Asymptotically Optimal Change Point Detection for Composite Hypothesis in State Space Models
IEEE Transactions on Information Theory(2021)
摘要
This paper investigates change point detection in state space models, in which the pre-change distribution f
θ0
is given, while the post-distribution f
θ
after change is unknown. The problem is to raise an alarm as soon as possible after the distribution changes from f
θ0
to f
θ
, under a restriction on the false alarms. We investigate theoretical properties of a weighted Shiryayev-Roberts-Pollak (SRP) change point detection rule in state space models. By making use of a Markov chain representation for the likelihood function, exponential embedding of the induced Markovian transition operator, nonlinear Markov renewal theory, and sequential hypothesis testing theory for Markov random walks, we show that the weighted SRP procedure is second-order asymptotically optimal. To this end, we derive an asymptotic approximation for the expected stopping time of such a stopping scheme when the change time w = 1. To illustrate our method we apply the results to two types of state space models: general state Markov chains and linear state space models. Simulation study is given to illustrate the method.
更多查看译文
关键词
Asymptotic optimality,change point detection,first passage time,iterated random functions system,nonlinear Markov renewal theory,sequential analysis,Shiryayev-Roberts-Pollak procedure
AI 理解论文
溯源树
样例
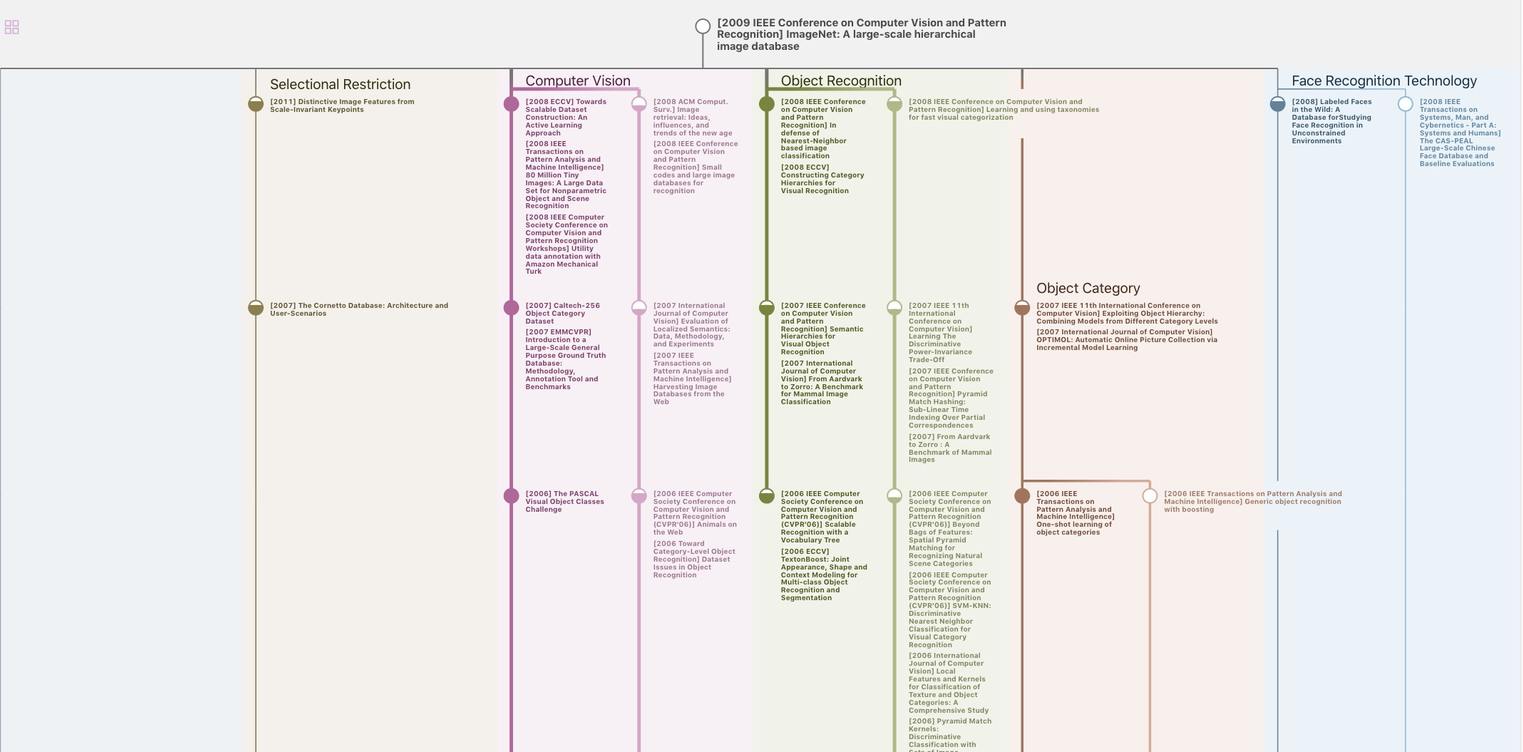
生成溯源树,研究论文发展脉络
Chat Paper
正在生成论文摘要