Fairness Constraints: A Flexible Approach for Fair Classification.
JOURNAL OF MACHINE LEARNING RESEARCH(2019)
摘要
Algorithmic decision making is employed in an increasing number of real-world applications to aid human decision making. While it has shown considerable promise in terms of improved decision accuracy, in some scenarios, its outcomes have been also shown to impose an unfair (dis) advantage on people from certain social groups (e.g., women, blacks). In this context, there is a need for computational techniques to limit unfairness in algorithmic decision making. In this work, we take a step forward to ful fi ll that need and introduce a flexible constraint-based framework to enable the design of fair margin-based classi fi ers. The main technical innovation of our framework is a general and intuitive measure of decision boundary unfairness, which serves as a tractable proxy to several of the most popular computational de fi nitions of unfairness from the literature. Leveraging our measure, we can reduce the design of fair margin-based classi fi ers to adding tractable constraints on their decision boundaries. Experiments on multiple synthetic and real-world datasets show that our framework is able to successfully limit unfairness, often at a small cost in terms of accuracy.
更多查看译文
关键词
Supervised learning,margin-based classifiers,fairness,discrimination,disparate impact
AI 理解论文
溯源树
样例
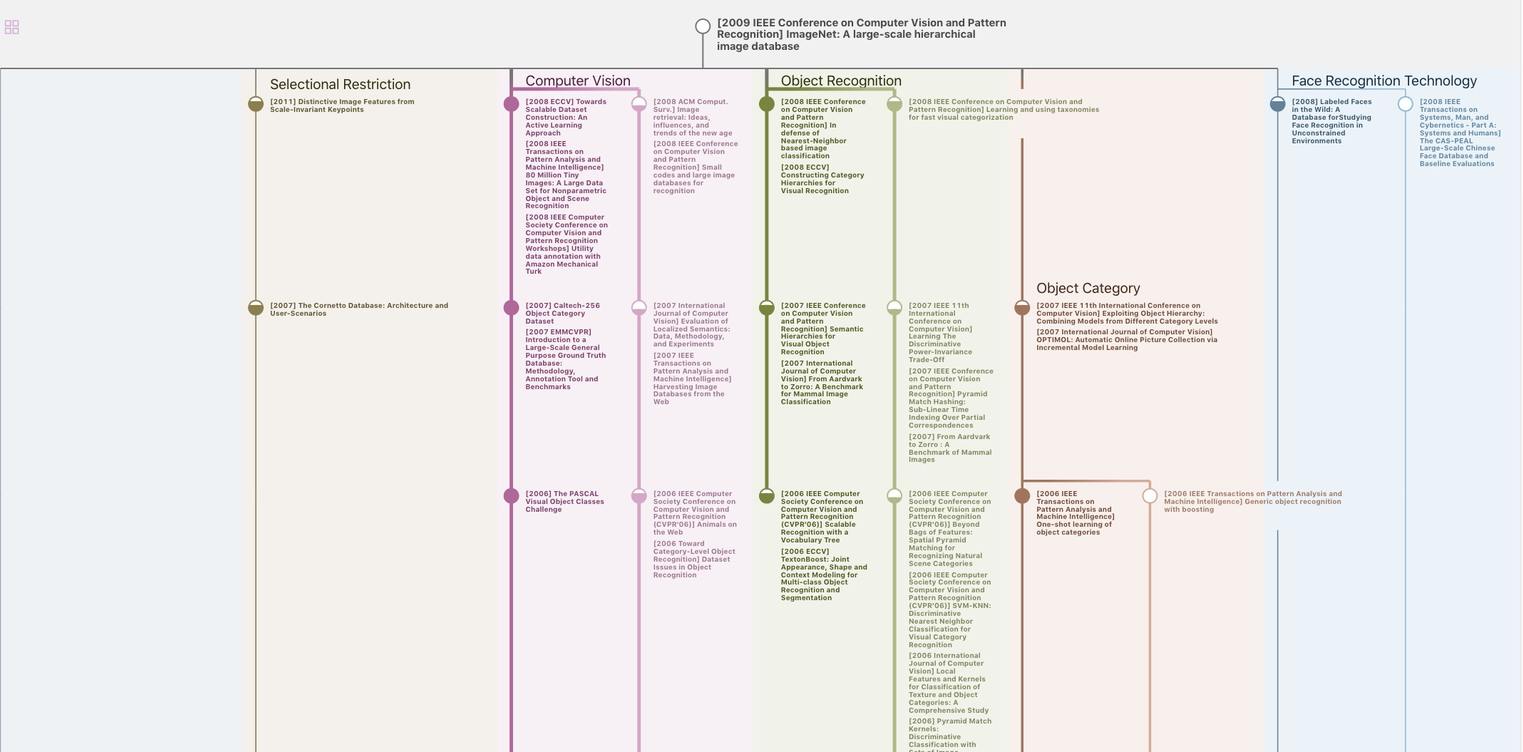
生成溯源树,研究论文发展脉络
Chat Paper
正在生成论文摘要