Efficient Estimation and Prediction for Sparse Time-Varying Underwater Acoustic Channels
IEEE Journal of Oceanic Engineering(2020)
摘要
This paper investigates the estimation and prediction of the sparse time-varying channel in underwater acoustic communication systems. The estimation approach exploits the sparse structure of the delay-Doppler representation of the channel. Various state-of-the-art matching pursuit (MP)-type algorithms for sparse signal reconstruction are compared. Among the considered algorithms, sparsity adaptive MP (SaMP) and its variant adaptive step size SaMP have the advantage of not requiring
a priori
knowledge of the sparsity level and outperform the other algorithms in terms of mean squared error (MSE). Moreover, due to the fast time-varying nature and the extremely limited bandwidth of the UWA channels, a channel prediction that can provide up-to-date channel state information is necessary for reliable symbol detection. This paper proposes an adaptive channel prediction scheme that extrapolates the channel knowledge estimated from a block of training symbols, and the predicted channel is used to decode consecutive data blocks. The proposed scheme does not require any
a priori
knowledge of channel dynamic model and noise statistics, and is able to provide future channel estimates based solely on current channel estimates. Furthermore, the proposed scheme operates in the delay-Doppler domain, and thus has a remarkably lower computational complexity when compared with the channel prediction in time domain. To further improve the prediction accuracy, past detected symbols are fed back to assist the proposed predictor with an up-to-date channel estimate. Simulation results of the proposed channel estimation and prediction demonstrate a good tradeoff between the MSE/bit error rate and the computational complexity.
更多查看译文
关键词
Channel estimation,Estimation,Doppler effect,Prediction algorithms,Matching pursuit algorithms,Complexity theory,Time-varying channels
AI 理解论文
溯源树
样例
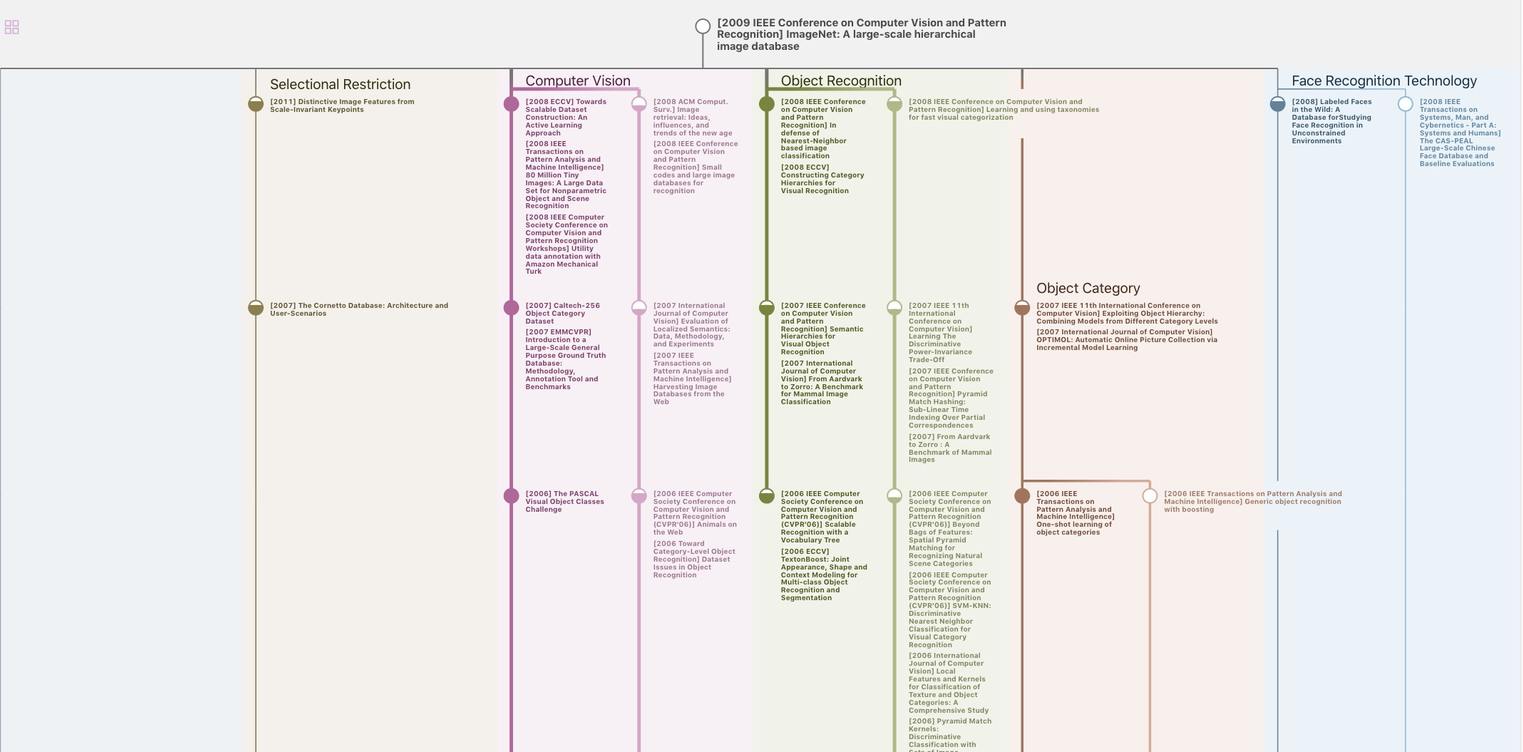
生成溯源树,研究论文发展脉络
Chat Paper
正在生成论文摘要