A Bayesian Deep Learning Framework for End-To-End Prediction of Emotion From Heartbeat
IEEE Transactions on Affective Computing(2022)
摘要
Automatic prediction of emotion promises to revolutionise human-computer interaction. Recent trends involve fusion of multiple data modalities
$-$
audio, visual, and physiological
$-$
to classify emotional state. However, in practice, collection of physiological data ‘in the wild’ is currently limited to heartbeat time series of the kind generated by affordable wearable heart monitors. Furthermore, real-world applications of emotion prediction often require some measure of uncertainty over model output, in order to inform downstream decision-making. We present here an end-to-end deep learning model for classifying emotional valence from unimodal heartbeat time series. We further propose a Bayesian framework for modelling uncertainty over these valence predictions, and describe a probabilistic procedure for choosing to accept or reject model output according to the intended application. We benchmarked our framework against two established datasets and achieved peak classification accuracy of 90 percent. These results lay the foundation for applications of affective computing in real-world domains such as healthcare, where a high premium is placed on non-invasive collection of data, and predictive certainty.
更多查看译文
关键词
Bayesian neural networks,electrocardiography,emotion recognition,end-to-end learning
AI 理解论文
溯源树
样例
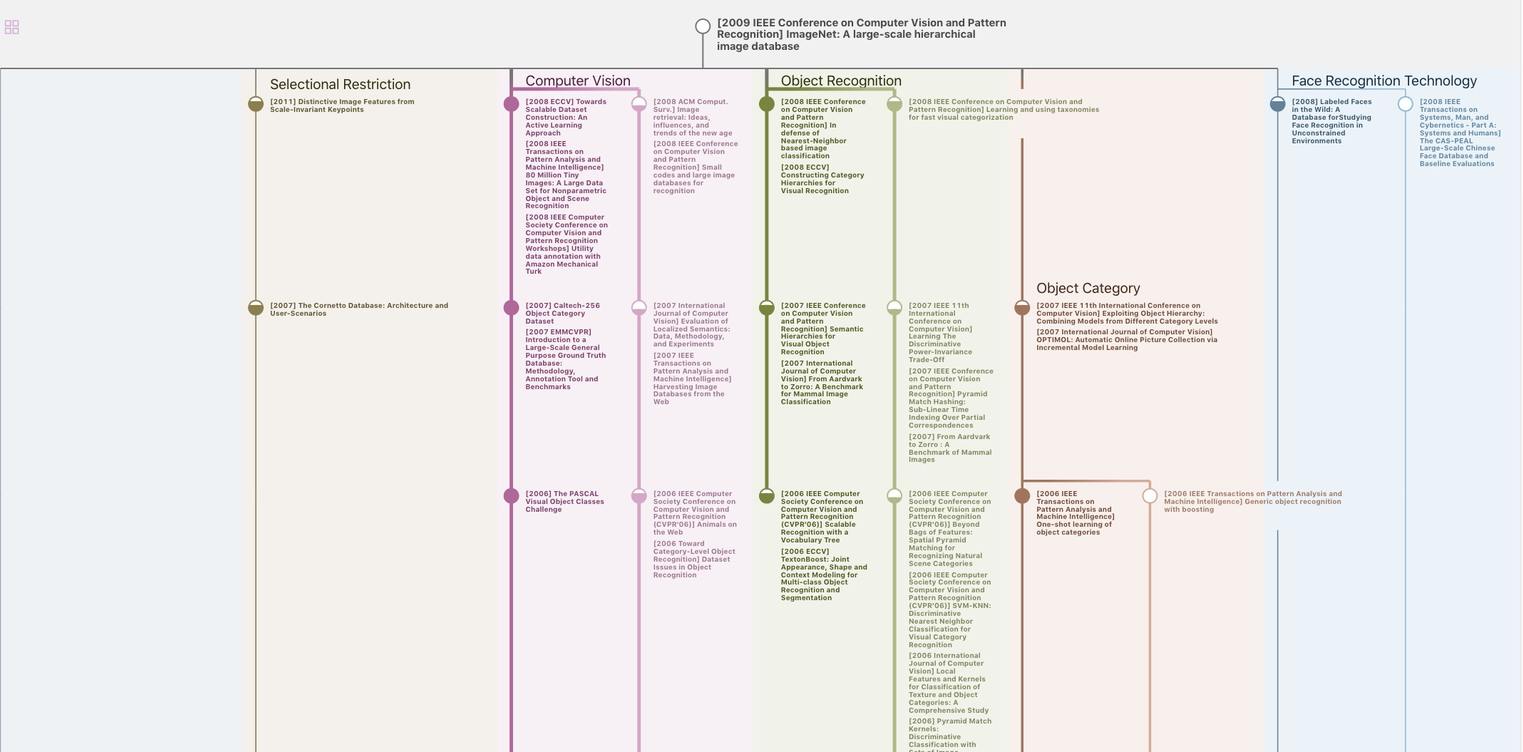
生成溯源树,研究论文发展脉络
Chat Paper
正在生成论文摘要