The calibrated model-based concordance improved assessment of discriminative ability in patient clusters of limited sample size
Diagnostic and Prognostic Research(2019)
摘要
Background Discriminative ability is an important aspect of prediction model performance, but challenging to assess in clustered (e.g., multicenter) data. Concordance (c)-indexes may be too extreme within small clusters. We aimed to define a new approach for the assessment of discriminative ability in clustered data. Methods We assessed discriminative ability of a prediction model for the binary outcome mortality after traumatic brain injury within centers of the CRASH trial. With multilevel logistic regression analysis, we estimated cluster-specific calibration slopes which we used to obtain the recently proposed calibrated model-based concordance ( c-mbc ) within each cluster . We compared the c-mbc with the naïve c-index in centers of the CRASH trial and in simulations of clusters with varying calibration slopes. Results The c-mbc was less extreme in distribution than the c-index in 19 European centers (internal validation; n = 1716) and 36 non-European centers (external validation; n = 3135) of the CRASH trial. In simulations, the c-mbc was biased but less variable than the naïve c-index, resulting in lower root mean squared errors. Conclusions The c-mbc , based on multilevel regression analysis of the calibration slope, is an attractive alternative to the c-index as a measure of discriminative ability in multicenter studies with patient clusters of limited sample size.
更多查看译文
关键词
Prediction model, Model performance, Discrimination, Concordance, Clustered data, Multilevel regression, Traumatic brain injury
AI 理解论文
溯源树
样例
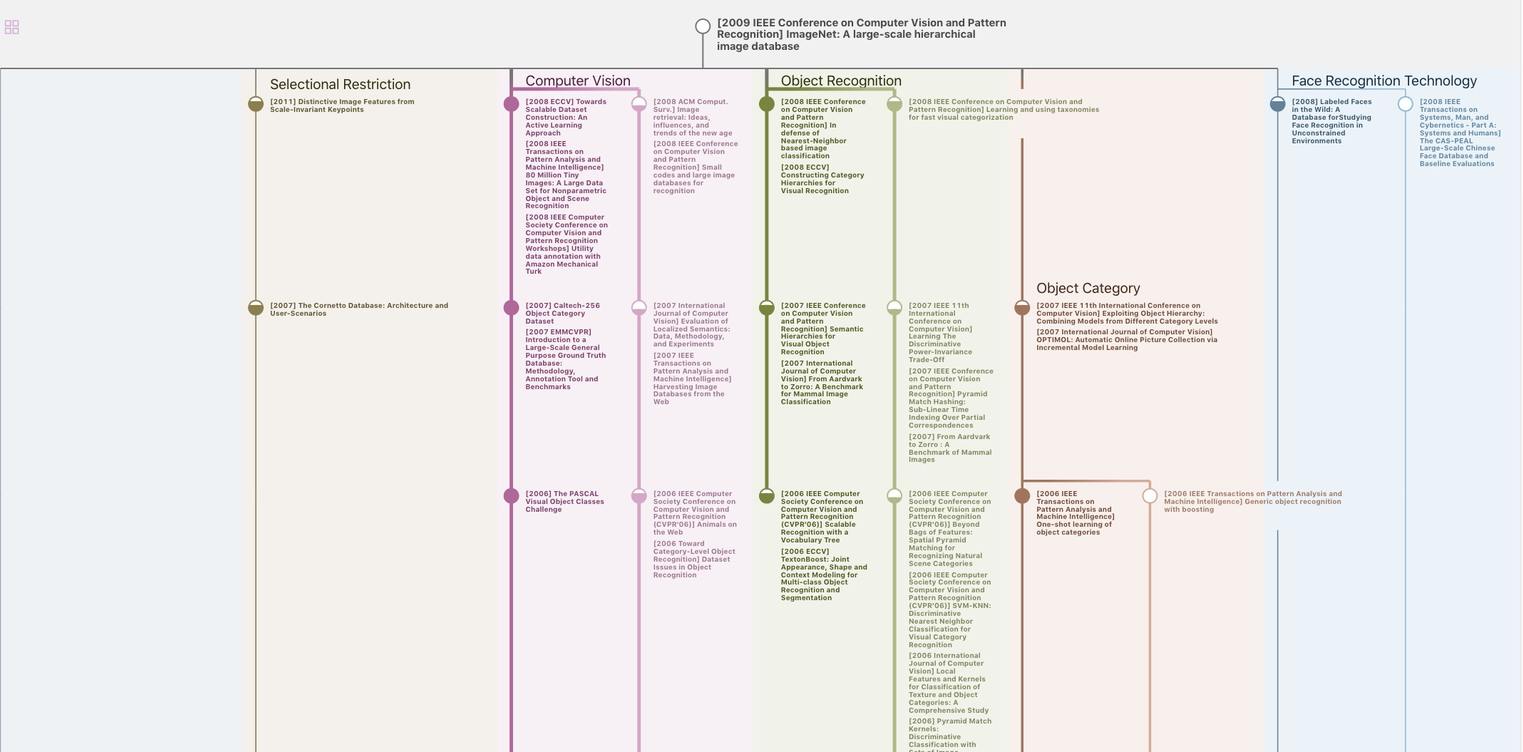
生成溯源树,研究论文发展脉络
Chat Paper
正在生成论文摘要