Kernel Joint Sparse Representation Based on Self-Paced Learning for Hyperspectral Image Classification.
REMOTE SENSING(2019)
摘要
By means of joint sparse representation (JSR) and kernel representation, kernel joint sparse representation (KJSR) models can effectively model the intrinsic nonlinear relations of hyperspectral data and better exploit spatial neighborhood structure to improve the classification performance of hyperspectral images. However, due to the presence of noisy or inhomogeneous pixels around the central testing pixel in the spatial domain, the performance of KJSR is greatly affected. Motivated by the idea of self-paced learning (SPL), this paper proposes a self-paced KJSR (SPKJSR) model to adaptively learn weights and sparse coefficient vectors for different neighboring pixels in the kernel-based feature space. SPL strateges can learn a weight to indicate the difficulty of feature pixels within a spatial neighborhood. By assigning small weights for unimportant or complex pixels, the negative effect of inhomogeneous or noisy neighboring pixels can be suppressed. Hence, SPKJSR is usually much more robust. Experimental results on Indian Pines and Salinas hyperspectral data sets demonstrate that SPKJSR is much more effective than traditional JSR and KJSR models.
更多查看译文
关键词
hyperspectral image classification,self-paced learning,kernel,joint sparse representation
AI 理解论文
溯源树
样例
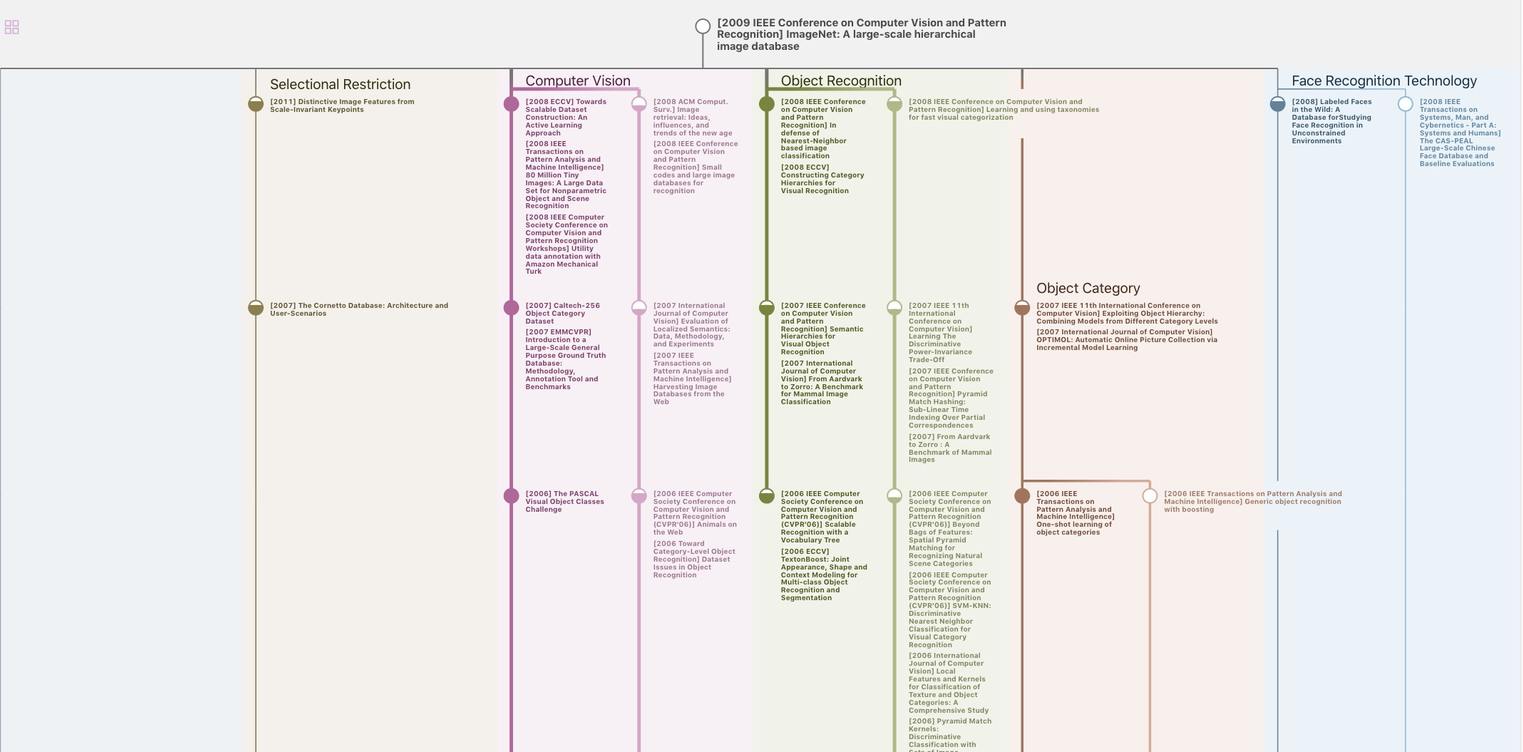
生成溯源树,研究论文发展脉络
Chat Paper
正在生成论文摘要