SNN under Attack: are Spiking Deep Belief Networks vulnerable to Adversarial Examples?
arXiv: Learning(2019)
摘要
Recently, many adversarial examples have emerged for Deep Neural Networks (DNNs) causing misclassifications. However, in-depth work still needs to be performed to demonstrate such attacks and security vulnerabilities for spiking neural networks (SNNs), i.e. the 3rd generation NNs. This paper aims at addressing the fundamental questions:"Are SNNs vulnerable to the adversarial attacks as well?" and "if yes, to what extent?" Using a Spiking Deep Belief Network (SDBN) for the MNIST database classification, we show that the SNN accuracy decreases accordingly to the noise magnitude in data poisoning random attacks applied to the test images. Moreover, SDBNs generalization capabilities increase by applying noise to the training images. We develop a novel black box attack methodology to automatically generate imperceptible and robust adversarial examples through a greedy algorithm, which is first of its kind for SNNs.
更多查看译文
AI 理解论文
溯源树
样例
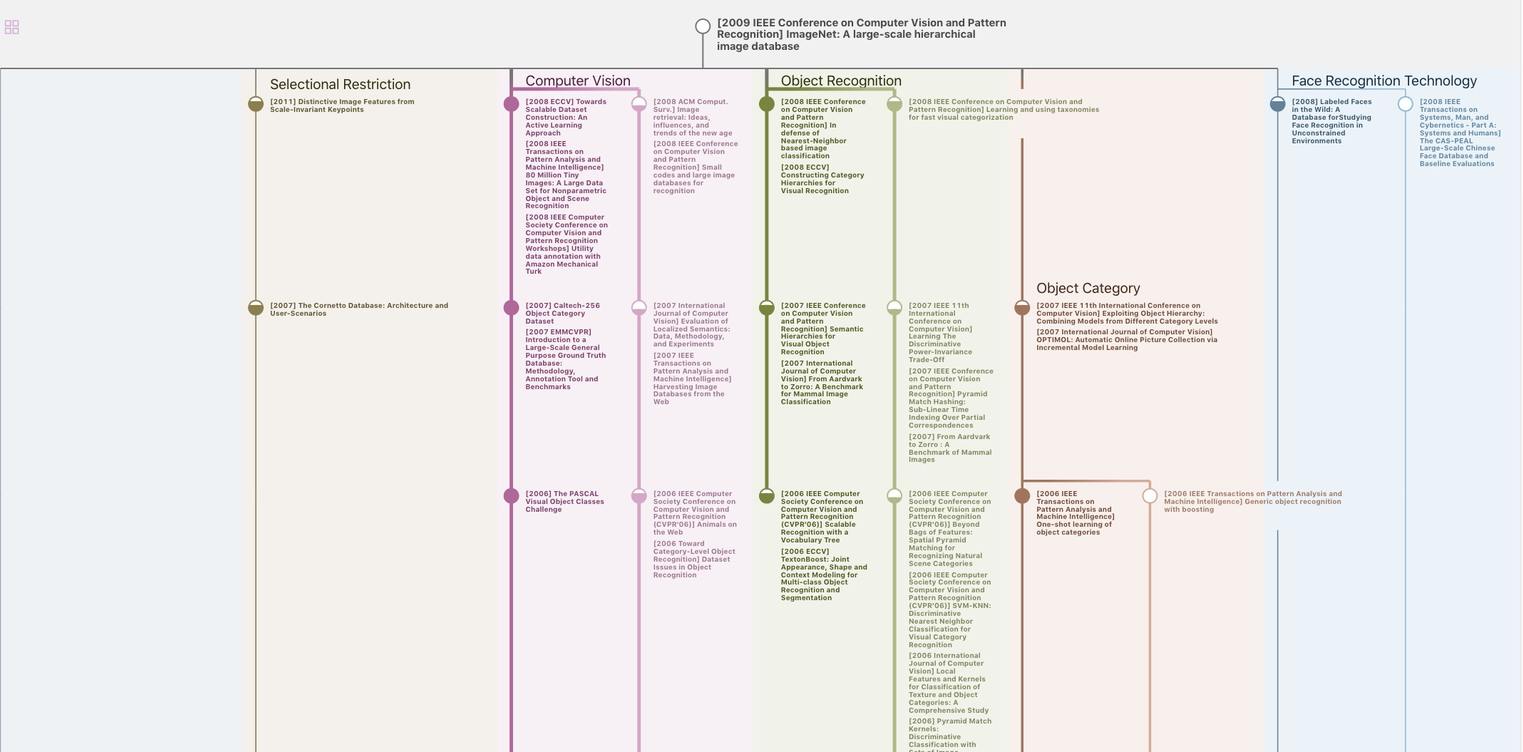
生成溯源树,研究论文发展脉络
Chat Paper
正在生成论文摘要