Multi-spatial Scale Event Detection from Geo-tagged Tweet Streams Via Power-law Verification
CoRR(2019)
摘要
Compared with traditional news media, social media nowadays provides a richer and more timely source of news. We are interested in multi-spatial level event detection from geo-tagged tweet streams. Specifically, in this paper we (1) examine the statistical characteristic for the time series of the number of geo-tagged tweets posted from specific regions during a short time interval, e.g., ten seconds or one minute; (2) verify from over thirty datasets that while almost all such time series exhibit self-similarity, those that correspond to events, especially short-term and unplanned outbursts, follow a power-law distribution; (3) demonstrate that these findings can be applied to facilitate event detection from tweet streams. We propose two algorithms—Power-law basic and Power-law advanced, where Power-law basic only checks the existence of power-law distributions in the time series from tweet streams at multi-spatial scales, without looking into the content of each tweet, and Power-law advanced integrates power-law verification with semantic analysis via word embedding. Our experiments on multiple datasets show that by considering spatio-temporal statistical distributions of tweets alone, the seemingly naive algorithm of Power-law basic achieves comparable results with more advanced event detection methods, while the semantic analysis enhanced version, Power-law advanced, can significantly increase both the precision and the recall.
更多查看译文
关键词
self-similarity,power-law distribution,multi-spatial event detection
AI 理解论文
溯源树
样例
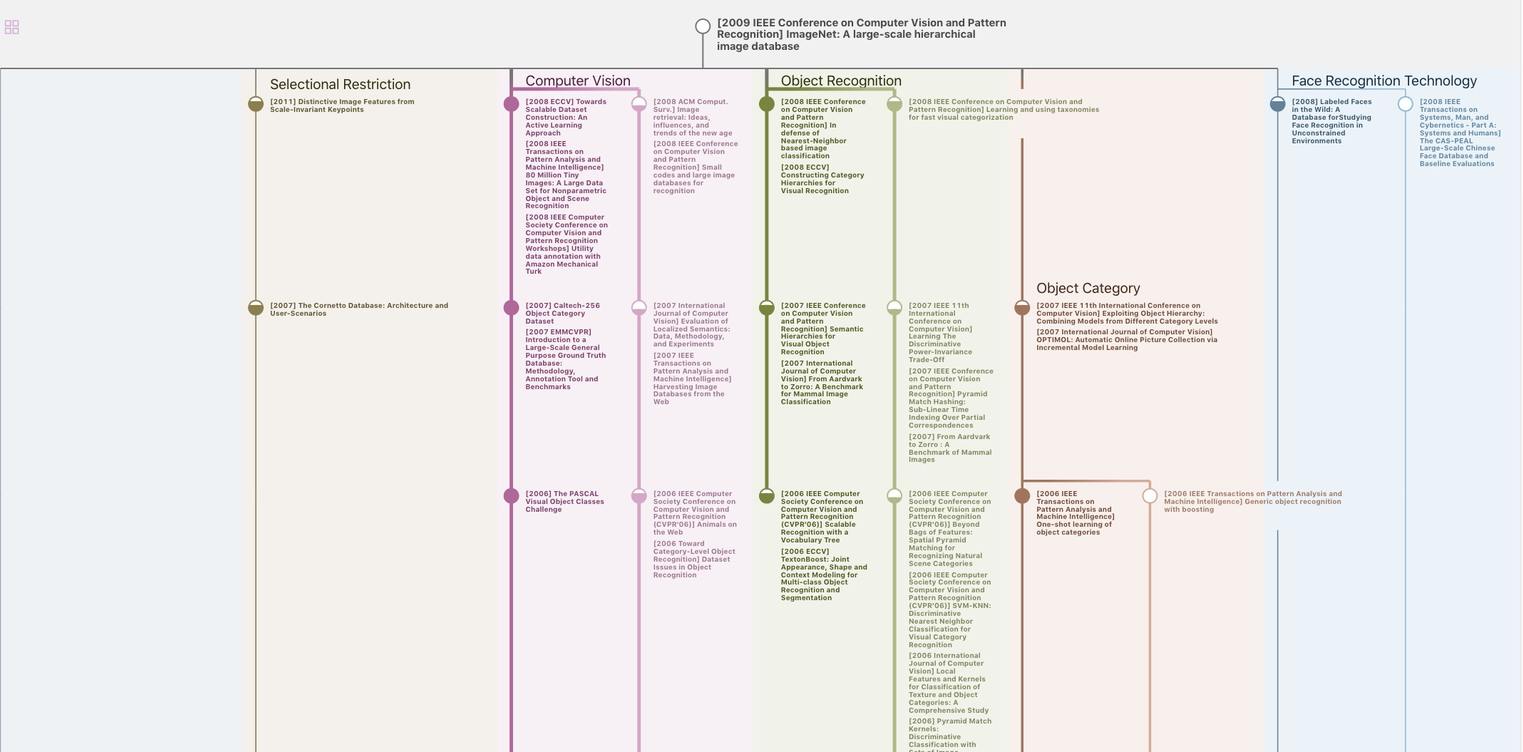
生成溯源树,研究论文发展脉络
Chat Paper
正在生成论文摘要