Estimation of the Daily Variability of Aggregate Wind Power Generation in Alberta, Canada
ENERGIES(2019)
摘要
This paper describes a hierarchy of increasingly complex statistical models for wind power generation in Alberta applied to wind power production data that are publicly available. The models are based on combining spatial and temporal correlations. We apply the method of Gaussian random fields to analyze the wind power time series of the 19 existing wind farms in Alberta. Following the work of Gneiting et al., three space-time models are used: Stationary, Separability, and Full Symmetry. We build several spatio-temporal covariance function estimates with increasing complexity: separable, non-separable and symmetric, and non-separable and non-symmetric. We compare the performance of the models using kriging predictions and prediction intervals for both the existing wind farms and a new farm in Alberta. It is shown that the spatial correlation in the models captures the predominantly westerly prevailing wind direction. We use the selected model to forecast the mean and the standard deviation of the future aggregate wind power generation of Alberta and investigate new wind farm siting on the basis of reducing aggregate variability.
更多查看译文
关键词
wind power modeling,statistical models,spatio-temporal modeling,Gaussian random fields,kriging,power forecast,aggregate power,variability
AI 理解论文
溯源树
样例
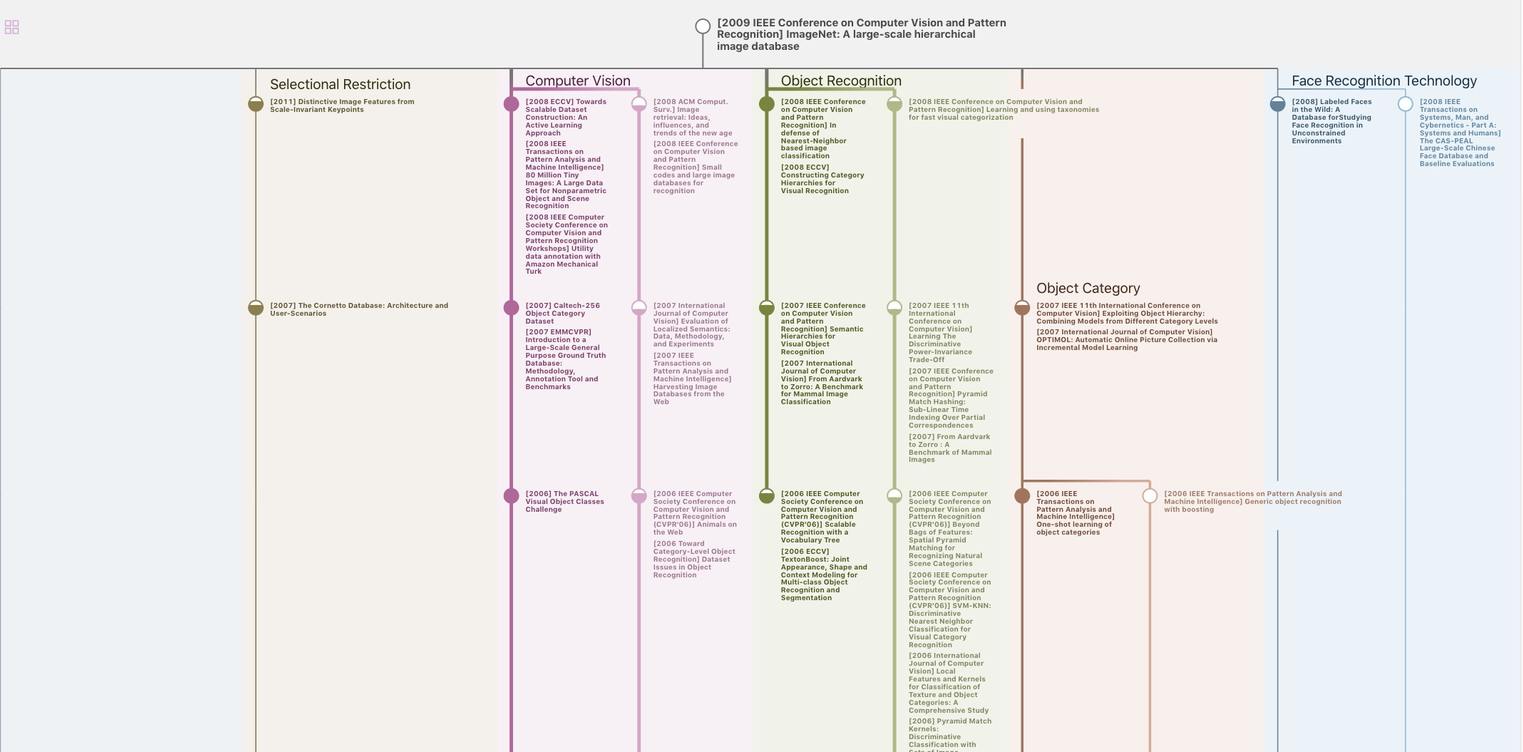
生成溯源树,研究论文发展脉络
Chat Paper
正在生成论文摘要