Consistent Rank Logits for Ordinal Regression with Convolutional Neural Networks.
arXiv: Learning(2019)
摘要
While extraordinary progress has been made towards developing neural network architectures for classification tasks, commonly used loss functions such as the multi-category cross entropy loss are inadequate for ranking and ordinal regression problems. To address this issue, approaches have been developed that transform ordinal target variables series of binary classification tasks, resulting in robust ranking algorithms with good generalization performance. However, to model ordinal information appropriately, ideally, a rank-monotonic prediction function is required such that confidence scores are ordered and consistent. We propose a new framework (Consistent Rank Logits, CORAL) with theoretical guarantees for rank-monotonicity and consistent confidence scores. Through parameter sharing, our framework benefits from low training complexity and can easily be implemented to extend common convolutional neural network classifiers for ordinal regression tasks. Furthermore, our empirical results support the proposed theory and show a substantial improvement compared to the current state-of-the-art ordinal regression method for age prediction from face images.
更多查看译文
AI 理解论文
溯源树
样例
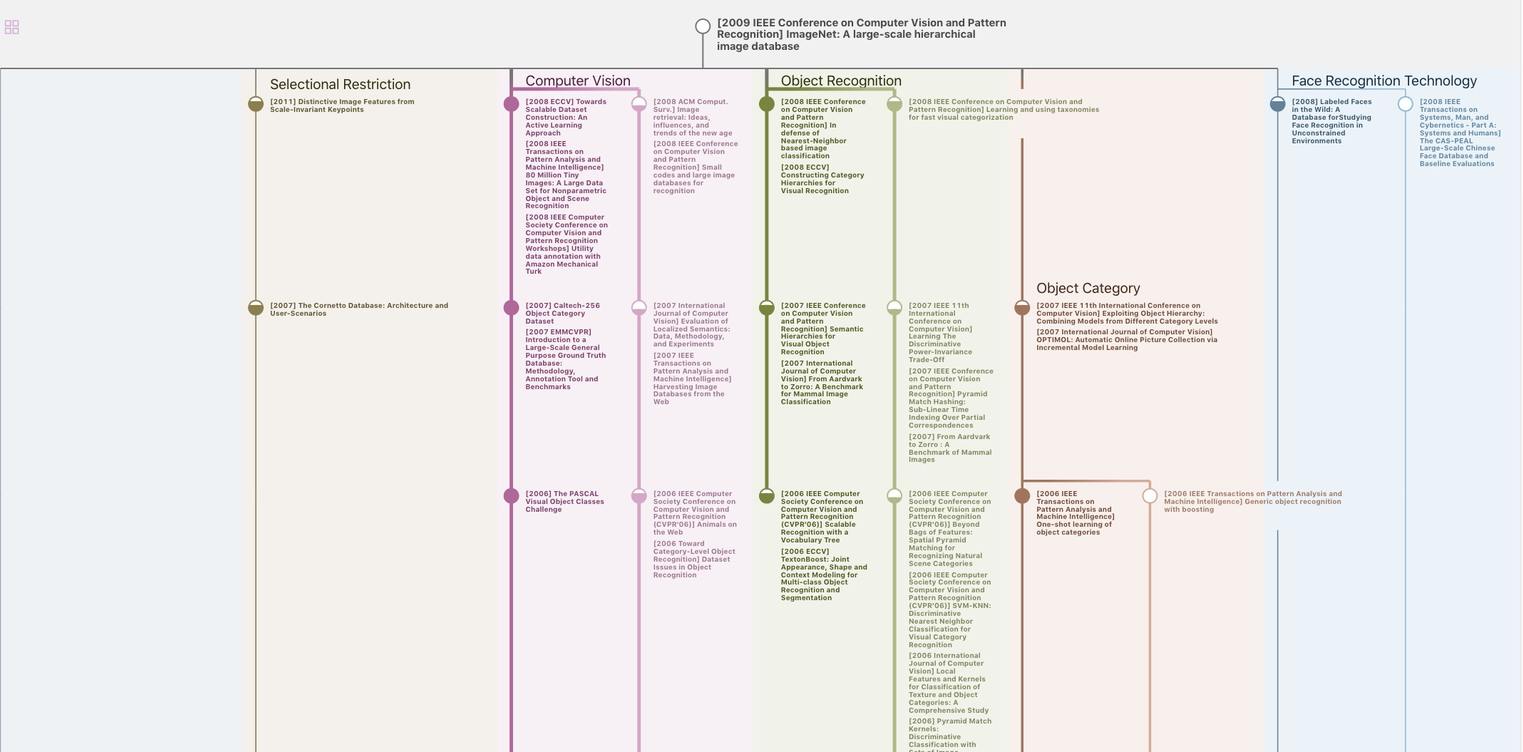
生成溯源树,研究论文发展脉络
Chat Paper
正在生成论文摘要