A New Framework to Reduce Doctor’s Workload for Medical Image Annotation
IEEE Access(2019)
摘要
Accurate annotation of the medical image is the crucial step for image artificial intelligence (AI) clinical application. However, annotating medical image will incur a lot of annotation efforts and expense due to its high complexity and needing experienced doctors. In order to reduce the cost of annotation, several active learning methods have been proposed previously. However, the focus of these methods is to reduce the number of annotation candidates with little regard for doctor's workload, which is not enough for medical image annotation, since even annotating a small amount of data will take a lot of time for doctors. In order to effectively reduce the workload of doctors, we developed a new framework for medical image annotation. First, by combining active learning and U-shape network, we employed a suggestive annotation strategy to select the most effective annotation candidates. We then exploited a fine annotation platform to alleviate annotating efforts on each candidate and utilized a new criterion to quantitatively calculate the efforts of doctors. In this paper, we used MR brain tissue segmentation as an example to evaluate the proposed method. The extensive experiments with the IBSR18 and MRBrainS18 Challenge datasets showed that with the proposed strategy, only 60% of the annotation candidates were needed to achieve the most advanced segmentation performance, and the annotation workload of doctors can be alleviated by at least 44%, 44%, and 47% on cerebrospinal fluid (CSF), gray matter (GM), and white matter (WM), respectively. Meanwhile, our proposed method was proven to be effective for annotation of clinical data collected from our hospital, where the annotation workload of doctors was alleviated by at least 32% on WM and the time of annotating each clinical candidate is expected to be saved by at least 64%.
更多查看译文
关键词
Medical image annotation,active learning,fine annotation,annotation workload
AI 理解论文
溯源树
样例
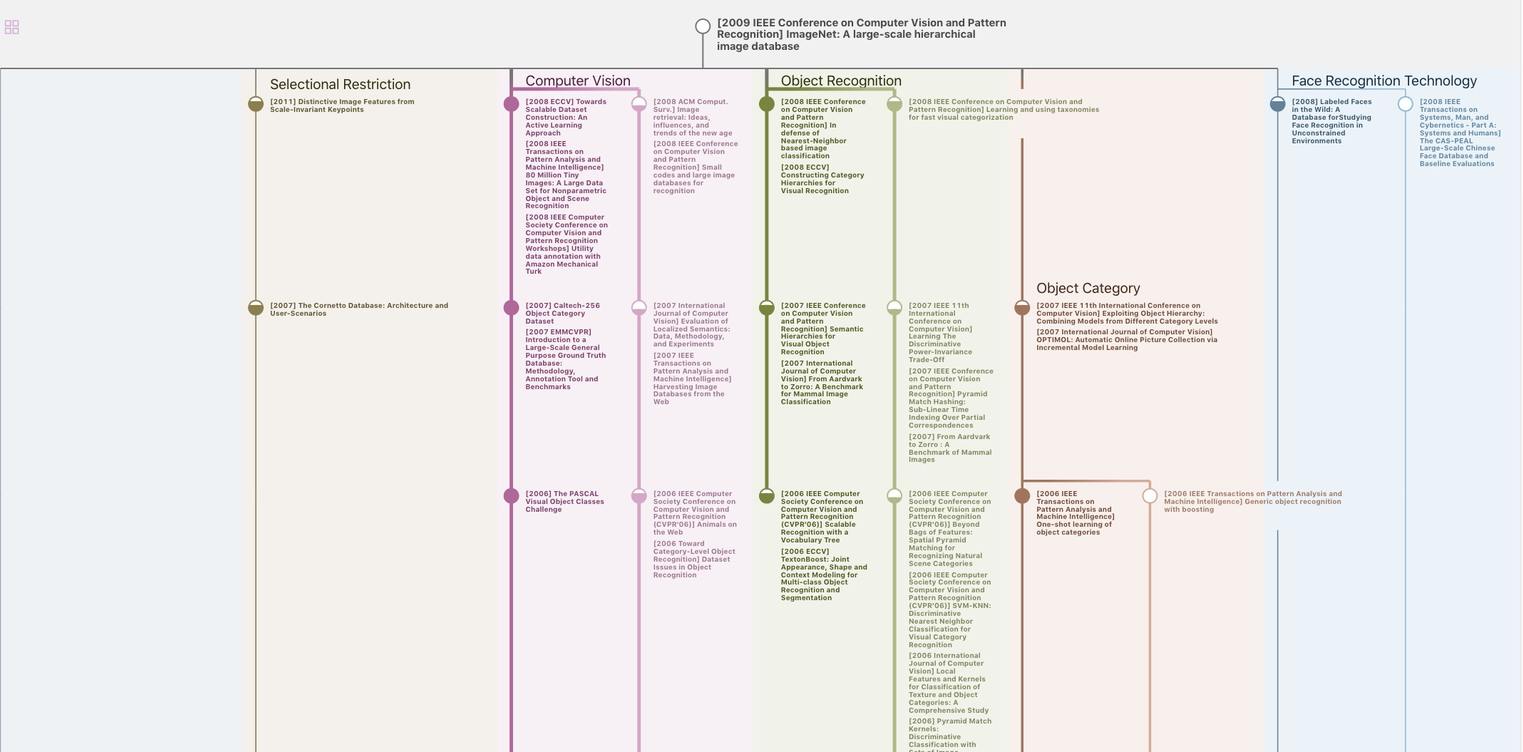
生成溯源树,研究论文发展脉络
Chat Paper
正在生成论文摘要