Gaussian Mean Field Regularizes by Limiting Learned Information.
ENTROPY(2019)
摘要
Variational inference with a factorized Gaussian posterior estimate is a widely-used approach for learning parameters and hidden variables. Empirically, a regularizing effect can be observed that is poorly understood. In this work, we show how mean field inference improves generalization by limiting mutual information between learned parameters and the data through noise. We quantify a maximum capacity when the posterior variance is either fixed or learned and connect it to generalization error, even when the KL-divergence in the objective is scaled by a constant. Our experiments suggest that bounding information between parameters and data effectively regularizes neural networks on both supervised and unsupervised tasks.
更多查看译文
关键词
information theory,variational inference,machine learning
AI 理解论文
溯源树
样例
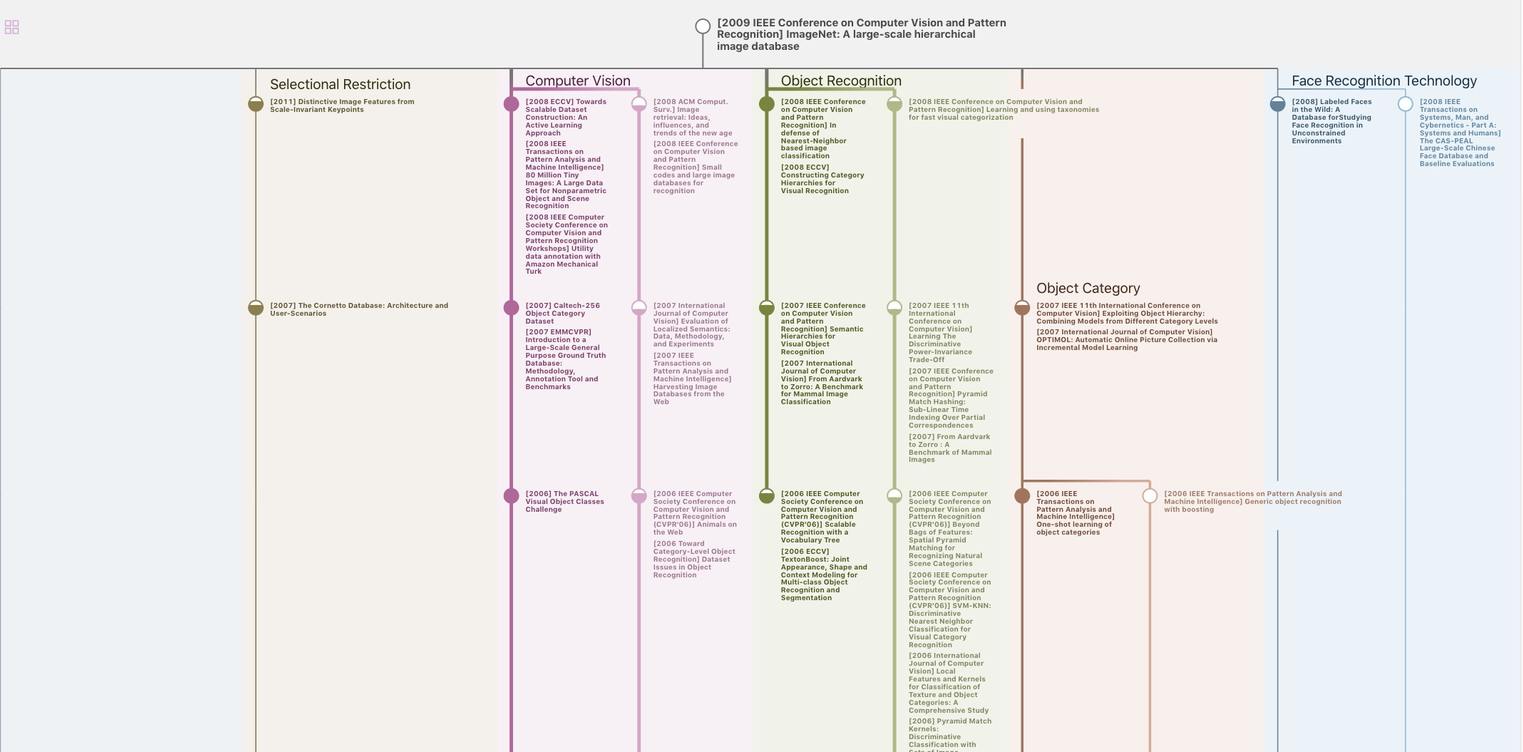
生成溯源树,研究论文发展脉络
Chat Paper
正在生成论文摘要