Contextual Bandits with Continuous Actions: Smoothing, Zooming, and Adapting
JOURNAL OF MACHINE LEARNING RESEARCH(2019)
摘要
We study contextual bandit learning with an abstract policy class and continuous action space. We obtain two qualitatively different regret bounds: one competes with a smoothed version of the policy class under no continuity assumptions, while the other requires standard Lipschitz assumptions. Both bounds exhibit data-dependent "zooming" behavior and, with no tuning, yield improved guarantees for benign problems. We also study adapting to unknown smoothness parameters, establishing a price-of-adaptivity and deriving optimal adaptive algorithms that require no additional information.
更多查看译文
关键词
Contextual bandits,nonparametric learning
AI 理解论文
溯源树
样例
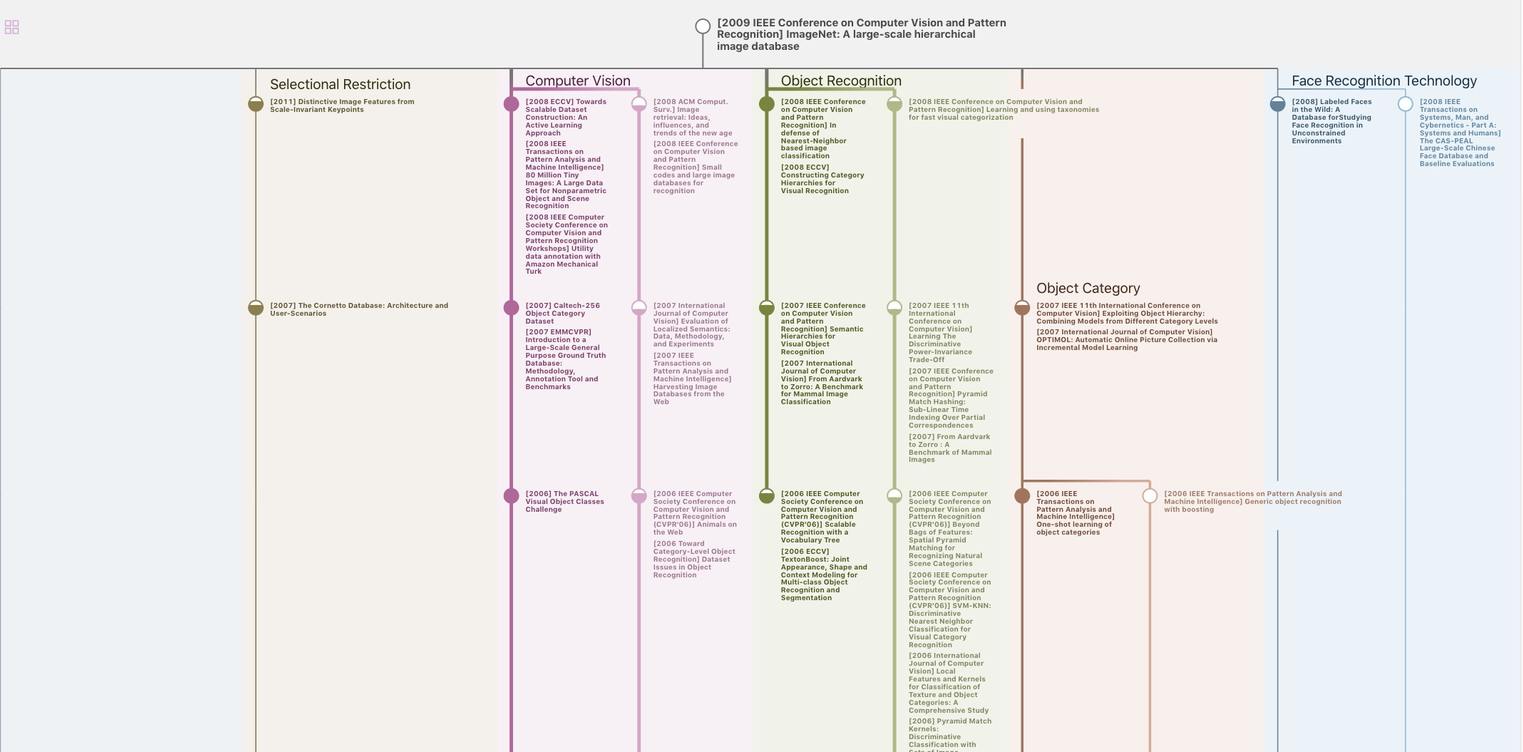
生成溯源树,研究论文发展脉络
Chat Paper
正在生成论文摘要