Hankel Matrix Factorization for Tagged Time Series to Recover Missing Values During Blackouts
2019 IEEE 35th International Conference on Data Engineering (ICDE)(2019)
摘要
Recovering missing values in time series is critical when performing time series analysis. And the blackouts issue studied in this paper, described as losing all the data during a certain period, is among the most urgent and challenging issues. While the existing approaches for missing value recovery in time series could not handle this issue properly, in this work, we proposes a Hankel matrix factorization-based approach for tagged time series called HKMF-T, following the idea of decomposing a data sequence into the smooth trend and the external impact components. By transforming the data sequence into its Hankel matrix form, HKMF-T models the smooth trend implied by high-order temporal correlations as the product of two low-rank matrices, and learns the external impacts indicated by a corresponding tag sequence. Through extensive experiments conducted on three real-world data sets, HKMF-T shows its effectiveness by outperforming all baseline methods for blackouts with durations longer than nine sampling intervals.
更多查看译文
关键词
Time series analysis,Matrix decomposition,Market research,Correlation,Time complexity,Task analysis,Estimation
AI 理解论文
溯源树
样例
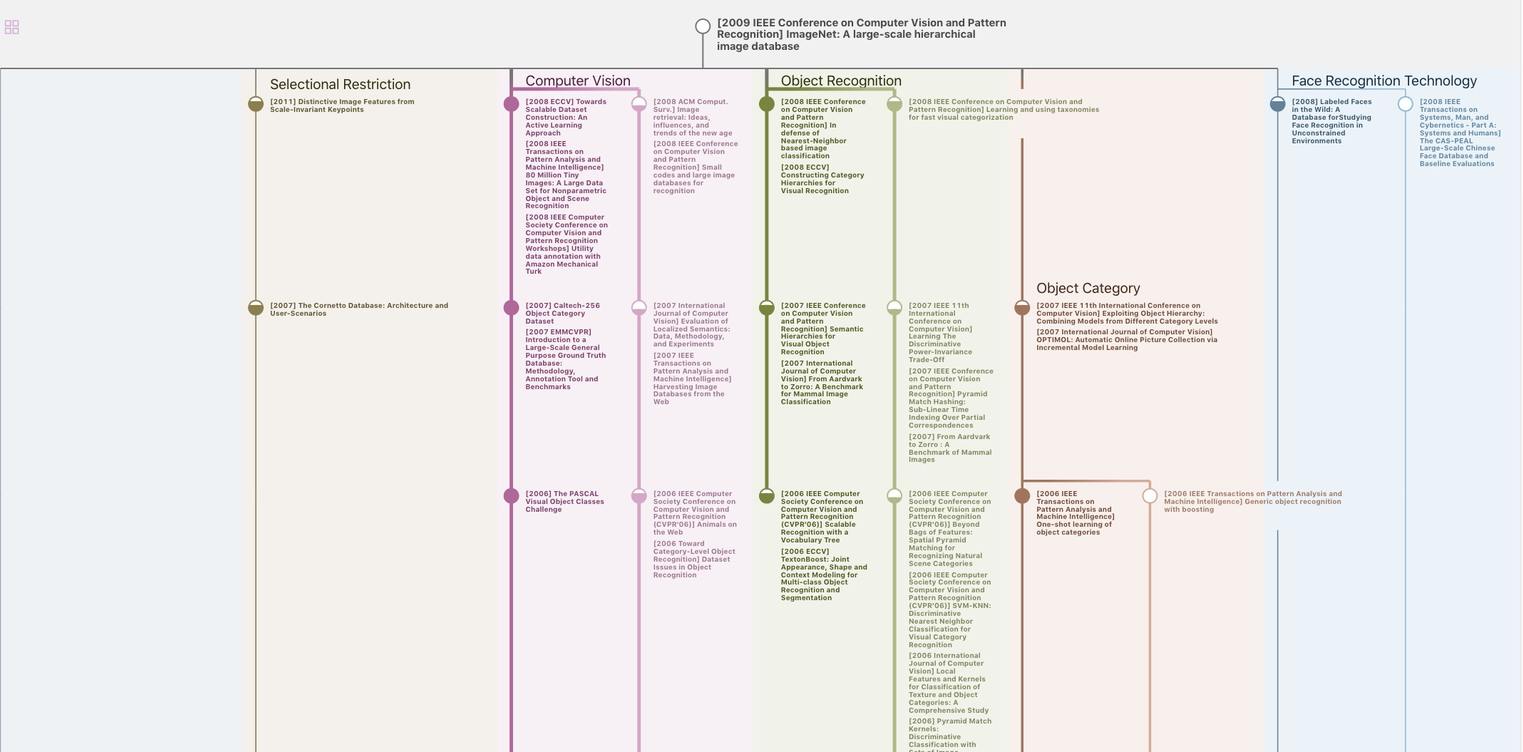
生成溯源树,研究论文发展脉络
Chat Paper
正在生成论文摘要