Generalized Intersection Over Union: A Metric and a Loss for Bounding Box Regression
2019 IEEE/CVF Conference on Computer Vision and Pattern Recognition (CVPR)(2019)
摘要
Intersection over Union (IoU) is the most popular evaluation metric used in the object detection benchmarks. However, there is a gap between optimizing the commonly used distance losses for regressing the parameters of a bounding box and maximizing this metric value. The optimal objective for a metric is the metric itself. In the case of axis-aligned 2D bounding boxes, it can be shown that IoU can be directly used as a regression loss. However, IoU has a plateau making it infeasible to optimize in the case of non-overlapping bounding boxes. In this paper, we address the this weakness by introducing a generalized version of IoU as both a new loss and a new metric. By incorporating this generalized IoU (GIoU) as a loss into the state-of-the art object detection frameworks, we show a consistent improvement on their performance using both the standard, IoU based, and new, GIoU based, performance measures on popular object detection benchmarks such as PASCAL VOC and MS COCO.
更多查看译文
关键词
Recognition: Detection,Categorization,Retrieval,Deep Learning
AI 理解论文
溯源树
样例
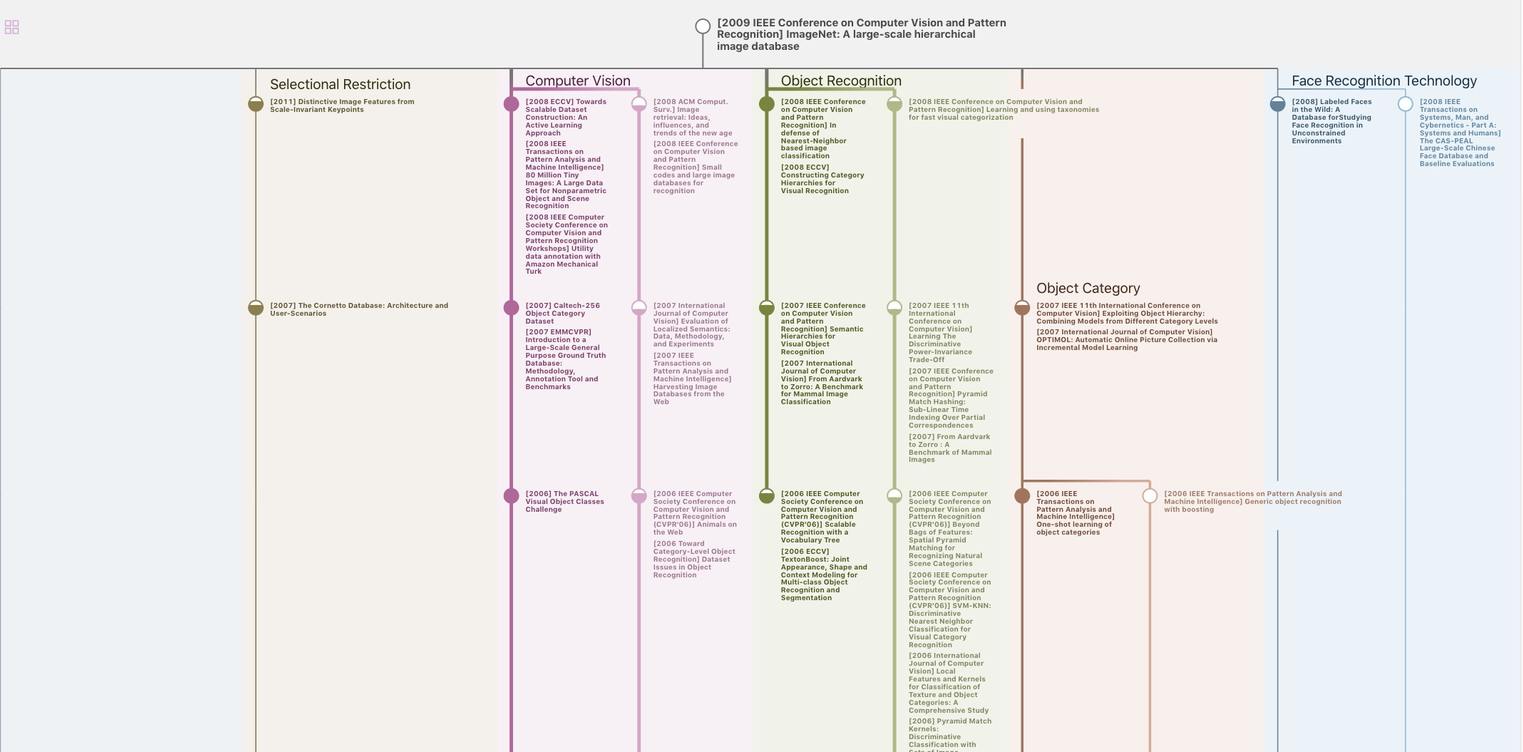
生成溯源树,研究论文发展脉络
Chat Paper
正在生成论文摘要