process is modified accordingly. The experiments on the benchmark datasets (CAVIAR4REID, ETHZ, and i-LIDS) demonstrate that the proposed model outperforms the state-of-the-art approaches.
更多查看译文
关键词
Piecewise linear transformation,discriminative dictionary learning,sparse representation,person re-identification
AI 理解论文
溯源树
样例
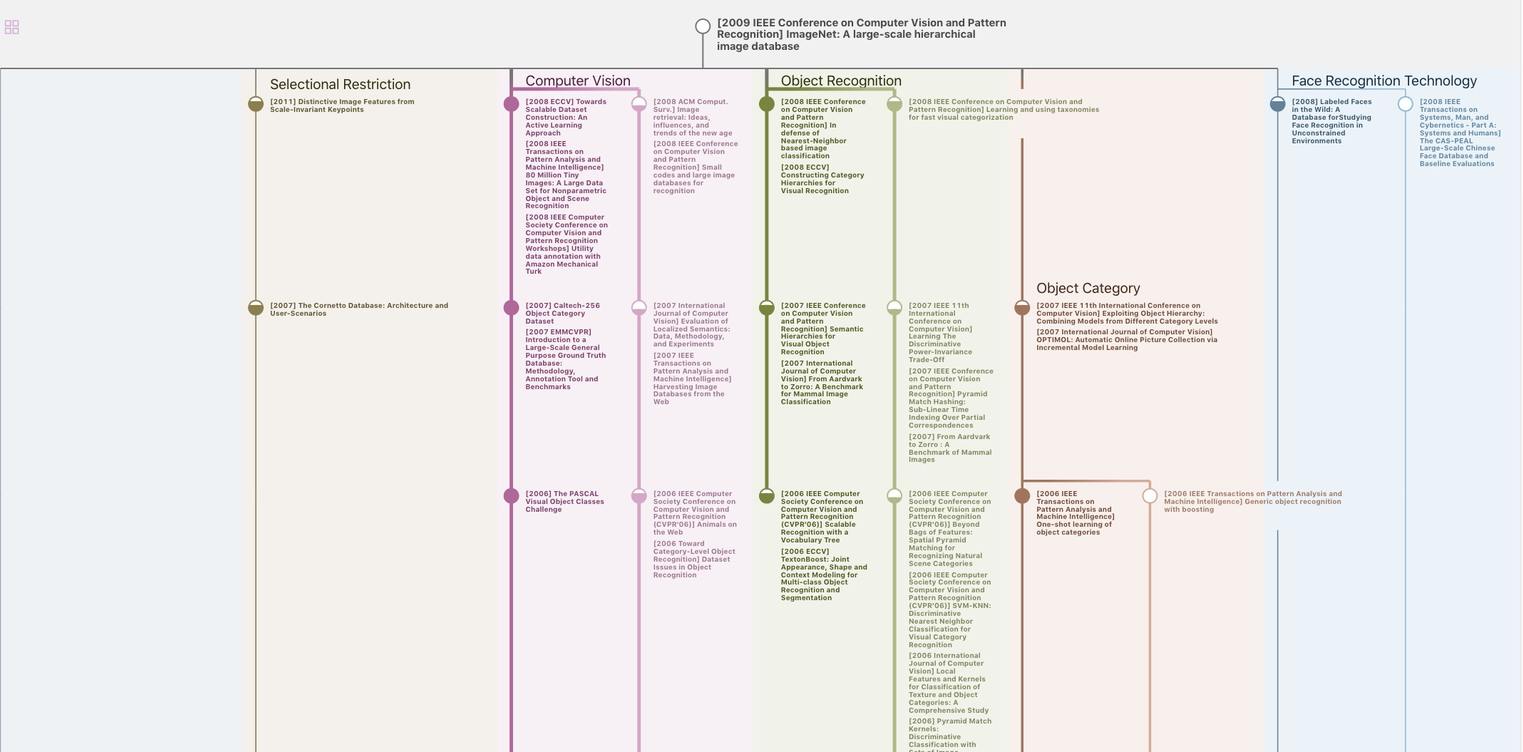
生成溯源树,研究论文发展脉络
Chat Paper
正在生成论文摘要