Streaming Variational Monte Carlo.
IEEE TRANSACTIONS ON PATTERN ANALYSIS AND MACHINE INTELLIGENCE(2019)
摘要
Nonlinear state-space models are powerful tools to describe dynamical structures in complex time series. In a streaming setting where data are processed one sample at a time, simultaneously inferring the state and their nonlinear dynamics has posed significant challenges in practice. We develop a novel online learning framework, leveraging variational inference and sequential Monte Carlo, which enables flexible and accurate Bayesian joint filtering. Our method provides a filtering posterior arbitrarily close to the true filtering distribution for a wide class of dynamics models and observation models. Specifically, the proposed framework can efficiently infer a posterior over the dynamics using sparse Gaussian processes. Constant time complexity per sample makes our approach amenable to online learning scenarios and suitable for real-time applications.
更多查看译文
关键词
Nonlinear state-space modeling,online filtering,Bayesian machine learning
AI 理解论文
溯源树
样例
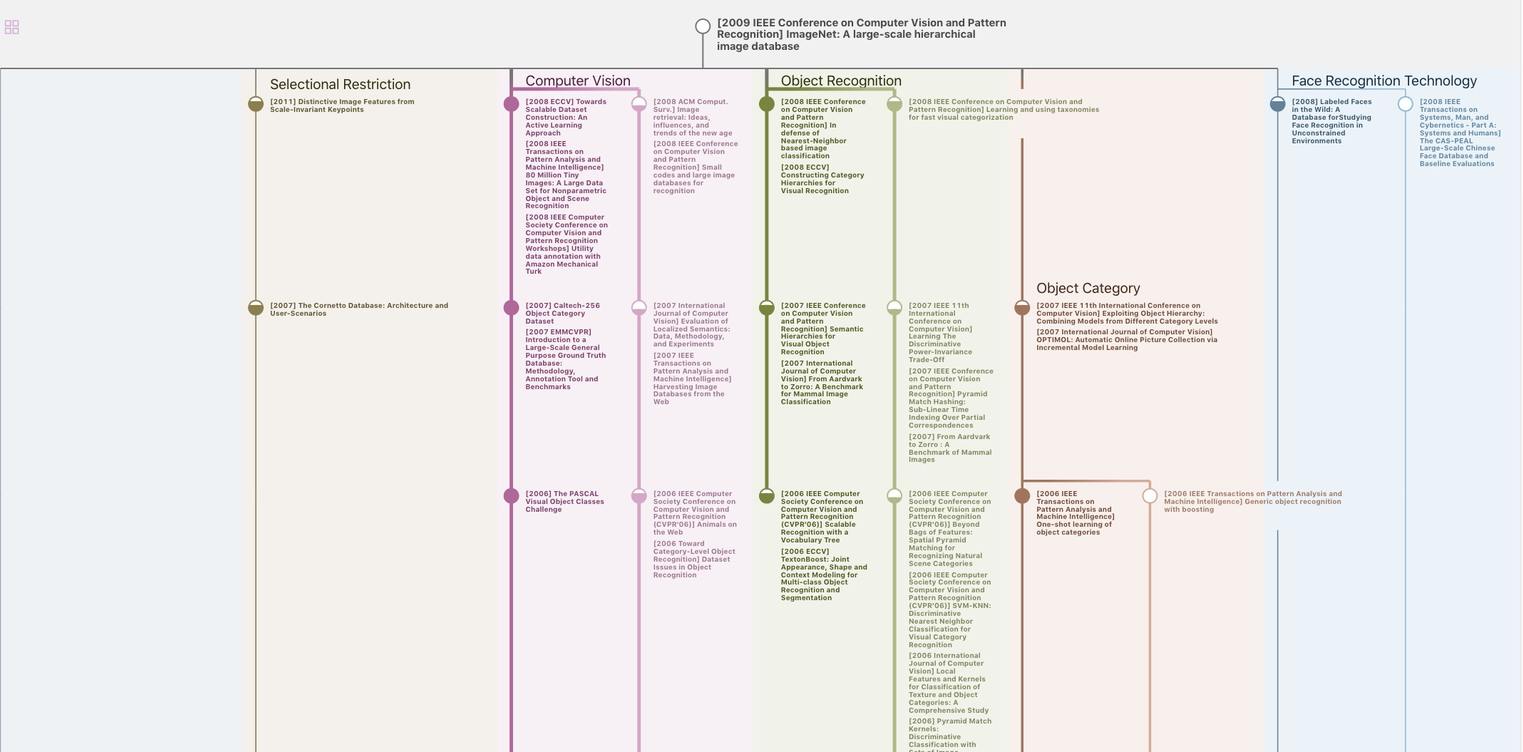
生成溯源树,研究论文发展脉络
Chat Paper
正在生成论文摘要