On The Complexity Of An Augmented Lagrangian Method For Nonconvex Optimization Ima
Ima Journal of Numerical Analysis(2021)
摘要
In this paper we study the worst-case complexity of an inexact augmented Lagrangian method for nonconvex constrained problems. Assuming that the penalty parameters are bounded we prove a complexity bound of O(vertical bar log(epsilon)vertical bar) outer iterations for the referred algorithm to generate an epsilon-approximate KKT point for epsilon is an element of (0, 1). When the penalty parameters are unbounded we prove an outer iteration complexity bound of O(epsilon(-2/(alpha-1))), where alpha > 1 controls the rate of increase of the penalty parameters. For linearly constrained problems these bounds yield to evaluation complexity bounds of O(vertical bar log(epsilon)vertical bar(2)epsilon(-)(2)) and O(epsilon(-(2(2+)(alpha)/)(alpha-1+2))), respectively, when appropriate first-order methods (p = 1) are used to approximately solve the unconstrained subproblems at each iteration. In the case of problems having only linear equality constraints the latter bounds are improved to O(vertical bar log(epsilon)vertical bar(2)epsilon(-)((p+1)/p)) and O(epsilon(-(4/)(alpha-1+p+1/p))), respectively, when appropriate p-order methods (p >= 2) are used as inner solvers.
更多查看译文
关键词
nonlinear programming, augmented Lagrangian methods, tensor methods, worst-case complexity
AI 理解论文
溯源树
样例
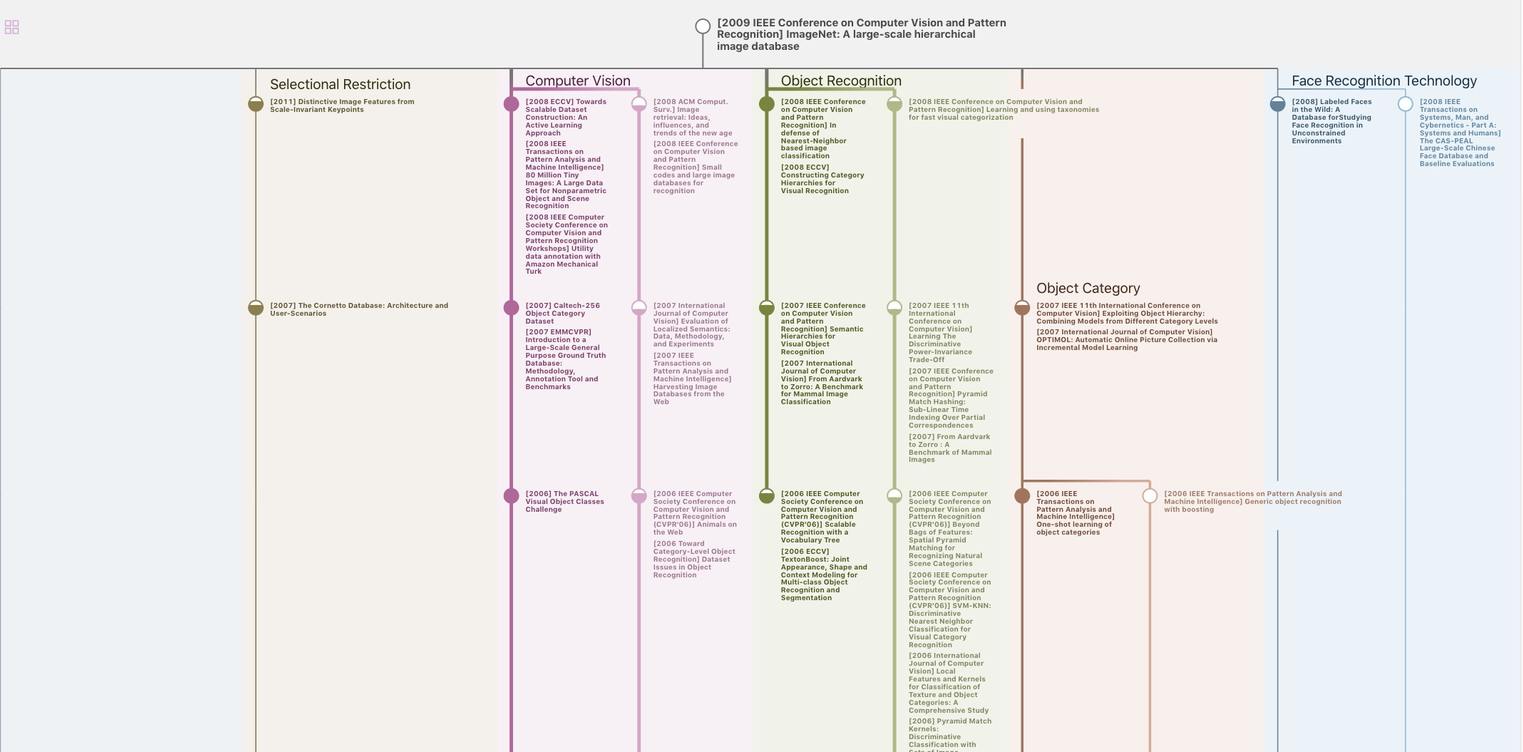
生成溯源树,研究论文发展脉络
Chat Paper
正在生成论文摘要