Improved Cooperative Multi-agent Reinforcement Learning Algorithm Augmented by Mixing Demonstrations from Centralized Policy
adaptive agents and multi-agents systems(2019)
摘要
Many decision problems for complex systems that involve multiple decision makers can be formulated as a decentralized partially observable markov decision process (dec-POMDP) problem. Due to the computational difficulty with obtaining optimal policies, recent approaches to dec-POMDP often use a multi-agent reinforcement learning (MARL) algorithm. We propose a method to improve the existing cooperative MARL algorithms by adopting an imitation learning technique. For a reference policy in the imitation learning part, we use a centralized policy from a multi-agent MDP or a multi-agent POMDP model reduced from the original dec-POMDP model. In the proposed method, during the training process, we mix demonstrations from the reference policy by using a demonstration buffer. Demonstration samples from the buffer are used in the augmented policy gradient function for policy updates. We assess the performance of the proposed method for three well-known dec-POMDP benchmark problems Mars rover, co-operative box pushing, and dec-tiger. Experimental results indicate that augmenting the baseline MARL algorithm by mixing the demonstrations significantly improves the quality of policy solutions. With these results, we conclude that the imitation learning can enhance MARL algorithms and that policy solutions from MMDP and MPOMDP models are a reasonable reference policy to use in the proposed algorithm.
更多查看译文
关键词
Multi-agent reinforcement learning,Cooperative decision making problem,dec-POMDP,Imitation learning
AI 理解论文
溯源树
样例
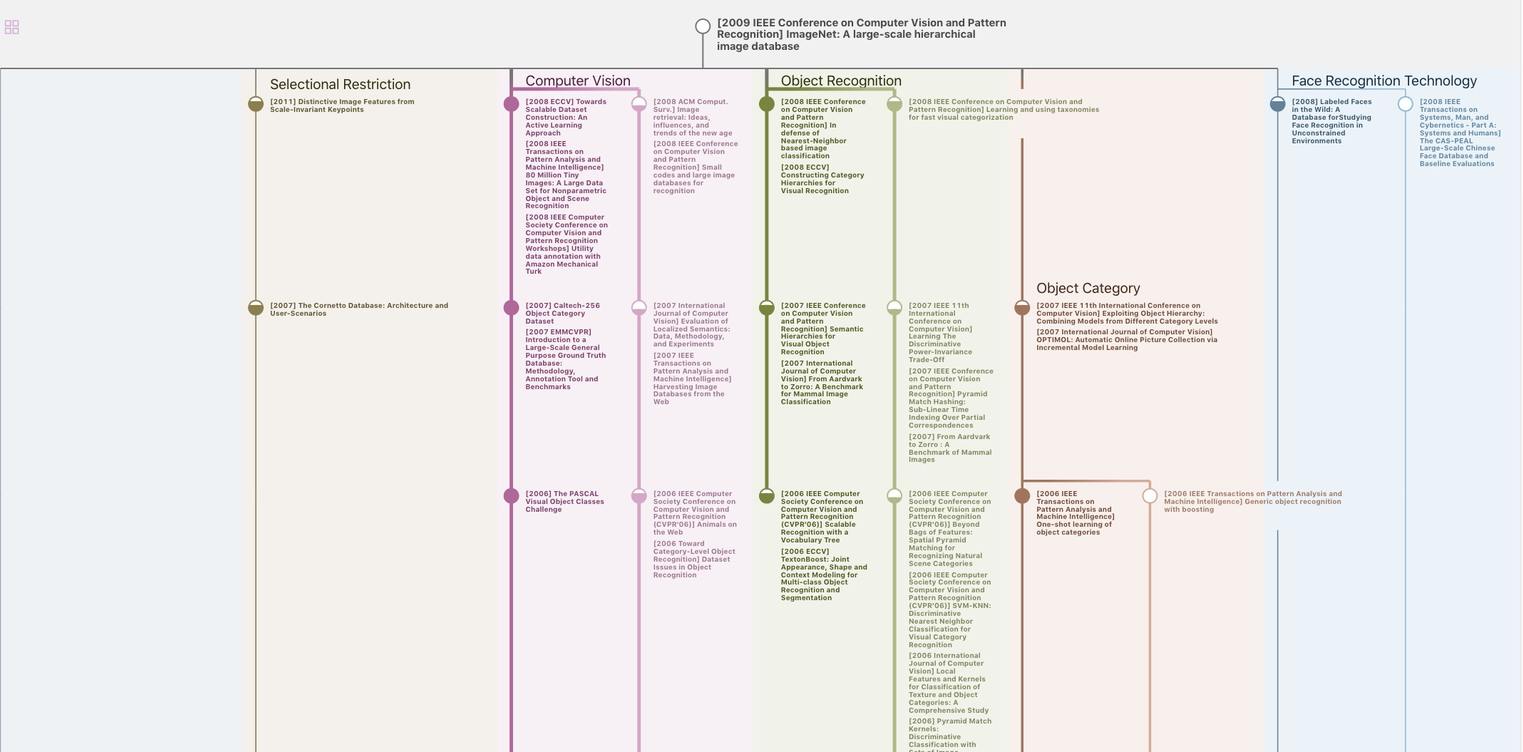
生成溯源树,研究论文发展脉络
Chat Paper
正在生成论文摘要