Scalable Community Detection over Geo-Social Network
GIS(2019)
摘要
We consider a community finding problem called Co-located Community Detection (CCD) over geo-social networks, which retrieves communities that satisfy both high structural tightness and spatial closeness constraints. To provide a solution that benefits from existing studies on community detection, we decouple the spatial constraint from graph structural constraint and propose a uniform CCD framework which gives users the freedom to choose customized measurements for social cohesiveness (e.g., k-core or k-truss). For the spatial closeness constraint, we apply the bounded radius spatial constraint and develop an exact algorithm together with effective pruning rules. To further improve the efficiency and make our framework scale to a very large scale of data, we propose a near-linear time approximation algorithm with a constant approximation ratio (√(2)). We conduct extensive experiments on both synthetic and real-world datasets to demonstrate the efficiency and effectiveness of our algorithms.
更多查看译文
关键词
Geo-Social Network,Co-located Community Detection,Computational Geometry,Big Spatial Data
AI 理解论文
溯源树
样例
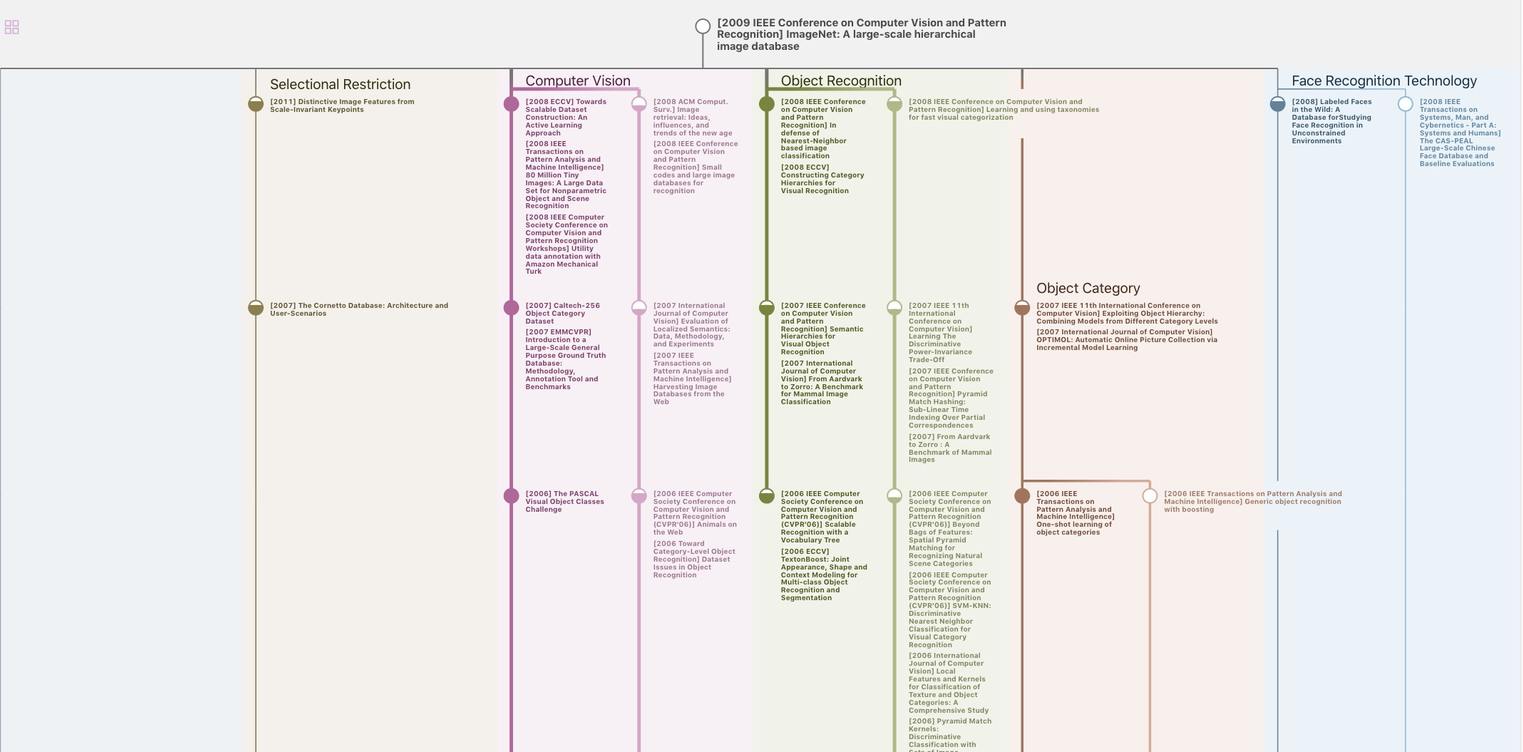
生成溯源树,研究论文发展脉络
Chat Paper
正在生成论文摘要