Deep Reinforcement Learning for Router Selection in Network With Heavy Traffic.
IEEE ACCESS(2019)
摘要
The rapid development of wireless communications brings a tremendous increase in the amount number of data streams and poses significant challenges to the traditional routing protocols. In this paper, we leverage deep reinforcement learning (DRL) for router selection in the network with heavy traffic, aiming at reducing the network congestion and the length of the data transmission path. We first illustrate the challenges of the existing routing protocols when the amount of the data explodes. We then utilize the Markov decision process (RSMDP) to formulate the routing problem. Two novel deep Q network (DQN)-based algorithms are designed to reduce the network congestion probability with a short transmission path: one focusing on reducing the congestion probability; while the other focuses on shortening the transmission path. The simulation results demonstrate that the proposed algorithms can achieve higher network throughput comparing to existing routing algorithms in heavy network traffic scenarios.
更多查看译文
关键词
Deep reinforcement learning,routing,network congestion,network throughput,deep Q network
AI 理解论文
溯源树
样例
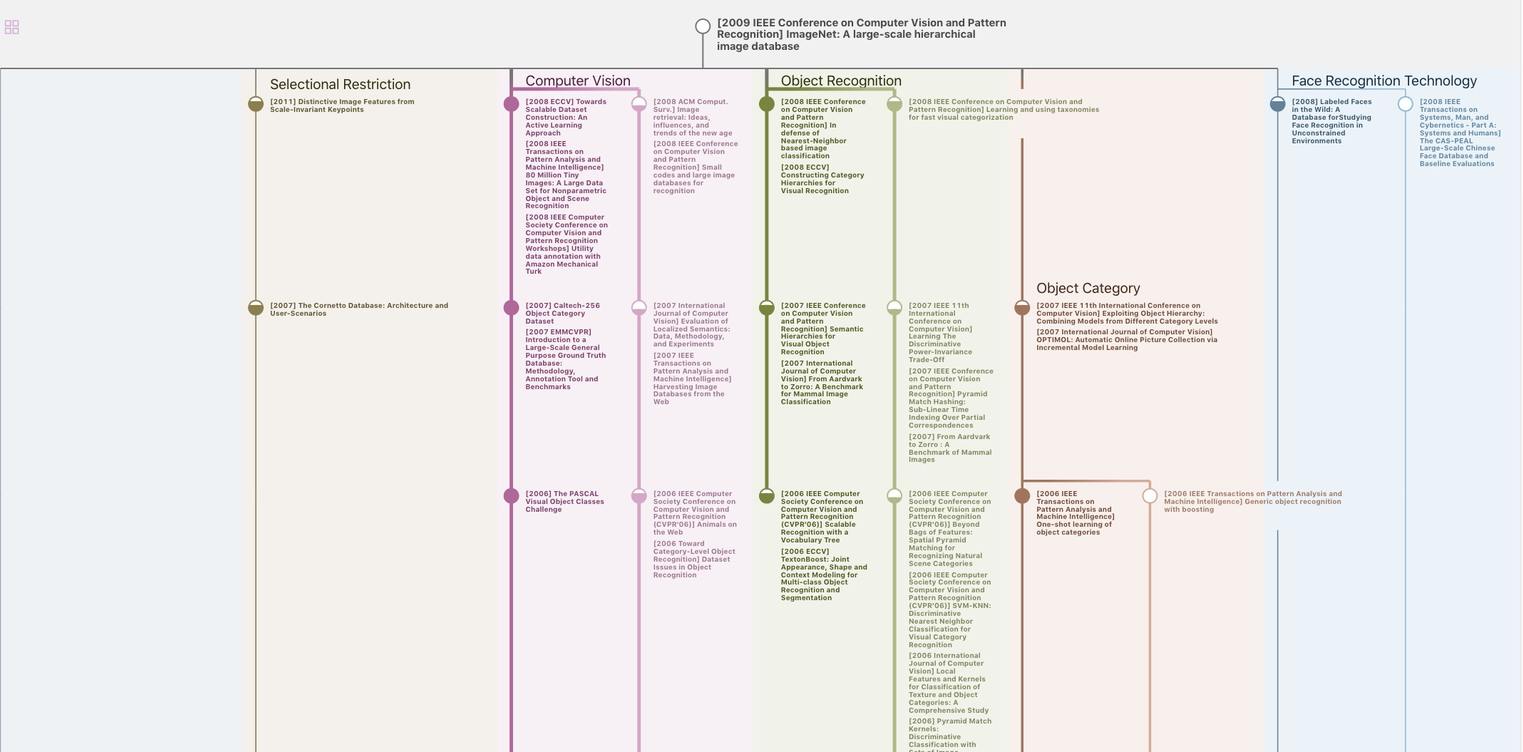
生成溯源树,研究论文发展脉络
Chat Paper
正在生成论文摘要