Saec: Similarity-Aware Embedding Compression in Recommendation Systems.
APSys '20: Proceedings of the 11th ACM SIGOPS Asia-Pacific Workshop on Systems(2019)
摘要
Embedding methods are commonly used in recommender systems to represent features about user and item. An impeding practical challenge is that the large number of embedding vectors incurs substantial memory footprint for serving queries, especially as the number of features continues to grow. We propose an embedding compression system called Saec to address this challenge. Saec exploits the similarity among features within a field as they represent the same attribute of user or item, and uses clustering to compress the embeddings. We propose a new fast clustering method that relies on the empirical heavy-tailed nature of features to drastically reduce the clustering overhead. We implement a prototype of Saec on a production system and evaluate it with private feature datasets from a large Internet company. Testbed experiments show that Saec consistently reduces the memory footprint of embedding vectors from 4.46 GB to 161 MB. The fast clustering method we design achieves 32x speedup compared to the baseline method.
更多查看译文
AI 理解论文
溯源树
样例
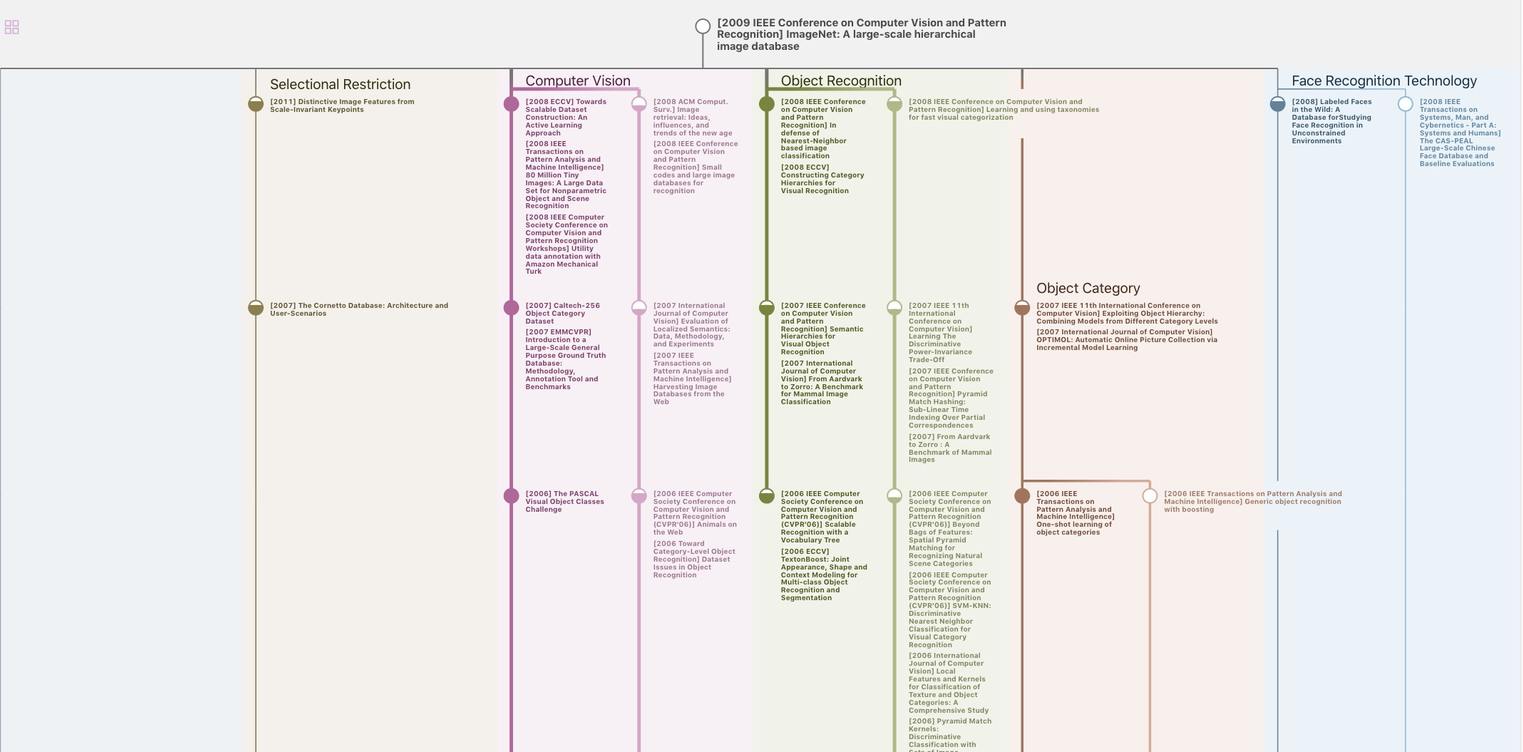
生成溯源树,研究论文发展脉络
Chat Paper
正在生成论文摘要