A Q-values Sharing Framework for Multiple Independent Q-learners
adaptive agents and multi-agents systems(2019)
摘要
By using a multiagent reinforcement learning (MARL) framework, cooperative agents can communicate with one another to accelerate the joint learning. In the teacher-student paradigm applied in MARL, a more experienced agent (advisor) can advise another agent (advisee) which action to take in a state. However, when agents need to cooperate with one another, the advisee may fail to cooperate well with others since their policies may have changed. It requires a long period for an advisee to learn the same best actions as an advisor has learned, especially when the amount of advice is limited. We propose a partaker-sharer advising framework (PSAF) for independent Q-learners with limited communication in cooperative MARL. In PSAF, the overall learning process is shown to accelerate by multiple independent Q-learners' sharing their maximum Q-values with one another at every time step. We perform experiments in the Predator-Prey domain and HFO game. The results show that our approach significantly outperforms existing advising methods.
更多查看译文
关键词
multiagent learning,Q-learning,reinforcement learning
AI 理解论文
溯源树
样例
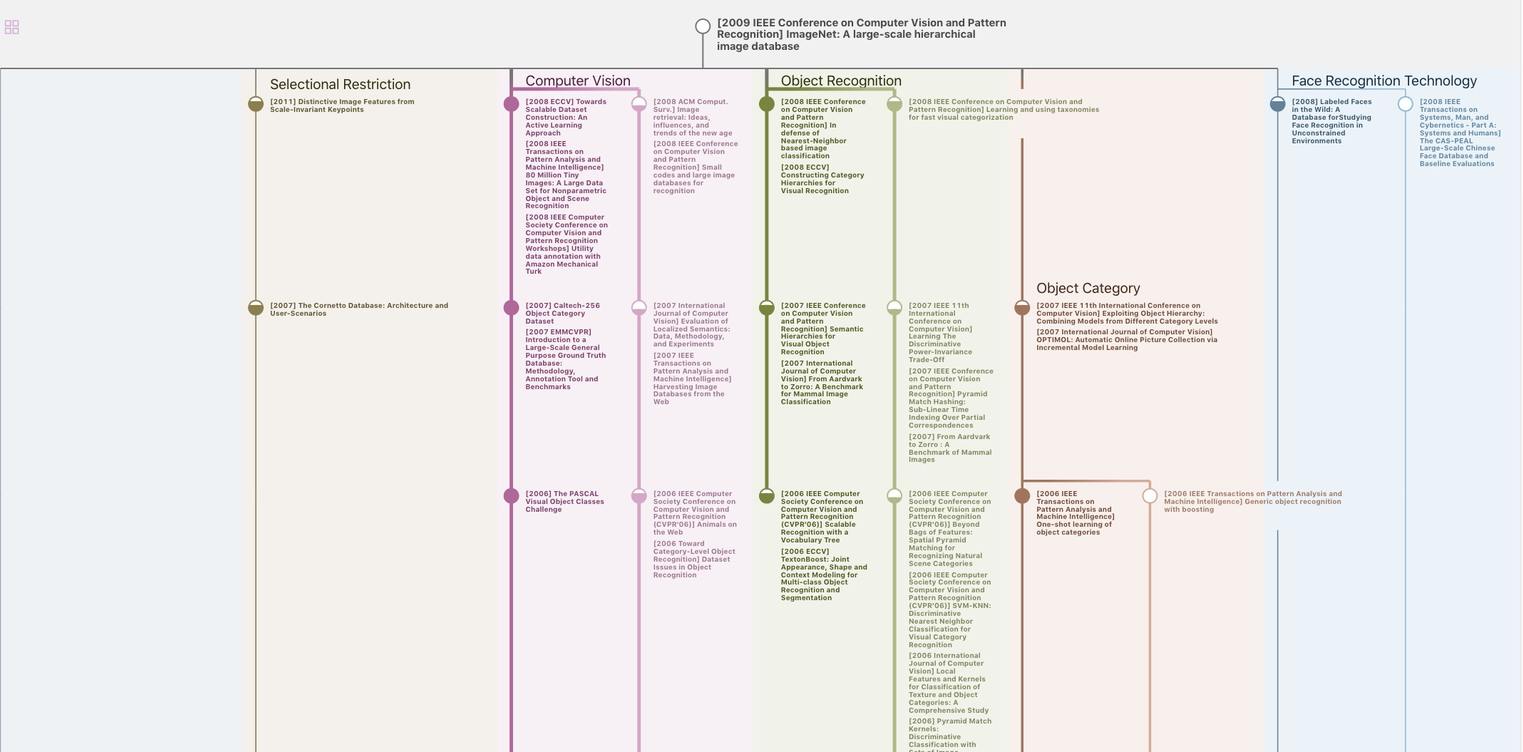
生成溯源树,研究论文发展脉络
Chat Paper
正在生成论文摘要