Generalizing from a Few Examples: A Survey on Few-shot Learning
ACM Computing Surveys(2020)
摘要
AbstractMachine learning has been highly successful in data-intensive applications but is often hampered when the data set is small. Recently, Few-shot Learning (FSL) is proposed to tackle this problem. Using prior knowledge, FSL can rapidly generalize to new tasks containing only a few samples with supervised information. In this article, we conduct a thorough survey to fully understand FSL. Starting from a formal definition of FSL, we distinguish FSL from several relevant machine learning problems. We then point out that the core issue in FSL is that the empirical risk minimizer is unreliable. Based on how prior knowledge can be used to handle this core issue, we categorize FSL methods from three perspectives: (i) data, which uses prior knowledge to augment the supervised experience; (ii) model, which uses prior knowledge to reduce the size of the hypothesis space; and (iii) algorithm, which uses prior knowledge to alter the search for the best hypothesis in the given hypothesis space. With this taxonomy, we review and discuss the pros and cons of each category. Promising directions, in the aspects of the FSL problem setups, techniques, applications, and theories, are also proposed to provide insights for future research.1
更多查看译文
关键词
Few-shot learning, one-shot learning, low-shot learning, small sample learning, meta-learning, prior knowledge
AI 理解论文
溯源树
样例
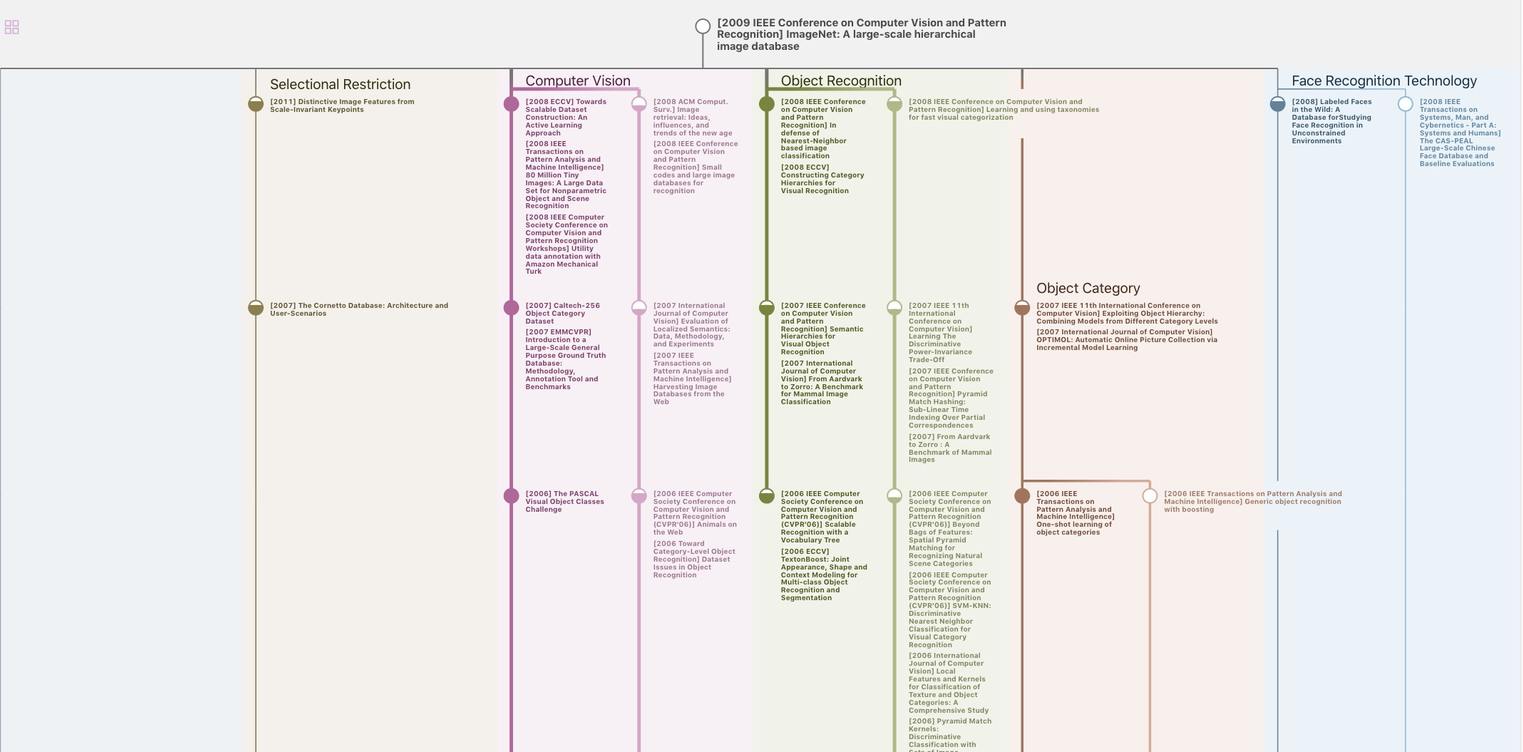
生成溯源树,研究论文发展脉络
Chat Paper
正在生成论文摘要