Multi-fidelity Bayesian Optimization with Max-value Entropy Search.
arXiv: Machine Learning(2019)
摘要
Bayesian optimization (BO) is an effective tool for black-box optimization in which objective function evaluation is usually quite expensive. In practice, lower fidelity approximations of the objective function are often available. Recently, multi-fidelity Bayesian optimization (MFBO) has attracted considerable attention because it can dramatically accelerate the optimization process by using those cheaper observations. We propose a novel information theoretic approach to MFBO. Information-based approaches are popular and empirically successful in BO, but existing studies for information-based MFBO are plagued by difficulty for accurately estimating the information gain. Our approach is based on a variant of information-based BO called max-value entropy search (MES), which greatly facilitates evaluation of the information gain in MFBO. In fact, computations of our acquisition function is written analytically except for one dimensional integral and sampling, which can be calculated efficiently and accurately. We demonstrate effectiveness of our approach by using synthetic and benchmark datasets, and further we show a real-world application to materials science data.
更多查看译文
AI 理解论文
溯源树
样例
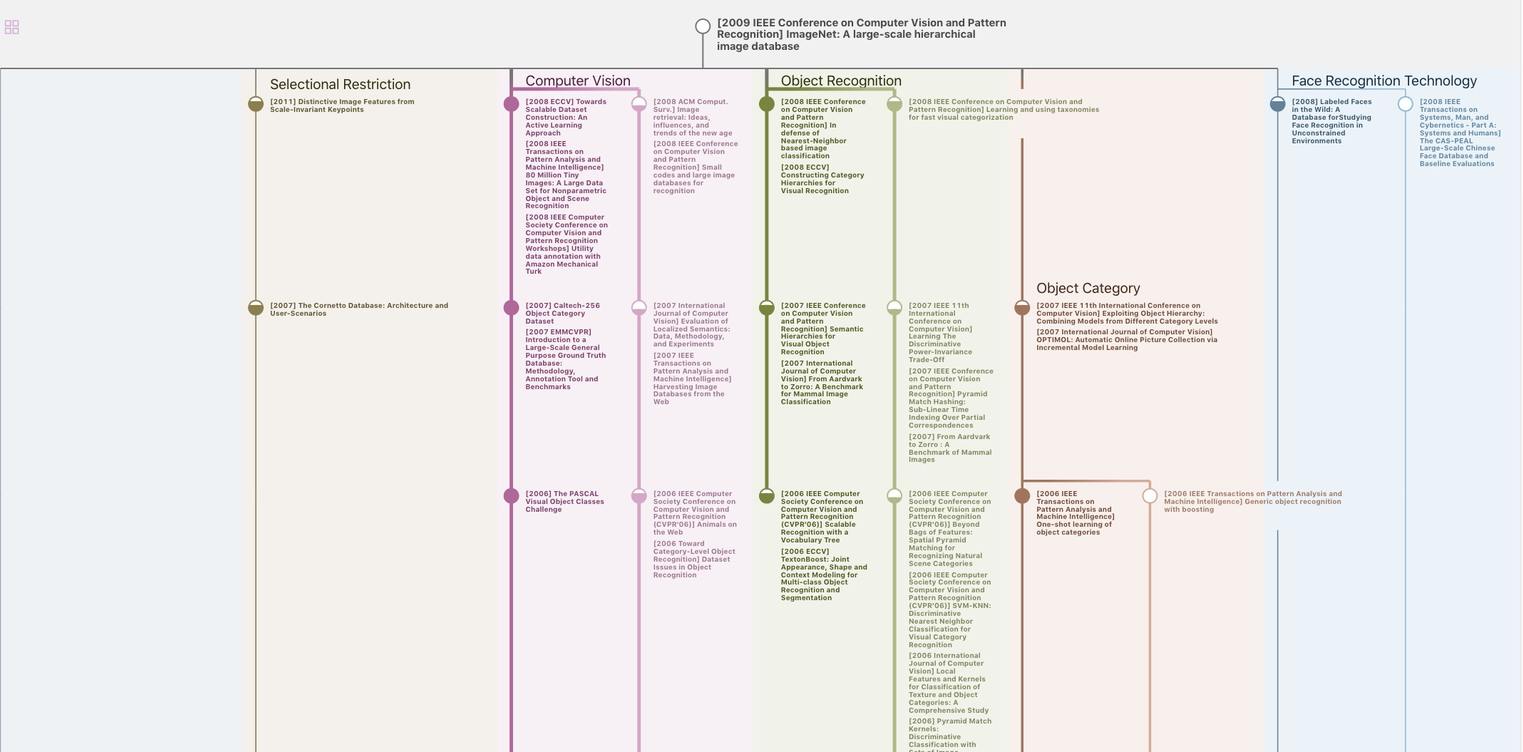
生成溯源树,研究论文发展脉络
Chat Paper
正在生成论文摘要