Estimation Rates for Sparse Linear Cyclic Causal Models
UAI(2020)
摘要
Causal models are important tools to understand complex phenomena and predict the outcome of controlled experiments, also known as interventions. In this work, we present statistical rates of estimation for linear cyclic causal models under the assumption of homoscedastic Gaussian noise by analyzing both the LLC estimator introduced by Hyttinen, Eberhardt and Hoyer and a novel two-step penalized maximum likelihood estimator. We establish asymptotic near minimax optimality for the maximum likelihood estimator over a class of sparse causal graphs in the case of near-optimally chosen interventions. Moreover, we find evidence for practical advantages of this estimator compared to LLC in synthetic numerical experiments.
更多查看译文
AI 理解论文
溯源树
样例
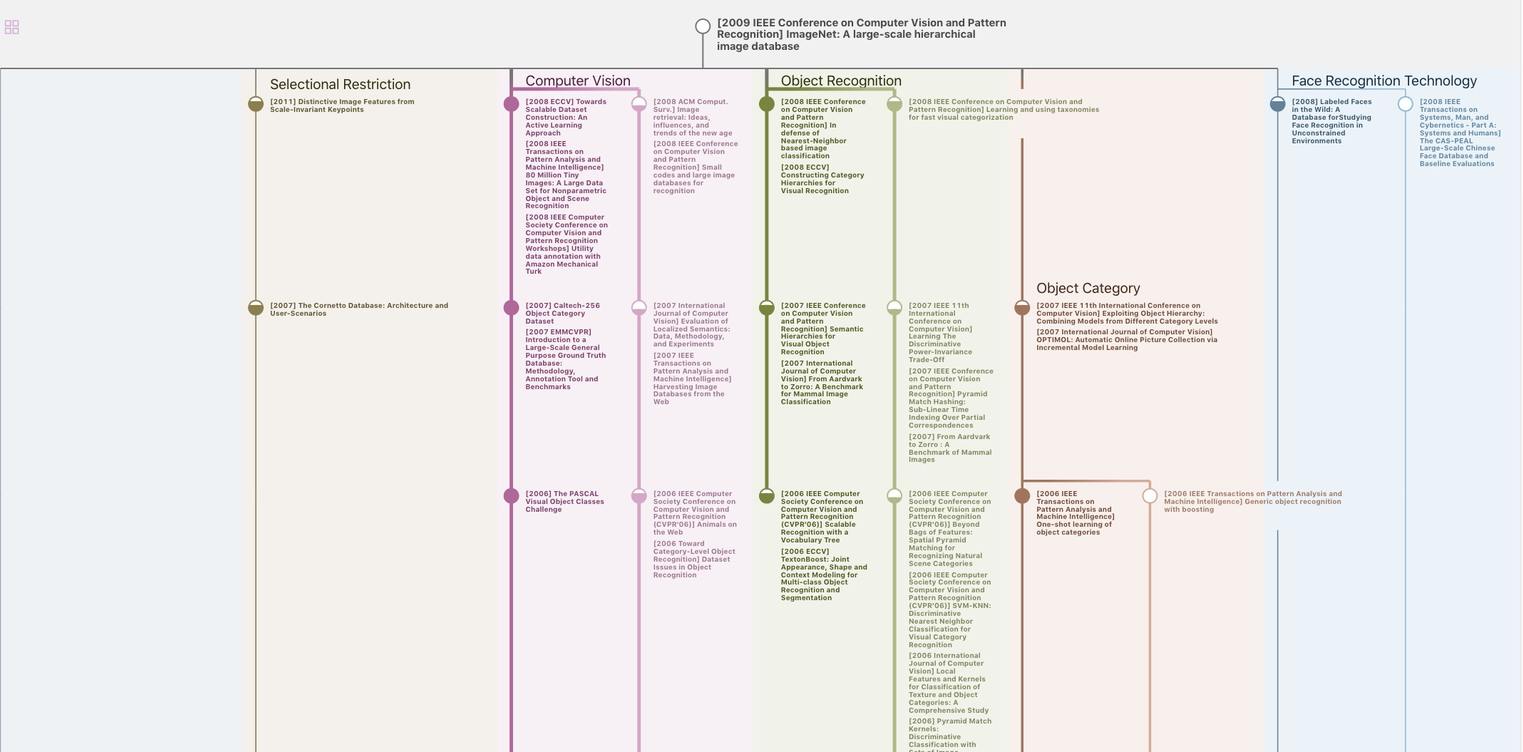
生成溯源树,研究论文发展脉络
Chat Paper
正在生成论文摘要