Evolving Knowledge Graphs
IEEE INFOCOM 2019 - IEEE Conference on Computer Communications(2019)
摘要
Many practical applications have observed knowledge evolution, i.e., continuous born of new knowledge, with its formation influenced by the structure of historical knowledge. This observation gives rise to evolving knowledge graphs whose structure temporally grows over time. However, both the modal characterization and the algorithmic implementation of evolving knowledge graphs remain unexplored. To this end, we propose EvolveKG, a framework that reveals cross-time knowledge interaction with desirable performance of storage and computation. The novelty of EvolveKG lies in Derivative Graph – a static weighted snapshot of evolution at a certain time. Particularly, each weight quantifies knowledge effectiveness with a temporarily decaying function of consistency and attenuation, two proposed factors depicting whether or not the effectiveness of a fact fades away with time. Thanks to the cross-time interaction, EvolveKG allows future knowledge prediction by virtue of the influence from the historical ones. Empirically tested under two real datasets, the superiority of EvolveKG is confirmed via its prediction accuracy.
更多查看译文
关键词
Attenuation,Knowledge discovery,Information retrieval,Semantics,Lifting equipment,Prediction algorithms,Computational complexity
AI 理解论文
溯源树
样例
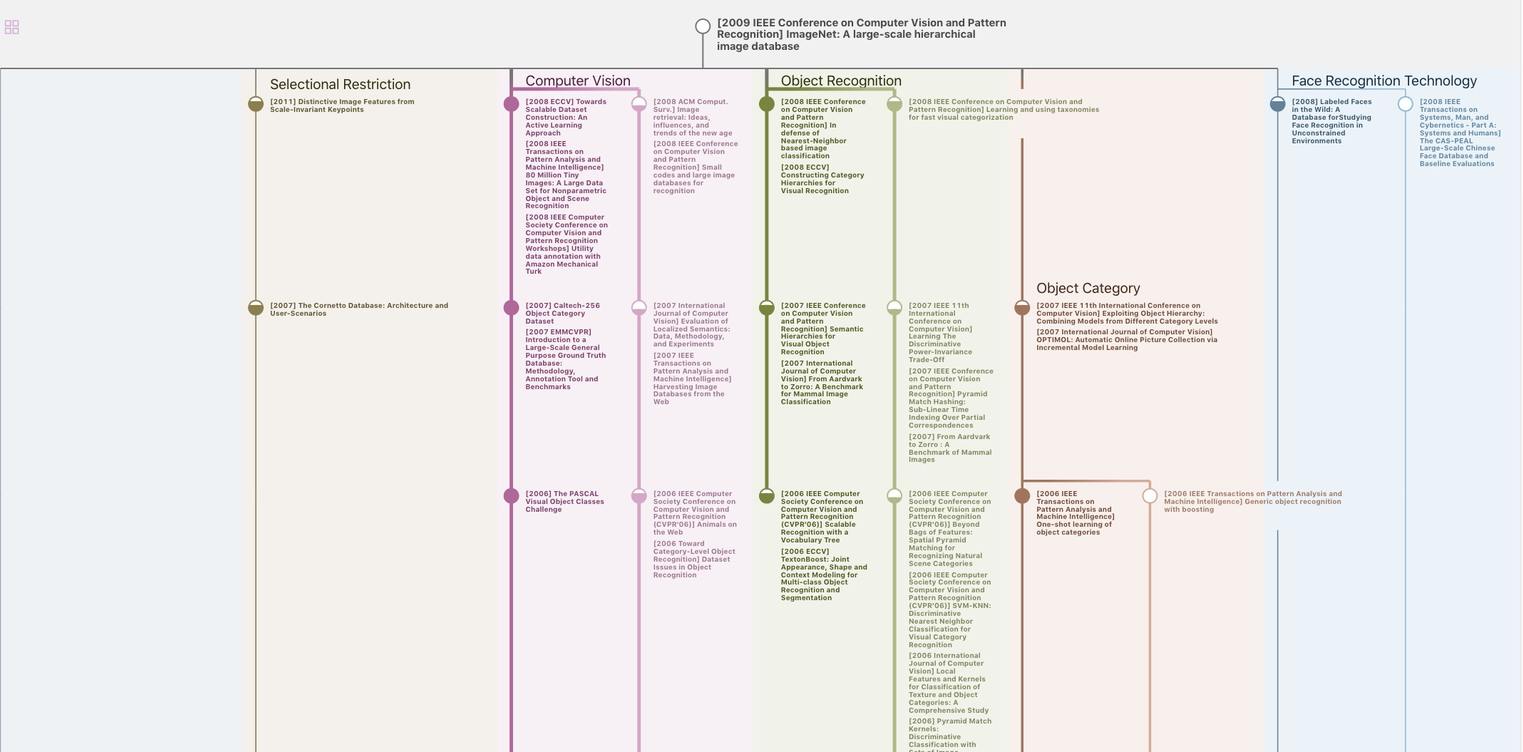
生成溯源树,研究论文发展脉络
Chat Paper
正在生成论文摘要