Predictive models of aqueous solubility of organic compounds built on A large dataset of high integrity.
Bioorganic & Medicinal Chemistry(2019)
摘要
Aqueous solubility is one of the most important properties in drug discovery, as it has profound impact on various drug properties, including biological activity, pharmacokinetics (PK), toxicity, and in vivo efficacy. Both kinetic and thermodynamic solubilities are determined during different stages of drug discovery and development. Since kinetic solubility is more relevant in preclinical drug discovery research, especially during the structure optimization process, we have developed predictive models for kinetic solubility with in-house data generated from 11,780 compounds collected from over 200 NCATS intramural research projects. This represents one of the largest kinetic solubility datasets of high quality and integrity. Based on the customized atom type descriptors, the support vector classification (SVC) models were trained on 80% of the whole dataset, and exhibited high predictive performance for estimating the solubility of the remaining 20% compounds within the test set. The values of the area under the receiver operating characteristic curve (AUC-ROC) for the compounds in the test sets reached 0.93 and 0.91, when the threshold for insoluble compounds was set to 10 and 50 μg/mL respectively. The predictive models of aqueous solubility can be used to identify insoluble compounds in drug discovery pipeline, provide design ideas for improving solubility by analyzing the atom types associated with poor solubility and prioritize compound libraries to be purchased or synthesized.
更多查看译文
关键词
Kinetic solubility,Atom typing descriptors,Support vector classification (SVC),In silico ADME model,Prediction
AI 理解论文
溯源树
样例
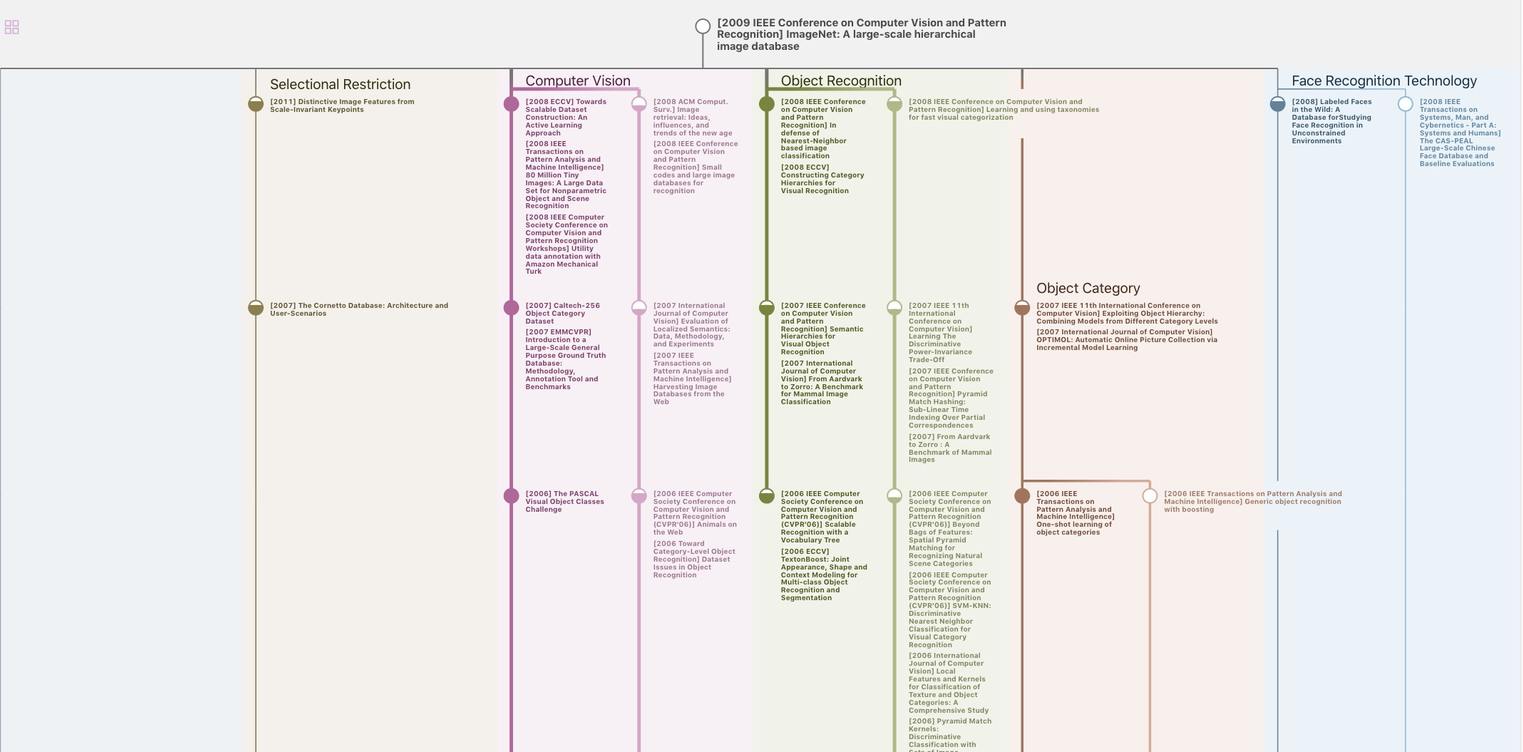
生成溯源树,研究论文发展脉络
Chat Paper
正在生成论文摘要