Improving Textual Network Embedding With Global Attention Via Optimal Transport
57TH ANNUAL MEETING OF THE ASSOCIATION FOR COMPUTATIONAL LINGUISTICS (ACL 2019)(2019)
摘要
Constituting highly informative network embeddings is an important tool for network analysis. It encodes network topology, along with other useful side information, into low-dimensional node-based feature representations that can be exploited by statistical modeling. This work focuses on learning context-aware network embeddings augmented with text data. We reformulate the network-embedding problem, and present two novel strategies to improve over traditional attention mechanisms: (i) a content-aware sparse attention module based on optimal transport, and (ii) a high-level attention parsing module. Our approach yields naturally sparse and self-normalized relational inference. It can capture long-term interactions between sequences, thus addressing the challenges faced by existing textual network embedding schemes. Extensive experiments are conducted to demonstrate our model can consistently outperform alternative state-of-the-art methods.
更多查看译文
关键词
textual network,global attention,transport
AI 理解论文
溯源树
样例
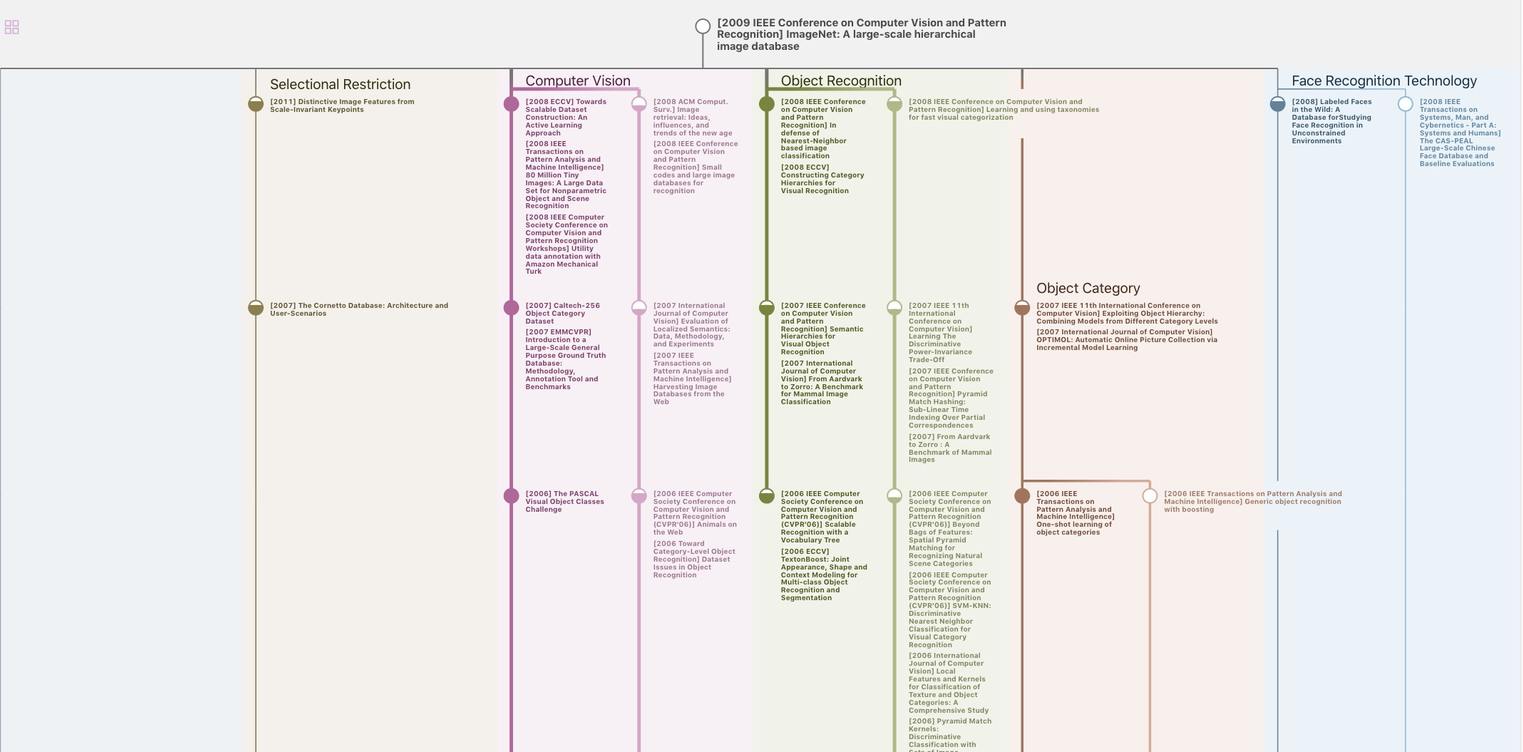
生成溯源树,研究论文发展脉络
Chat Paper
正在生成论文摘要