Cross-modal Variational Auto-encoder with Distributed Latent Spaces and Associators
arXiv (Cornell University)(2019)
摘要
In this paper, we propose a novel structure for a cross-modal data association, which is inspired by the recent research on the associative learning structure of the brain. We formulate the cross-modal association in Bayesian inference framework realized by a deep neural network with multiple variational auto-encoders and variational associators. The variational associators transfer the latent spaces between auto-encoders that represent different modalities. The proposed structure successfully associates even heterogeneous modal data and easily incorporates the additional modality to the entire network via the proposed cross-modal associator. Furthermore, the proposed structure can be trained with only a small amount of paired data since auto-encoders can be trained by unsupervised manner. Through experiments, the effectiveness of the proposed structure is validated on various datasets including visual and auditory data.
更多查看译文
关键词
Cross-Modal Retrieval
AI 理解论文
溯源树
样例
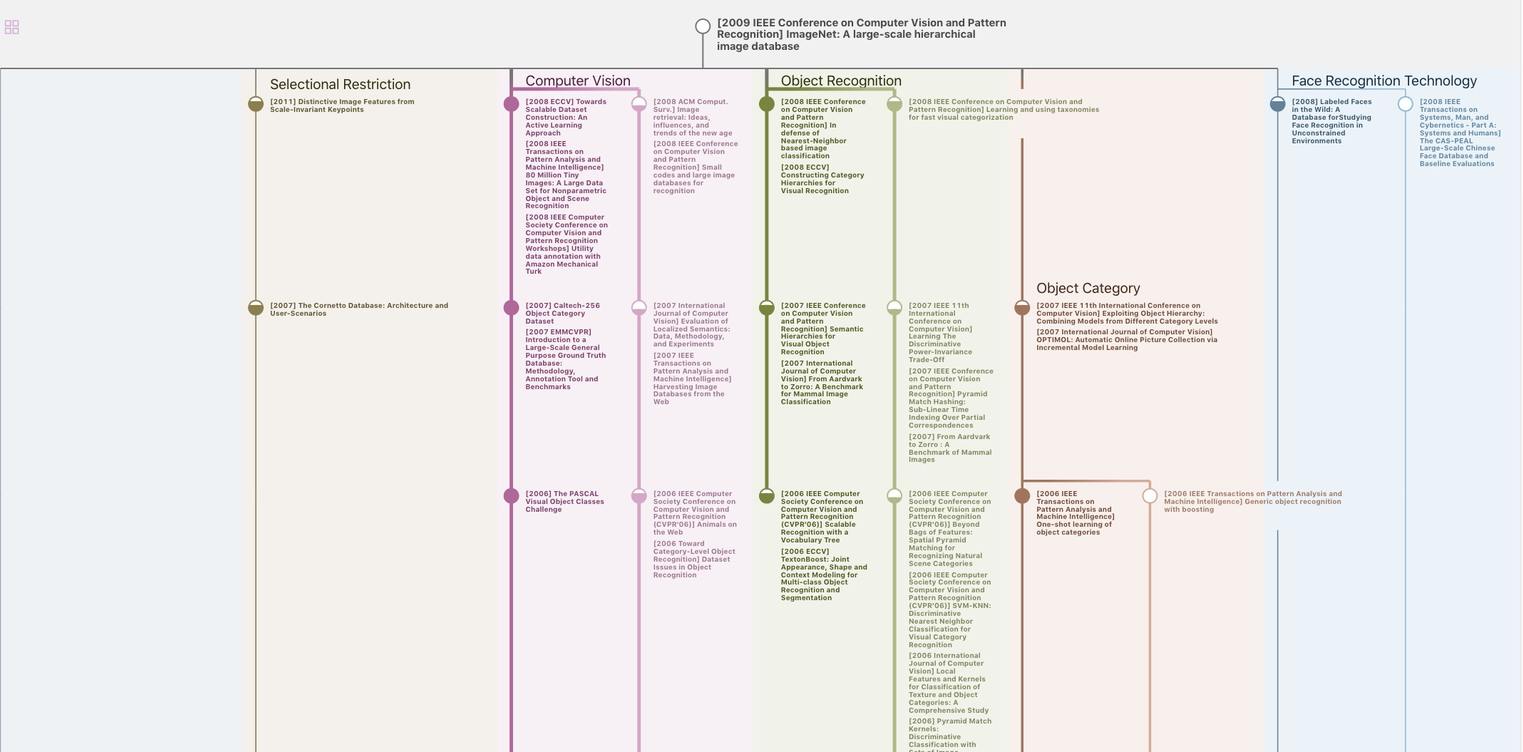
生成溯源树,研究论文发展脉络
Chat Paper
正在生成论文摘要