Filling missing values by local reconstruction for incomplete label distribution learning.
IJWMC(2019)
摘要
Label distribution learning (LDL) deals with the problems when we care more about the relative importance of different labels in the description of an instance, where labels are associated with each instance to some degree. LDL has achieved great success in many applications, but most existing LDL methods cannot handle learning tasks with incomplete annotation information. In this paper, we propose a novel incomplete label distribution learning method based on local reconstruction (IncomLDL-LR). Both the feature matrix and label information are included in a unified Principal Component Analysis (PCA) model to describe the intrinsic structure of original data in the supervised low-dimensional space. Based on the reasonable assumption that the incomplete label of each instance can be linearly reconstructed from its neighbours' labels, IncomLDL-LR gradually recovers the missing label values by the averaged column score of corresponding neighbours in the PCA space. The proposed algorithms are compared with state-of-the-art algorithms using five LDL evaluation metrics on 15 public datasets. Extensive experiments validate the effectiveness of our proposal.
更多查看译文
关键词
incomplete label distribution learning,local reconstruction,neighbourhood information,label distribution learning,principal component analysis
AI 理解论文
溯源树
样例
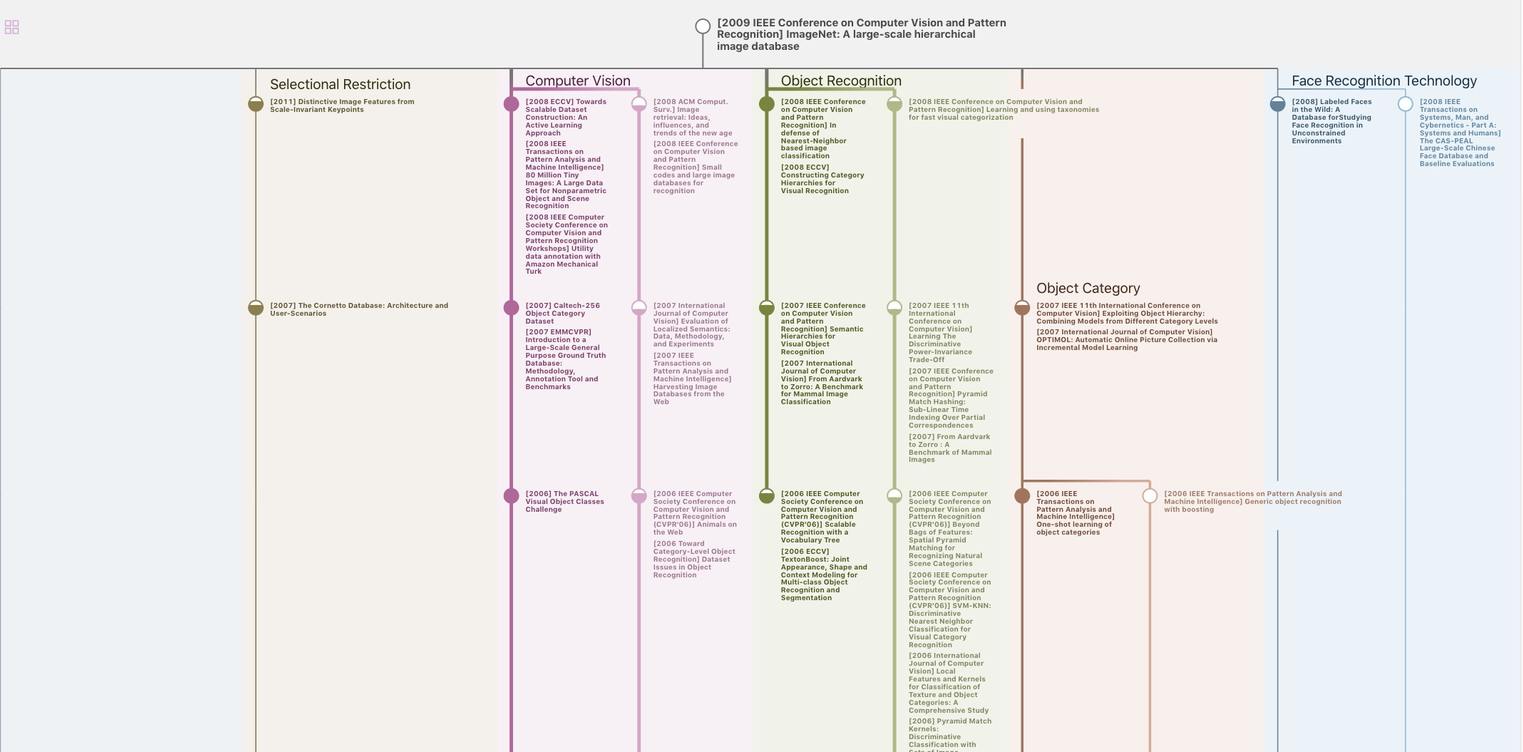
生成溯源树,研究论文发展脉络
Chat Paper
正在生成论文摘要