Improving Transparency of Deep Neural Inference Process
Progress in artificial intelligence(2019)
摘要
Deep learning techniques are rapidly advanced recently and becoming a necessity component for widespread systems. However, the inference process of deep learning is black box and is not very suitable to safety-critical systems which must exhibit high transparency. In this paper, to address this black-box limitation, we develop a simple analysis method which consists of (1) structural feature analysis: lists of the features contributing to inference process, (2) linguistic feature analysis: lists of the natural language labels describing the visual attributes for each feature contributing to inference process, and (3) consistency analysis: measuring consistency among input data, inference (label), and the result of our structural and linguistic feature analysis. Our analysis is simplified to reflect the actual inference process for high transparency, whereas it does not include any additional black-box mechanisms such as LSTM for highly human readable results. We conduct experiments and discuss the results of our analysis qualitatively and quantitatively and come to believe that our work improves the transparency of neural networks. Evaluated through 12,800 human tasks, 75% workers answer that input data and result of our feature analysis are consistent, and 70% workers answer that inference (label) and result of our feature analysis are consistent. In addition to the evaluation of the proposed analysis, we find that our analysis also provides suggestions, or possible next actions such as expanding neural network complexity or collecting training data to improve a neural network.
更多查看译文
关键词
Transparency,Deep neural network,Black box,Explainable AI,Visualization,Visual attribute
AI 理解论文
溯源树
样例
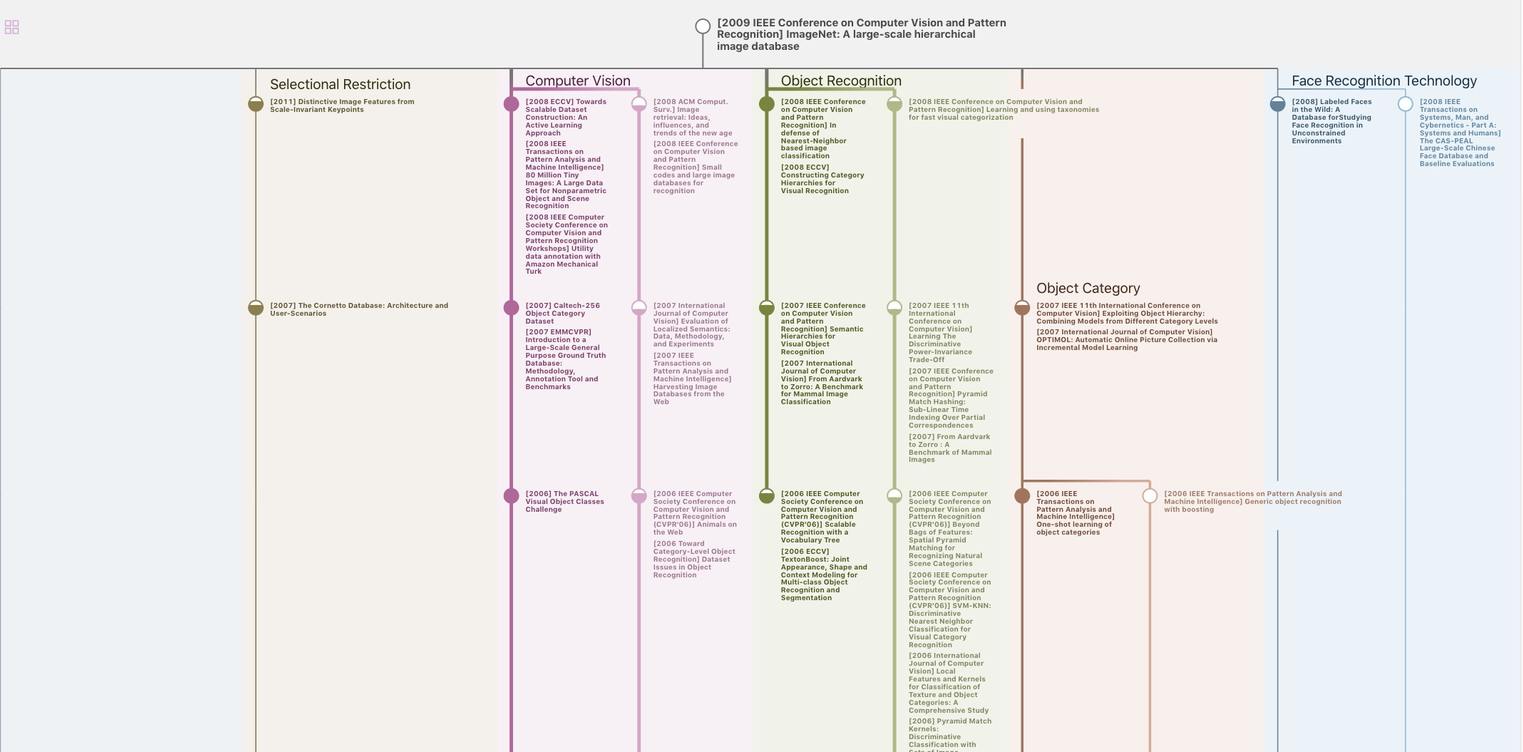
生成溯源树,研究论文发展脉络
Chat Paper
正在生成论文摘要