An adaptable approach to automated visual detection of plant organs with applications in grapevine breeding
Biosystems Engineering(2019)
摘要
Periodic and multi-scale field phenotyping of seedlings, mapping populations or genetic repositories are one of the most challenging demands of today's (grapevine) breeding programs and research. The challenges are given by large field sizes with several hundreds of plants that need to be phenotyped nowadays by laborious, manual and subjective classification methods. This work presents an adaptable and transferable approach to the automated detection, localisation and counting of plant organs in RGB images given different growth stages of the plants and different procedures of image acquisition. Adaptability and transferability are achieved by employing a deep learning semantic segmentation framework allowing an end-to-end learning of all system parameters of the complete workflow and thereby replacing the programming of different system variants for different scenarios. Adaptability and transferability are demonstrated within an extensive evaluation of six experimental series using images taken at the Institute for Grapevine Breeding Geilweilerhof yielding localisation accuracies of up to 87.3% IoU and classification accuracies up to 88.6% F1 score. (C) 2019 IAgrE. Published by Elsevier Ltd. All rights reserved.
更多查看译文
关键词
Vitis vinifera ssp. vinifera,computer-based phenotyping,semantic segmentation,digitisation,BBCH stages,Phenoliner
AI 理解论文
溯源树
样例
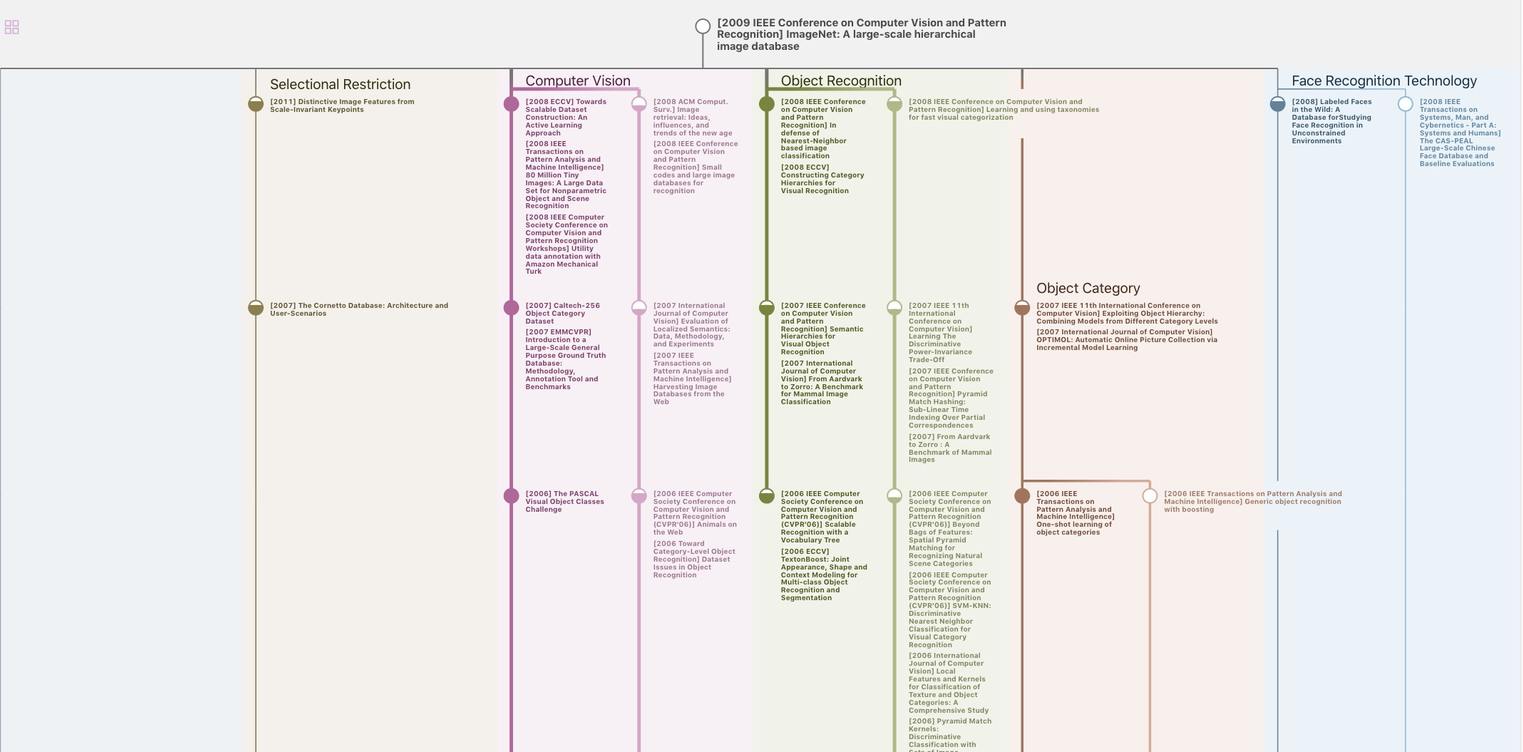
生成溯源树,研究论文发展脉络
Chat Paper
正在生成论文摘要