Learning Classifier Synthesis for Generalized Few-Shot Learning
arxiv(2019)
摘要
Visual recognition in real-world requires handling long-tailed and even open-ended data. It is a practical utility of a visual system to reliably recognizing the populated "head" visual concepts and meanwhile to learn about "tail" categories of few instances. Class-balanced many-shot learning and few-shot learning tackle one side of this challenging problem, via either learning strong classifiers for populated categories or few-shot classifiers for the tail classes. In this paper, we investigate the problem of generalized few-shot learning, where recognition on the head and the tail are performed jointly. We propose a neural dictionary-based ClAssifier SynThesis LEarning (CASTLE) approach to synthesizes the calibrated "tail" classifiers in addition to the multi-class "head" classifiers, and simultaneously recognizes the head and tail visual categories in a global discerning framework. CASTLE has demonstrated superior performances across different learning scenarios, i.e., many-shot learning, few-shot learning, and generalized few-shot learning, on two standard benchmark datasets --- MiniImageNet and TieredImageNet.
更多查看译文
AI 理解论文
溯源树
样例
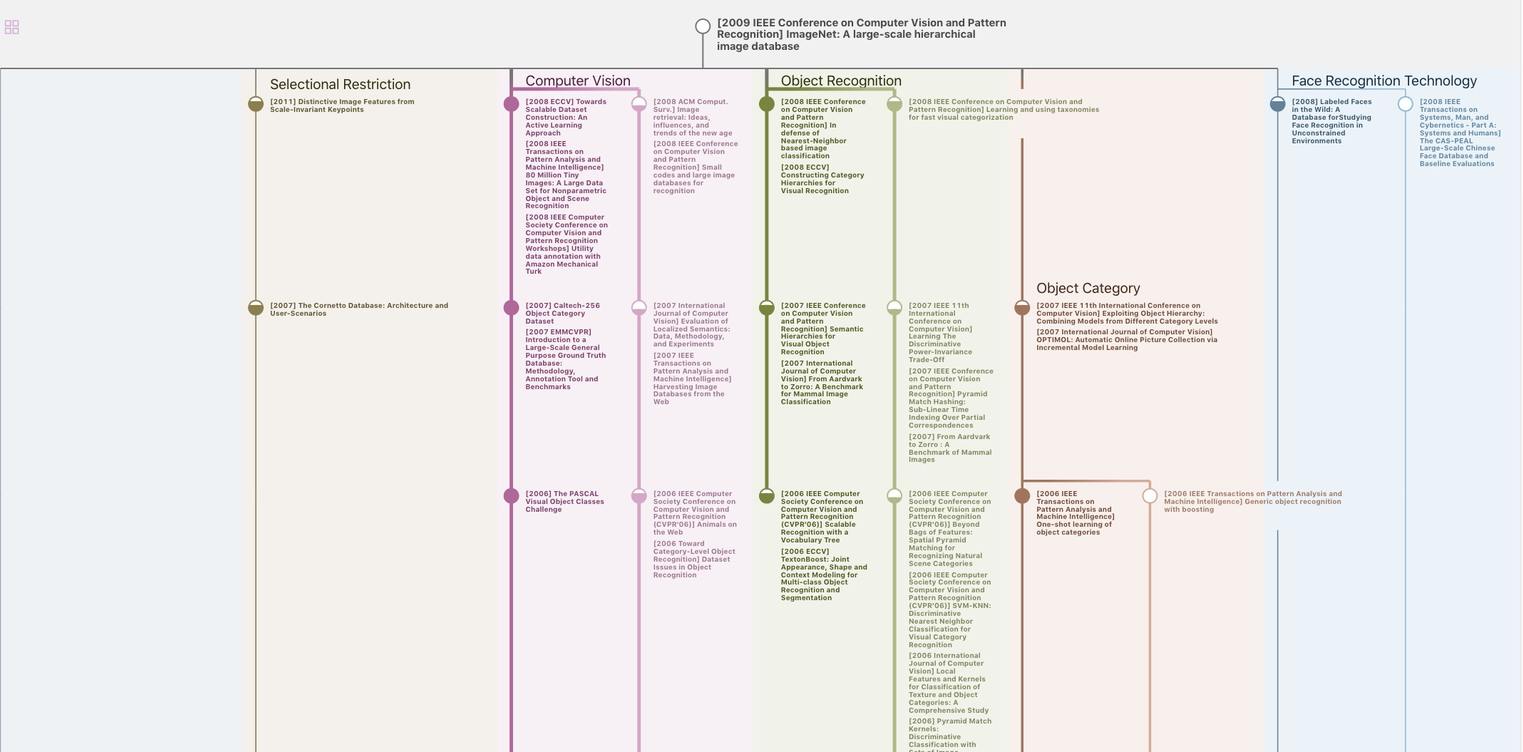
生成溯源树,研究论文发展脉络
Chat Paper
正在生成论文摘要