Seemingly unrelated extreme learning machine.
Neurocomputing(2019)
摘要
A seemingly unrelated regression (SUR) system simultaneously studies the groups of samples that are related with each other through the covariance of decision attributes. Each group of samples is studied by a regression equation, and the error terms of the regression equations are correlated in SUR system. Extreme Learning Machine (ELM) is a training method for single-hidden layer feedforward neural network, it is widely used in many machine learning domains due to the good generalization capability and fast training speed. Since a single ELM ignores the correlated information among different groups of samples, it fails to solve the SUR problem effectively. This ineffectiveness becomes more obvious with the correlation among equations going up. In order to overcome this problem, an extended ELM model is proposed in this paper, described as Seemingly Unrelated ELM (SUELM). SUELM simultaneously learns multiple ELMs by sufficiently using the correlated information among different groups of samples, thus it can solve the SUR problem effectively. In comparison with a single ELM, SUELM significantly improves the performance. Simulation results show that SUELM performs better than the single ELM with respect to mean square error and generalization ability, especially when significant correlations exist among different groups of samples. This paper provides an effective way for solving the SUR problem by adopting ELM as the learning model.
更多查看译文
关键词
Seemingly unrelated regression (SUR) model,Extreme learning machine (ELM),Significant correlation,Prediction error
AI 理解论文
溯源树
样例
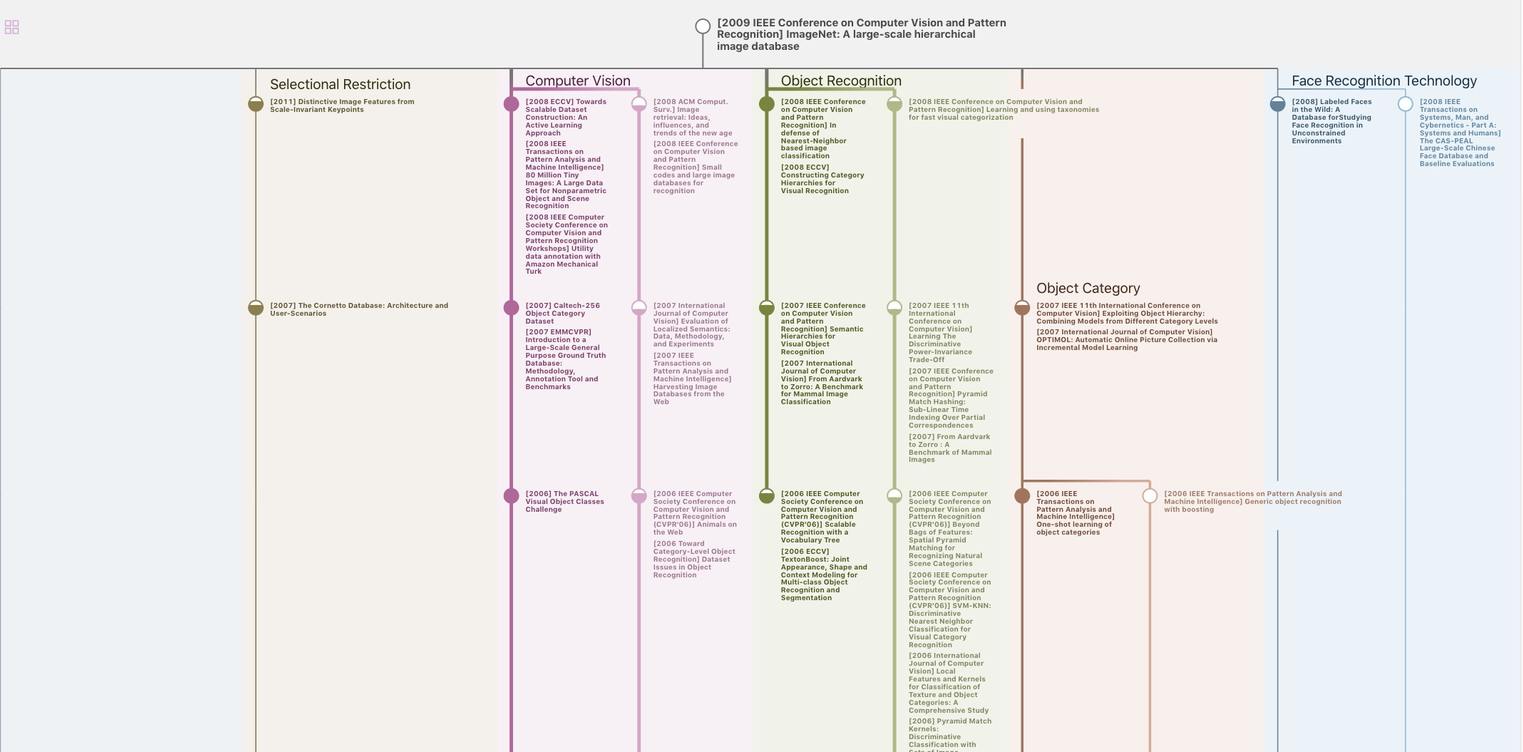
生成溯源树,研究论文发展脉络
Chat Paper
正在生成论文摘要