Predicting Road Traffic Accident Severity Using Accident Report Data in South Africa
Digital Government Research(2019)
摘要
Road traffic accidents (RTAs) have a significant impact on individuals, their families and the nation. Without knowledgeable action, road traffic injuries are said to be the seventh leading cause of death by the year 2030. Generally, classification models have been created to predict injury severity in RTAs while taking into account vehicle, light & weather conditions, and road characteristics. This study looked at how to best create a classification model that predicts injury severity of drivers by adding distance features of nearest places of interest namely, malls, bars, schools, buildings, and points of interest. The reason for this was to determine if distances to closest places of interest has an impact on injury severity of drivers in RTAs. The classification models were created with the Multivariate logistic regression (MLR) classification method and the Extreme gradient boosting tree (XGBoost) classification method, where XGBoost outperformed MLR. The findings also showed that data that is collected on South African Accident Report (AR) forms and spatial data have the ability to significantly improve the overall performance of a injury severity model. Also, truck licenses code C1, light motor duty license code EB, vehicle type (motor car or station wagon), single vehicle: overturned accident type, vehicle manoeuvre, and the distance to the closest building were among the top predictors that contributed to injury severity of drivers in RTAs.
更多查看译文
关键词
Road traffic accidents,Multivariate logistic regression,Extreme gradient boosting trees (XGBoost)
AI 理解论文
溯源树
样例
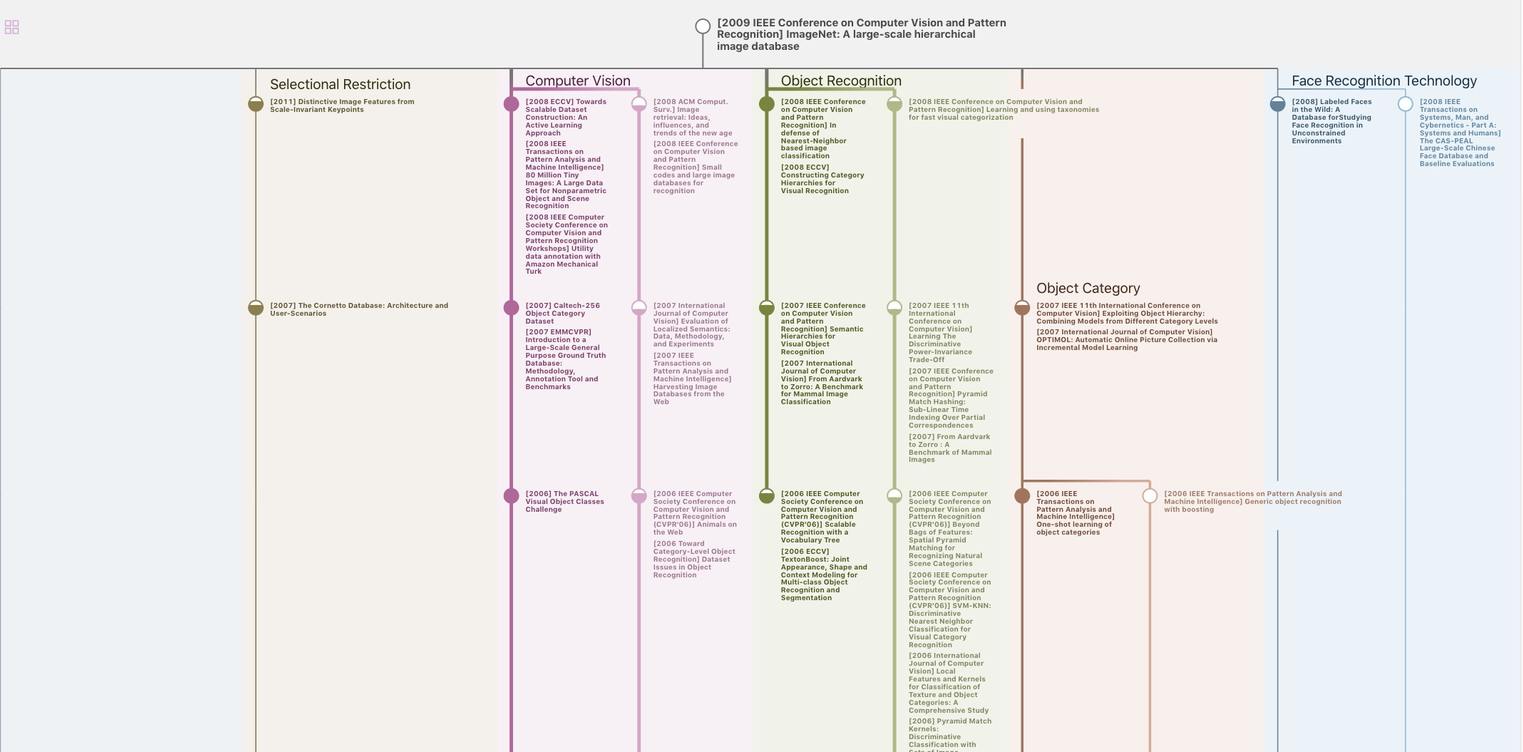
生成溯源树,研究论文发展脉络
Chat Paper
正在生成论文摘要