Self-Attention Networks For Connectionist Temporal Classification In Speech Recognition
2019 IEEE INTERNATIONAL CONFERENCE ON ACOUSTICS, SPEECH AND SIGNAL PROCESSING (ICASSP)(2019)
摘要
The success of self-attention in NLP has led to recent applications in end-to-end encoder-decoder architectures for speech recognition. Separately, connectionist temporal classification (CTC) has matured as an alignment-free, non-autoregressive approach to sequence transduction, either by itself or in various multitask and decoding frameworks. We propose SAN-CTC, a deep, fully self-attentional network for CTC, and show it is tractable and competitive for end-to-end speech recognition. SAN-CTC trains quickly and outperforms existing CTC models and most encoder-decoder models, with character error rates (CERs) of 4.7% in 1 day on WSJ eval92 and 2.8% in 1 week on LibriSpeech test-clean, with a fixed architecture and one GPU. Similar improvements hold for WERs after LM decoding. We motivate the architecture for speech, evaluate position and down-sampling approaches, and explore how label alphabets (character, phoneme, subword) affect attention heads and performance.
更多查看译文
关键词
speech recognition, connectionist temporal classification, self-attention, multi-head attention, end-to-end
AI 理解论文
溯源树
样例
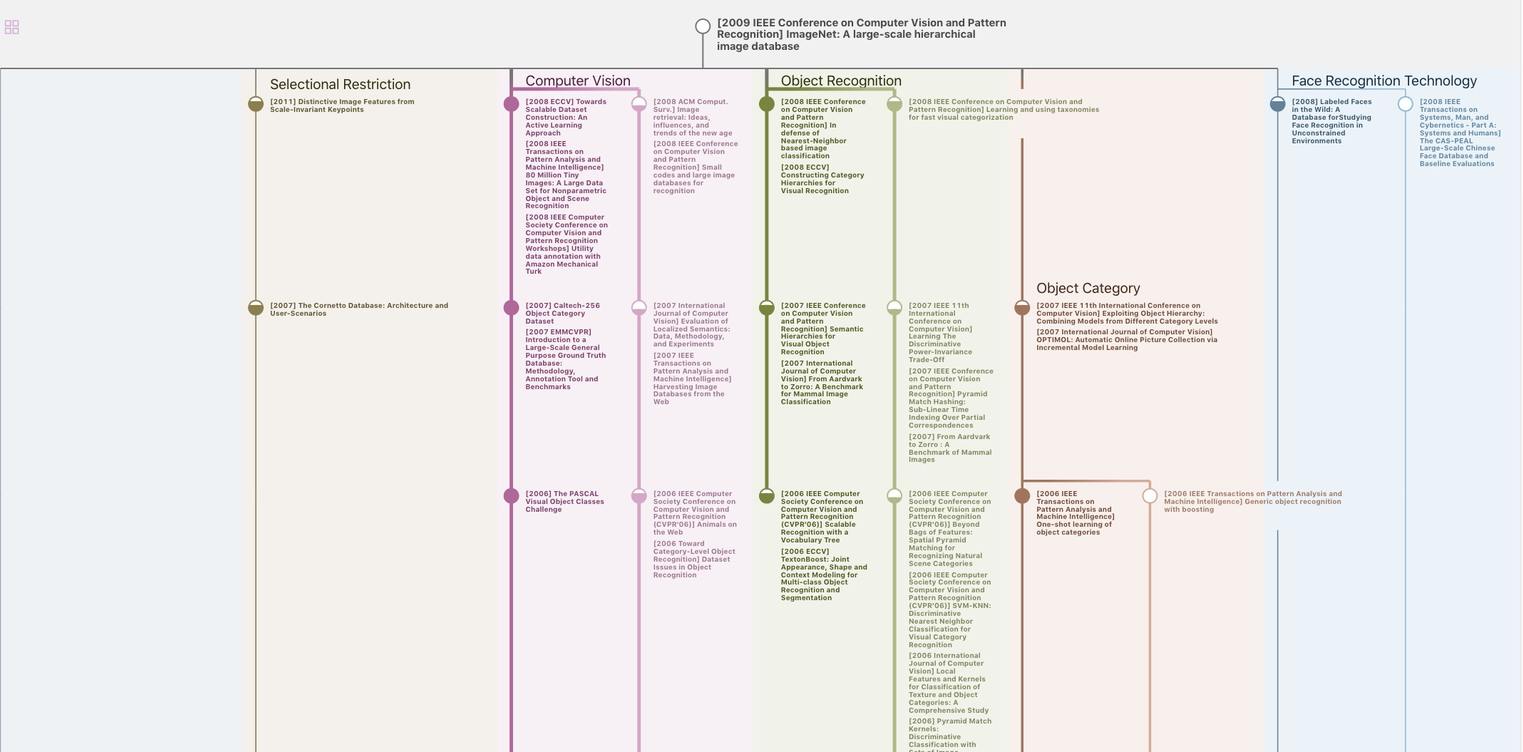
生成溯源树,研究论文发展脉络
Chat Paper
正在生成论文摘要