The Simpler the Better: Constant Velocity for Pedestrian Motion Prediction.
arXiv: Computer Vision and Pattern Recognition(2019)
摘要
Pedestrian motion prediction is a fundamental task for autonomous robots and vehicles to operate safely. In recent years many complex models have been proposed to address this problem. While complex models can be justified, simple models should be preferred given the same or better performance. In this work we show that a simple Constant Velocity Model can achieve competitive performance on this task. We evaluate the Constant Velocity Model using two popular benchmark datasets for pedestrian motion prediction and show that it outperforms state-of-the-art models and several common baselines. The success of this model indicates that either neural networks are not able to make use of the additional information they are provided with, or it is not as relevant as commonly believed. Therefore, we analyze how neural networks process this information and how it impacts their predictions. Our analysis shows that neural networks implicitly learn environmental priors that have a negative impact on their generalization capability, most of the pedestrianu0027s motion history is ignored and interactions - while happening - are too complex to predict. These findings explain the success of the Constant Velocity Model and lead to a better understanding of the problem at hand.
更多查看译文
AI 理解论文
溯源树
样例
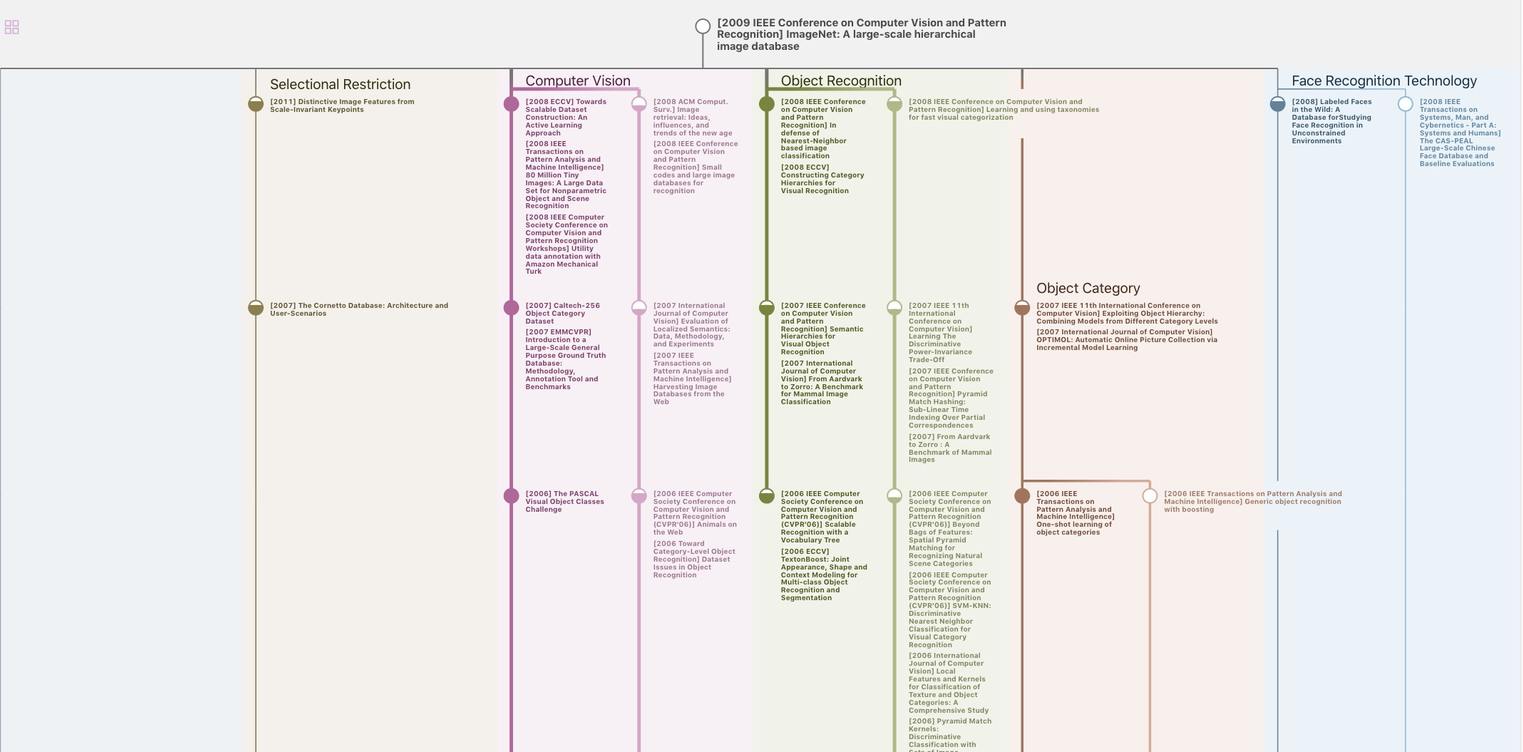
生成溯源树,研究论文发展脉络
Chat Paper
正在生成论文摘要