Anytime Online-to-Batch, Optimism and Acceleration
arXiv: Machine Learning(2019)
摘要
A standard way to obtain convergence guarantees in stochastic convex optimization is to run an online learning algorithm and then output the average of its iterates: the actual iterates of the online learning algorithm do not come with individual guarantees. We close this gap by introducing a black-box modification to any online learning algorithm whose iterates converge to the optimum in stochastic scenarios. We then consider the case of smooth losses, and show that combining our approach with optimistic online learning algorithms immediately yields a fast convergence rate of $O(L/T^{3/2}+\sigma/\sqrt{T})$ on $L$-smooth problems with $\sigma^2$ variance in the gradients. Finally, we provide a reduction that converts any adaptive online algorithm into one that obtains the optimal accelerated rate of $\tilde O(L/T^2 + \sigma/\sqrt{T})$, while still maintaining $\tilde O(1/\sqrt{T})$ convergence in the non-smooth setting. Importantly, our algorithms adapt to $L$ and $\sigma$ automatically: they do not need to know either to obtain these rates.
更多查看译文
关键词
optimism,online-to-batch
AI 理解论文
溯源树
样例
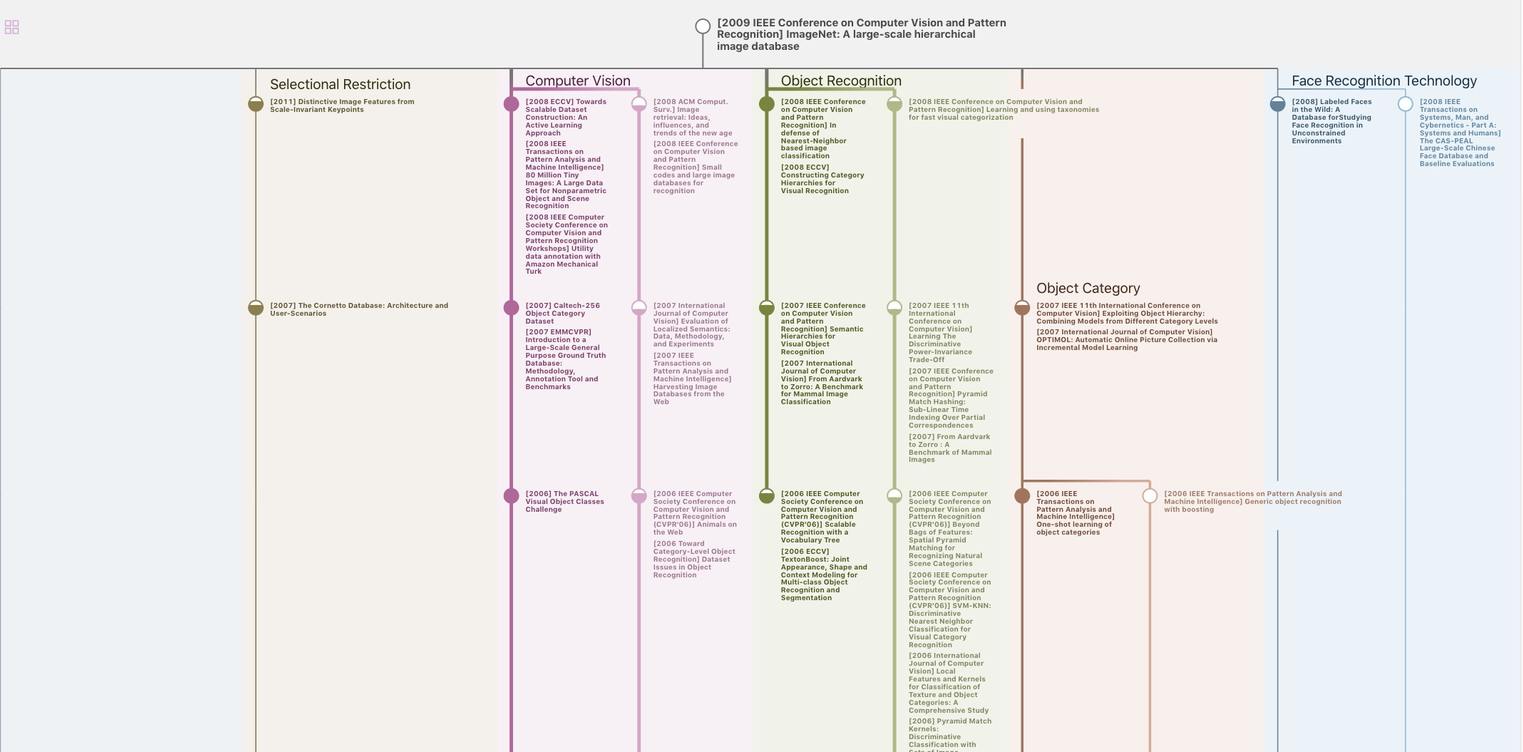
生成溯源树,研究论文发展脉络
Chat Paper
正在生成论文摘要