Learning Simulation-Based Games from Data
adaptive agents and multi-agents systems(2019)
摘要
We tackle a fundamental problem in empirical game-theoretic analysis (EGTA), that of learning equilibria of simulation-based games. Such games cannot be described in analytical form; instead, a blackbox simulator can be queried to obtain noisy samples of utilities. Our approach to EGTA is in the spirit of probably approximately correct learning. We design algorithms that learn empirical games, which uniformly approximate the utilities of simulation-based games from finitely many samples. Our methodology learns all the equilibria of simulation-based games, as opposed to a single one.
更多查看译文
关键词
Empirical Game-Theoretical Analysis,PAC Learning
AI 理解论文
溯源树
样例
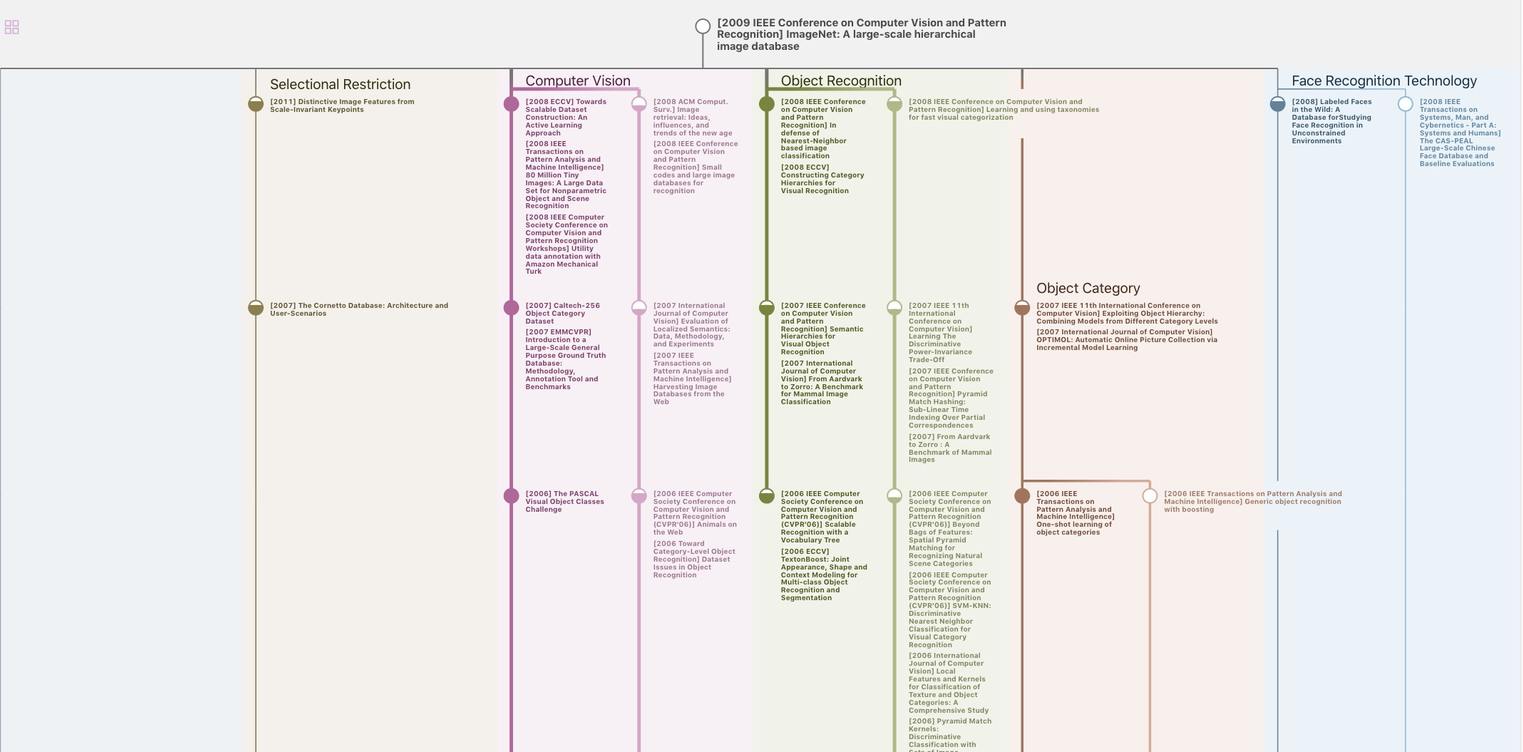
生成溯源树,研究论文发展脉络
Chat Paper
正在生成论文摘要