Patient-Specific Effects of Medication Using Latent Force Models with Gaussian Processes
INTERNATIONAL CONFERENCE ON ARTIFICIAL INTELLIGENCE AND STATISTICS, VOL 108(2019)
摘要
Multi-output Gaussian processes (GPs) are a flexible Bayesian nonparametric framework that has proven useful in jointly modeling the physiological states of patients in medical time series data. However, capturing the short-term effects of drugs and therapeutic interventions on patient physiological state remains challenging. We propose a novel approach that models the effect of interventions as a hybrid Gaussian process composed of a GP capturing patient physiology convolved with a latent force model capturing effects of treatments on specific physiological features. This convolution of a multi-output GP with a GP including a causal time-marked kernel leads to a well-characterized model of the patients' physiological state responding to interventions. We show that our model leads to analytically tractable cross-covariance functions, allowing scalable inference. Our hierarchical model includes estimates of patient-specific effects but allows sharing of support across patients. Our approach achieves competitive predictive performance on challenging hospital data, where we recover patient-specific response to the administration of three common drugs: one antihypertensive drug and two anticoagulants.
更多查看译文
关键词
latent force models,gaussian processes,medication,effects,patient-specific
AI 理解论文
溯源树
样例
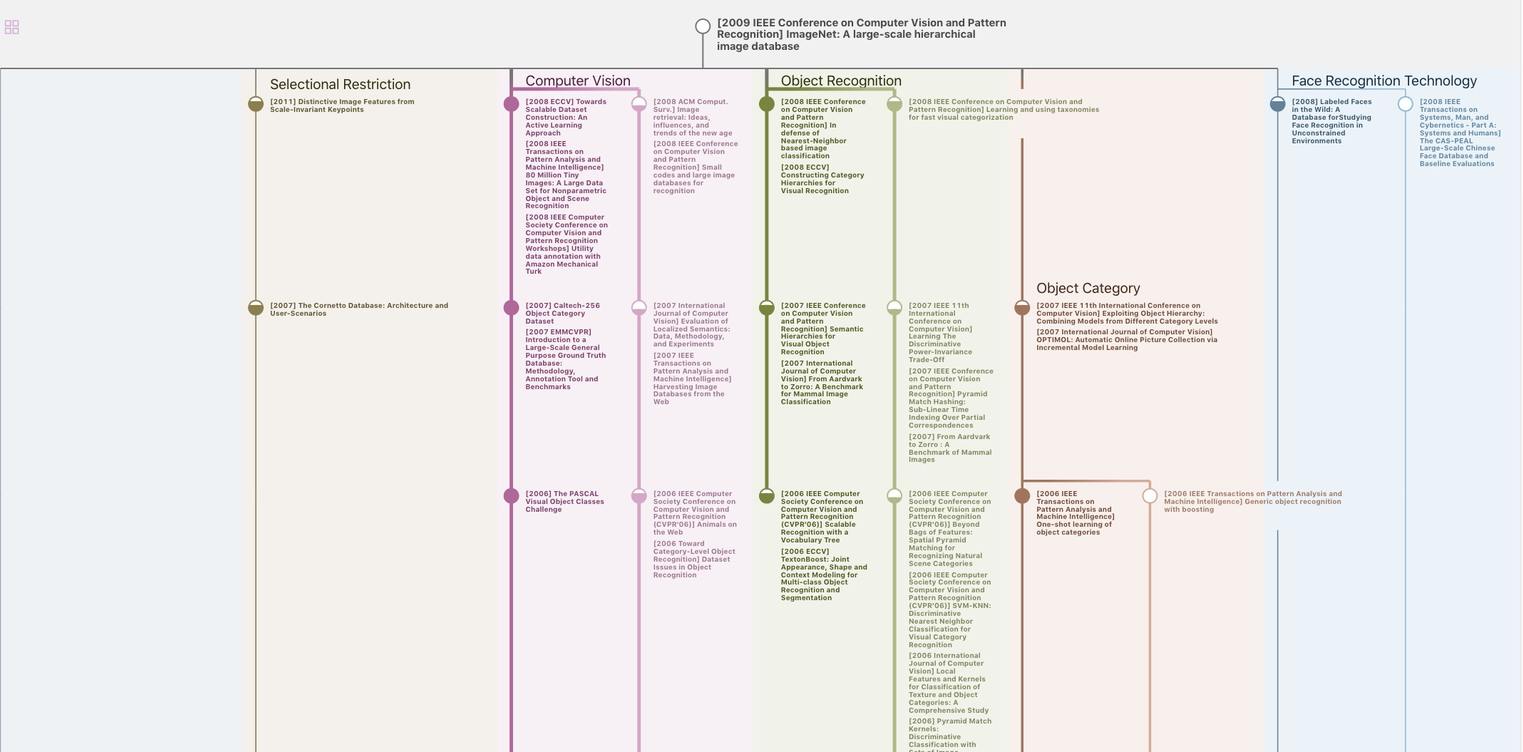
生成溯源树,研究论文发展脉络
Chat Paper
正在生成论文摘要