On the Consistency of Top-k Surrogate Losses.
ICML 2020(2020)
摘要
The top-$k$ error is often employed to evaluate performance for challenging classification tasks in computer vision as it is designed to compensate for ambiguity in ground truth labels. This practical success motivates our theoretical analysis of consistent top-$k$ classification. To this end, we define top-$k$ calibration as a necessary and sufficient condition for consistency, for bounded below loss functions. Unlike prior work, our analysis of top-$k$ calibration handles non-uniqueness of the predictor scores, and extends calibration to consistency -- providing a theoretically sound basis for analysis of this topic. Based on the top-$k$ calibration analysis, we propose a rich class of top-$k$ calibrated Bregman divergence surrogates. Our analysis continues by showing previously proposed hinge-like top-$k$ surrogate losses are not top-$k$ calibrated and thus inconsistent. On the other hand, we propose two new hinge-like losses, one which is similarly inconsistent, and one which is consistent. Our empirical results highlight theoretical claims, confirming our analysis of the consistency of these losses.
更多查看译文
AI 理解论文
溯源树
样例
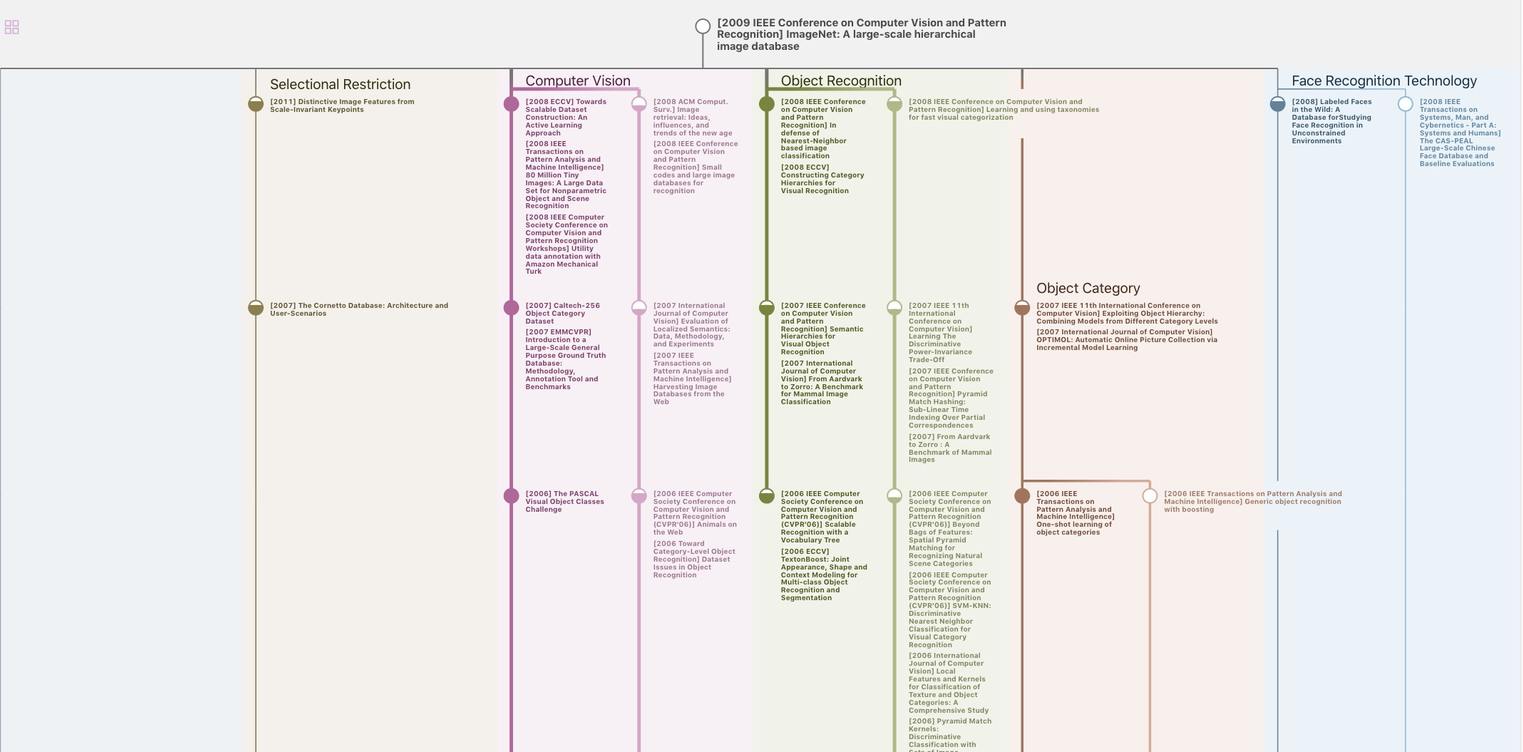
生成溯源树,研究论文发展脉络
Chat Paper
正在生成论文摘要