Grounding Language Attributes To Objects Using Bayesian Eigenobjects
2019 IEEE/RSJ INTERNATIONAL CONFERENCE ON INTELLIGENT ROBOTS AND SYSTEMS (IROS)(2019)
摘要
We develop a system to disambiguate object instances within the same class based on simple physical descriptions. The system takes as input a natural language phrase and a depth image containing a segmented object and predicts how similar the observed object is to the object described by the phrase. Our system is designed to learn from only a small amount of human-labeled language data and generalize to viewpoints not represented in the language-annotated depth image training set. By decoupling 3D shape representation from language representation, this method is able to ground language to novel objects using a small amount of language-annotated depth-data and a larger corpus of unlabeled 3D object meshes, even when these objects are partially observed from unusual viewpoints. Our system is able to disambiguate between novel objects, observed via depth images, based on natural language descriptions. Our method also enables viewpoint transfer; trained on human-annotated data on a small set of depth images captured from frontal viewpoints, our system successfully predicted object attributes from rear views despite having no such depth images in its training set. Finally, we demonstrate our approach on a Baxter robot, enabling it to pick specific objects based on human-provided natural language descriptions.
更多查看译文
关键词
object attributes,human-provided natural language descriptions,language attributes,object instances,physical descriptions,natural language phrase,segmented object,observed object,human-labeled language data,language-annotated depth image training set,3D shape representation,language representation,language-annotated depth-data,unusual viewpoints,human-annotated data,Bayesian eigenobjects
AI 理解论文
溯源树
样例
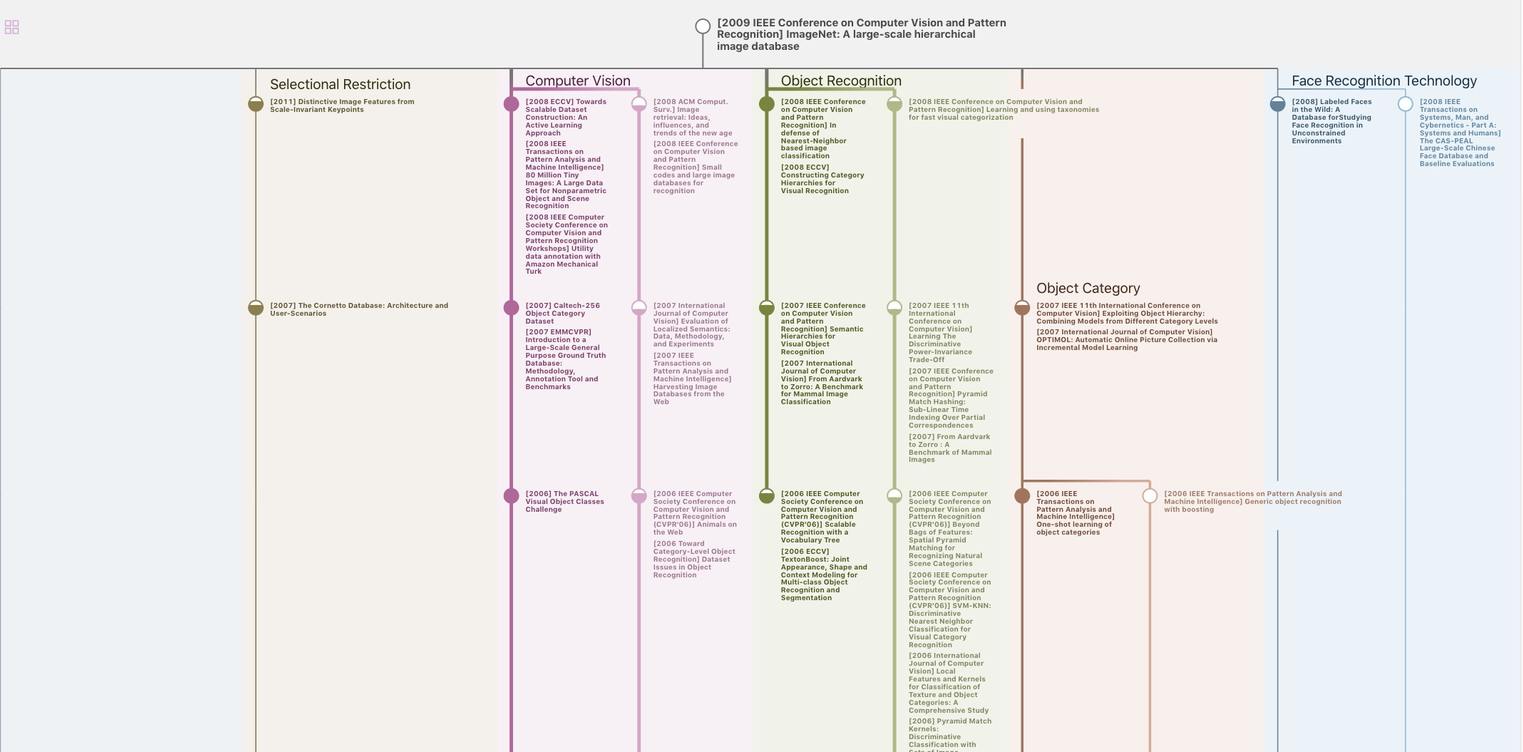
生成溯源树,研究论文发展脉络
Chat Paper
正在生成论文摘要