Preference-based interactive multi-document summarisation
Information Retrieval Journal(2019)
摘要
Interactive NLP is a promising paradigm to close the gap between automatic NLP systems and the human upper bound. Preference-based interactive learning has been successfully applied, but the existing methods require several thousand interaction rounds even in simulations with perfect user feedback. In this paper, we study preference-based interactive summarisation. To reduce the number of interaction rounds, we propose the Active Preference-based ReInforcement Learning (APRIL) framework. APRIL uses active learning to query the user, preference learning to learn a summary ranking function from the preferences, and neural Reinforcement learning to efficiently search for the (near-)optimal summary. Our results show that users can easily provide reliable preferences over summaries and that APRIL outperforms the state-of-the-art preference-based interactive method in both simulation and real-user experiments.
更多查看译文
关键词
Interactive Natural Language Processing,Document summarisation,Reinforcement learning,Active learning,Preference learning
AI 理解论文
溯源树
样例
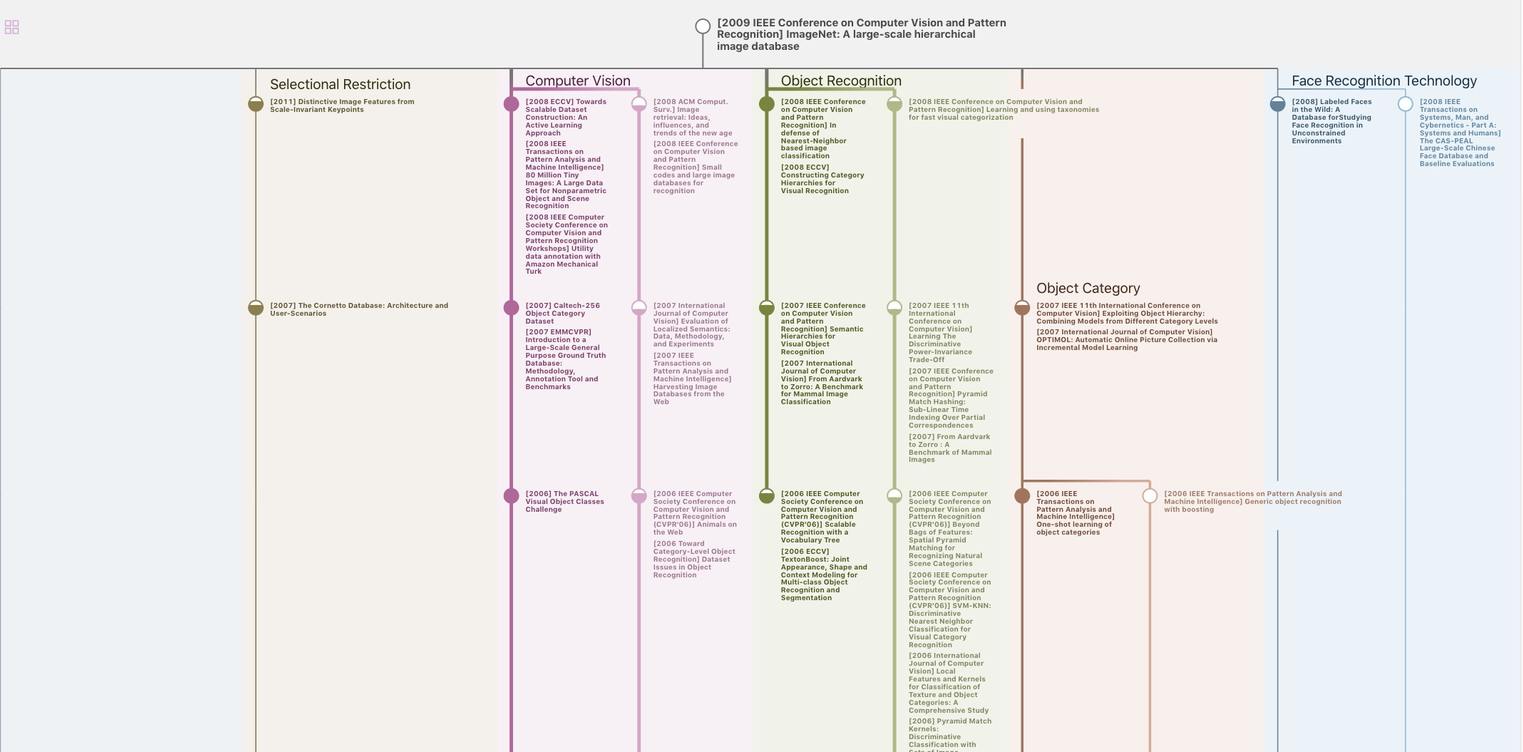
生成溯源树,研究论文发展脉络
Chat Paper
正在生成论文摘要