Improving Event Coreference Resolution by Learning Argument Compatibility from Unlabeled Data
north american chapter of the association for computational linguistics(2019)
摘要
Argument compatibility is a linguistic condition that is frequently incorporated into modern event coreference resolution systems. If two event mentions have incompatible arguments in any of the argument roles, they cannot be coreferent. On the other hand, if these mentions have compatible arguments, then this may be used as information toward deciding their coreferent status. One of the key challenges in leveraging argument compatibility lies in the paucity of labeled data. In this work, we propose a transfer learning framework for event coreference resolution that utilizes a large amount of unlabeled data to learn the argument compatibility between two event mentions. In addition, we adopt an interactive inference network based model to better capture the (in)compatible relations between the context words of two event mentions. Our experiments on the KBP 2017 English dataset confirm the effectiveness of our model in learning argument compatibility, which in turn improves the performance of the overall event coreference model.
更多查看译文
关键词
Named Entity Recognition,Natural Language Processing,Word Representation
AI 理解论文
溯源树
样例
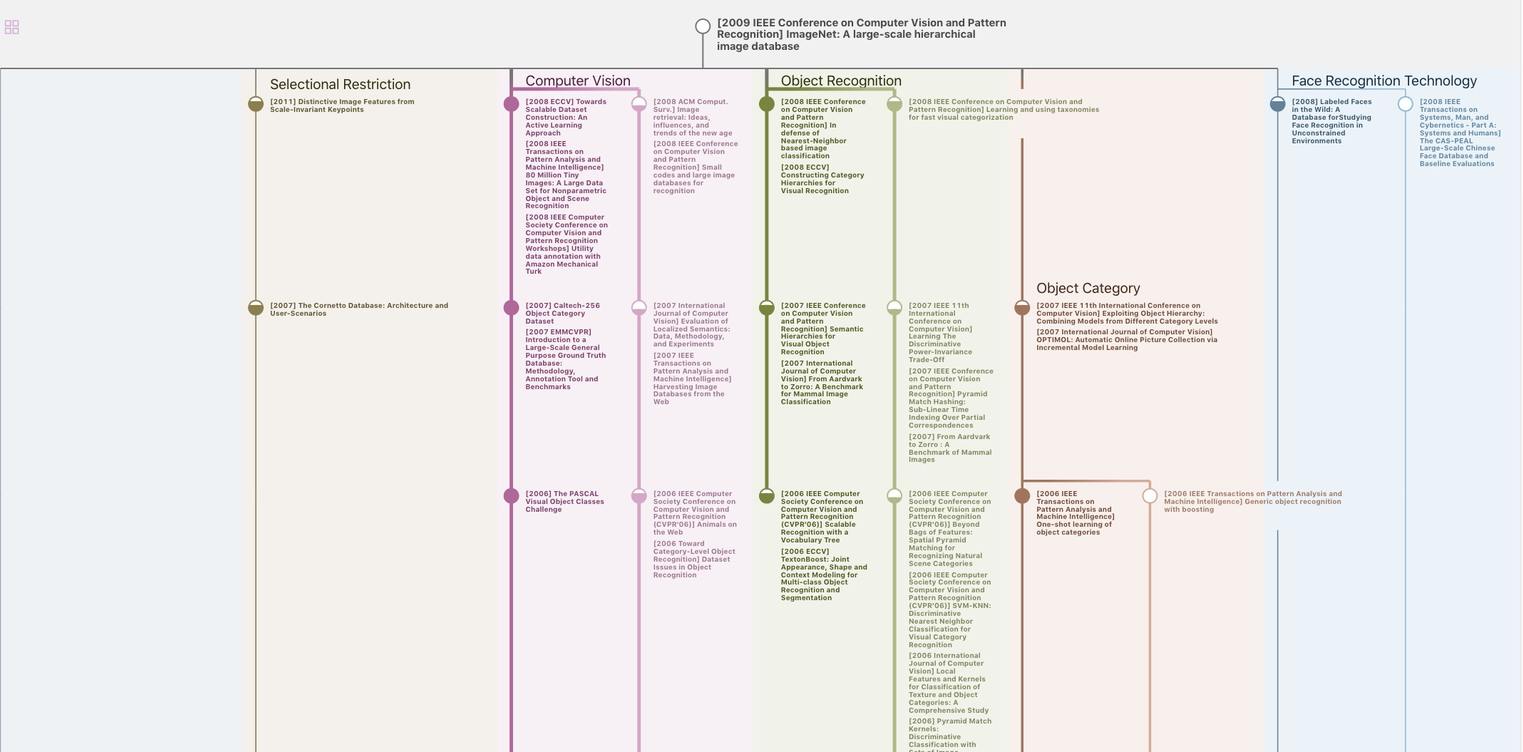
生成溯源树,研究论文发展脉络
Chat Paper
正在生成论文摘要