Online Detection of Abnormal Passenger Out-Flow in Urban Metro System
Neurocomputing(2019)
摘要
Accurate forecasting of the number of passengers coming into the urban metro system, namely, passenger in-flow has been one of the most important issues in the research on the intelligent transportation system. Existing studies aim at making the prediction mainly by learning the recurring patterns from historical passenger in-flows. However, passenger out-flows from the urban metro system, which may usually contribute to understanding passenger behavior, and help forecast passenger in-flows, especially burst in-flows on unusual conditions, have rarely been studied yet. In this paper, we propose a model called EAD-OF (Elastic Abnormality Detection for Out-flow) to real-time detect the abnormal passenger out-flows and alarm the administrators in time. Furthermore, we analyze the characteristics of passenger in-flow, out-flow and their correlation. We evaluate our model with real transportation data of the biggest city in China and use large-scale social event data to further explain our model. The result shows that EAD-OF can effectively detect the abnormal out-flows which would cause burst in-flows with performance tens of times better than the baseline models. In term of efficiency, EAD-OF can also beat the direct computation approach several times.
更多查看译文
关键词
Anomaly detection,Online algorithm,Intelligent transportation system,Big data mining,Urban computing
AI 理解论文
溯源树
样例
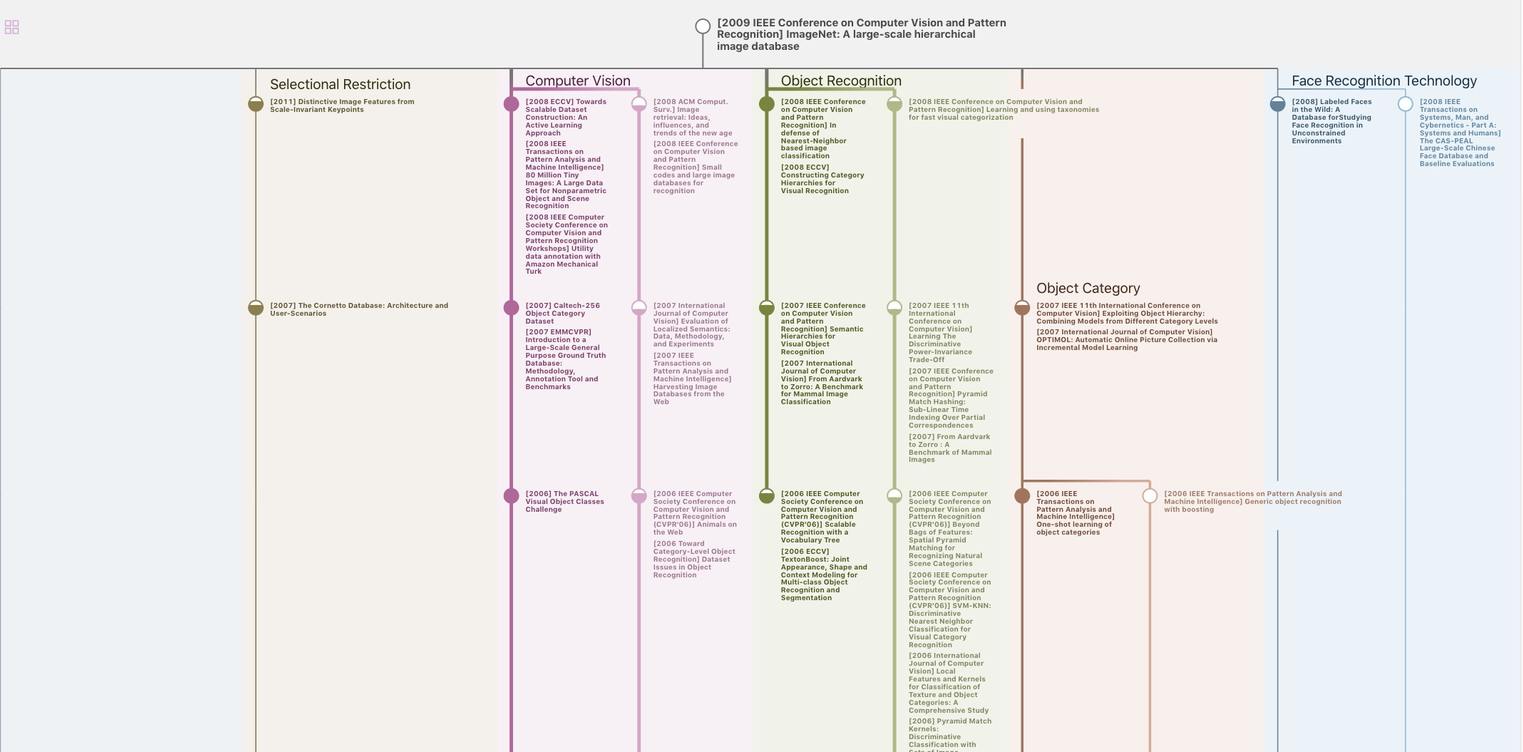
生成溯源树,研究论文发展脉络
Chat Paper
正在生成论文摘要