A semi-supervised approach of graph-based with local and global consistency.
IJITM(2019)
摘要
An approach of graph-based semi-supervised learning is proposed that consider the local and global consistency of data. Like most graph-based semi-supervised learning, the algorithm mainly focused on two key issues: the graph construction and the manifold regularisation framework. In the graph construction, these labelled and unlabelled data are represented as vertices encoding edges weights with the similarity of instances, which means that not only the local geometry information but also the class information are utilised. In manifold regularisation framework, the cost function contains two terms of smoothness constraint and fitting constraint, it is sufficiently smooth with respect to the intrinsic structure revealed by known labelled and unlabelled instances. Specifically, we design the algorithm that uses the normalised Laplacian eigenvectors, which ensure the cost function can converge to closed form expression and then, we provide the convergence proof. Experimental results on various datasets and entity relationship classification show that the proposed algorithm mostly outperforms the popular classification algorithm.
更多查看译文
关键词
semi-supervised learning,graph construction,data consistency,manifold regularisation
AI 理解论文
溯源树
样例
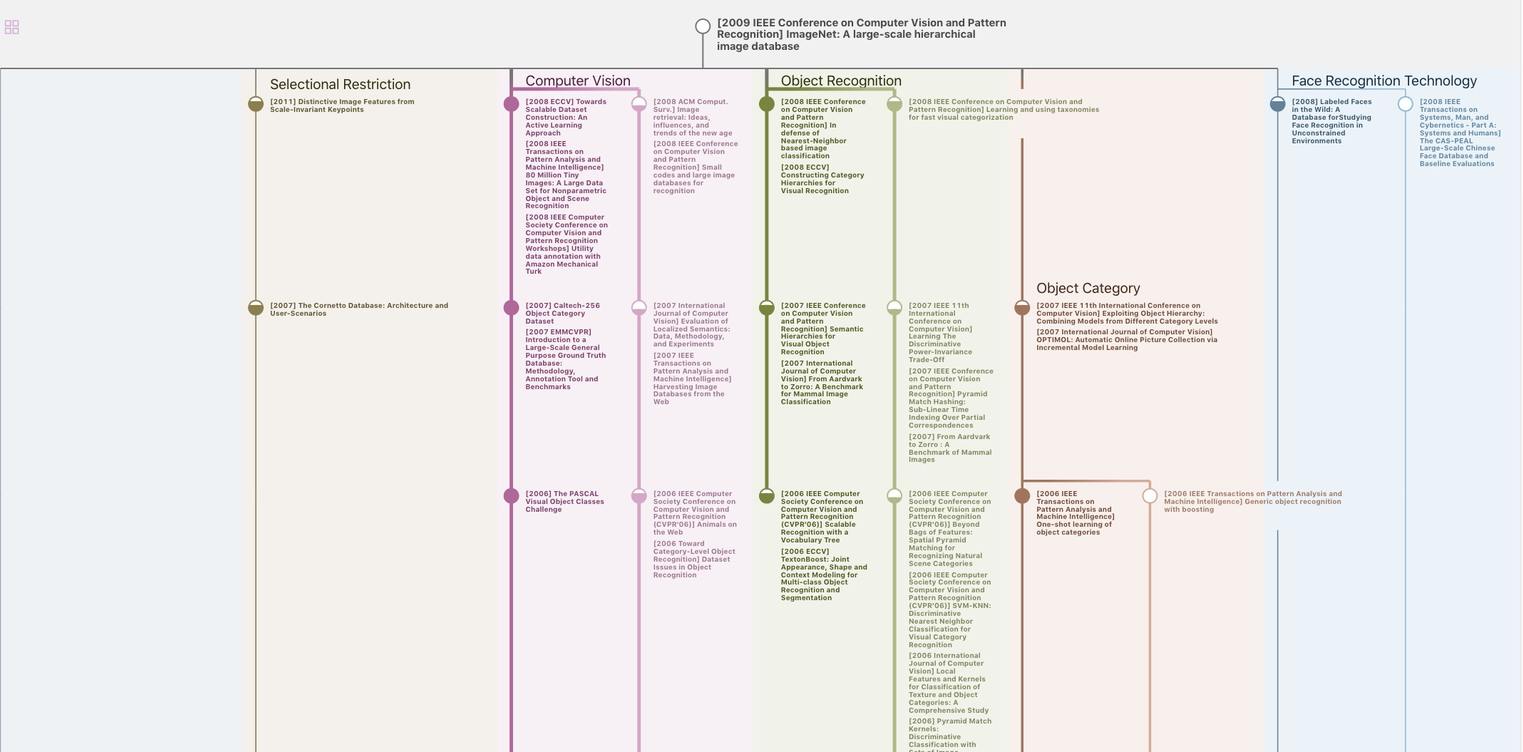
生成溯源树,研究论文发展脉络
Chat Paper
正在生成论文摘要