Self-supervised Body Image Acquisition Using a Deep Neural Network for Sensorimotor Prediction
Joint IEEE International Conference on Development and Learning and on Epigenetic Robotics(2019)
摘要
This work investigates how a naive agent can acquire its own body image in a self-supervised way, based on the predictability of its sensorimotor experience. Our working hypothesis is that, due to its temporal stability, an agent's body produces more consistent sensory experiences than the environment, which exhibits a greater variability. Given its motor experience, an agent can thus reliably predict what appearance its body should have. This intrinsic predictability can be used to automatically isolate the body image from the rest of the environment. We propose a two-branches deconvolutional neural network to predict the visual sensory state associated with an input motor state, as well as the prediction error associated with this input. We train the network on a dataset of first-person images collected with a simulated Pepper robot, and show how the network outputs can be used to automatically isolate its visible arm from the rest of the environment. Finally, the quality of the body image produced by the network is evaluated.
更多查看译文
关键词
self-supervised body image acquisition,deep neural network,sensorimotor prediction,naive agent,sensorimotor experience,working hypothesis,consistent sensory experiences,motor experience,intrinsic predictability,visual sensory state,input motor state,prediction error,first-person images,two-branch deconvolutional neural network
AI 理解论文
溯源树
样例
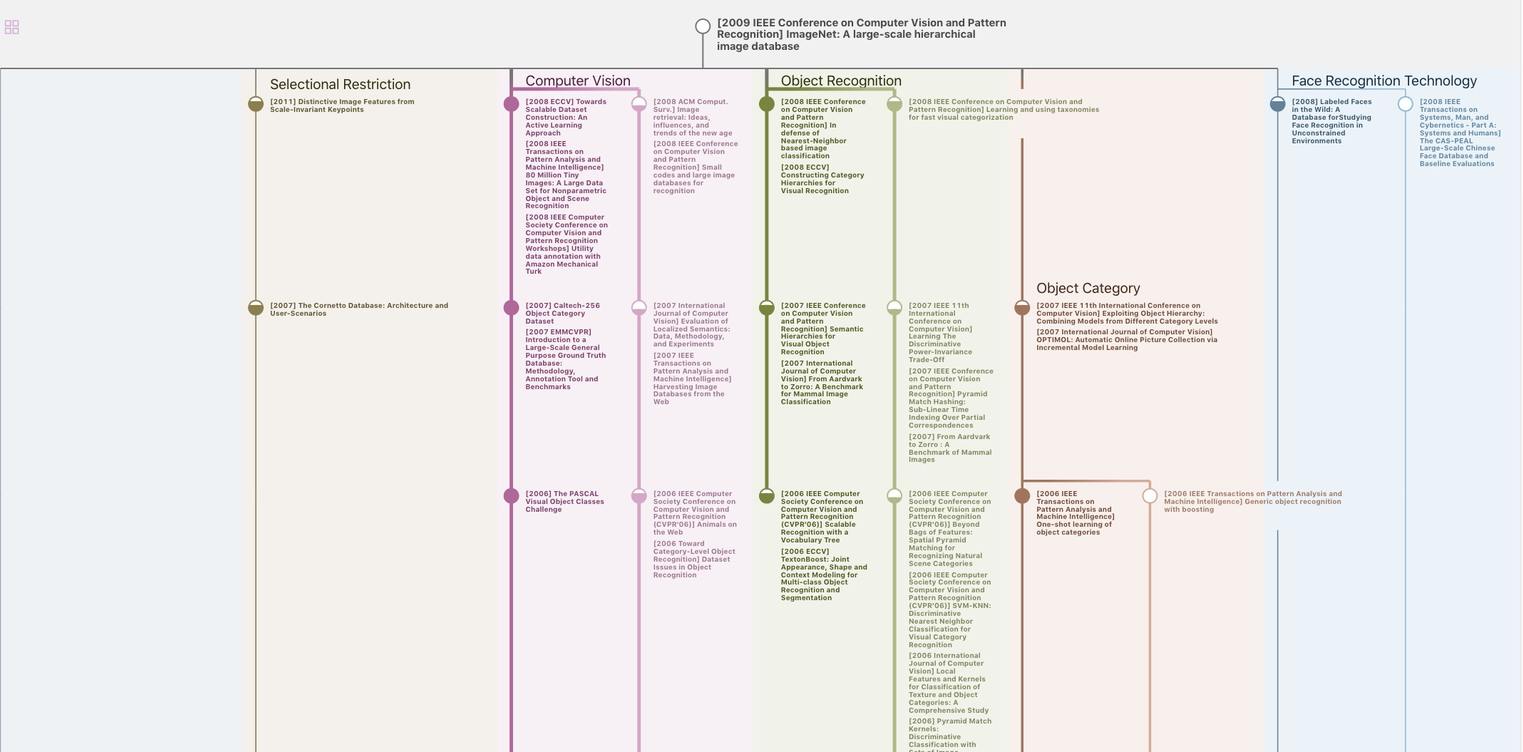
生成溯源树,研究论文发展脉络
Chat Paper
正在生成论文摘要